Deep Transfer Learning on Satellite Imagery Improves Air Quality Estimates in Developing Nations
CoRR(2022)
摘要
Urban air pollution is a public health challenge in low- and middle-income countries (LMICs). However, LMICs lack adequate air quality (AQ) monitoring infrastructure. A persistent challenge has been our inability to estimate AQ accurately in LMIC cities, which hinders emergency preparedness and risk mitigation. Deep learning-based models that map satellite imagery to AQ can be built for high-income countries (HICs) with adequate ground data. Here we demonstrate that a scalable approach that adapts deep transfer learning on satellite imagery for AQ can extract meaningful estimates and insights in LMIC cities based on spatiotemporal patterns learned in HIC cities. The approach is demonstrated for Accra in Ghana, Africa, with AQ patterns learned from two US cities, specifically Los Angeles and New York.
更多查看译文
关键词
satellite imagery,air quality
AI 理解论文
溯源树
样例
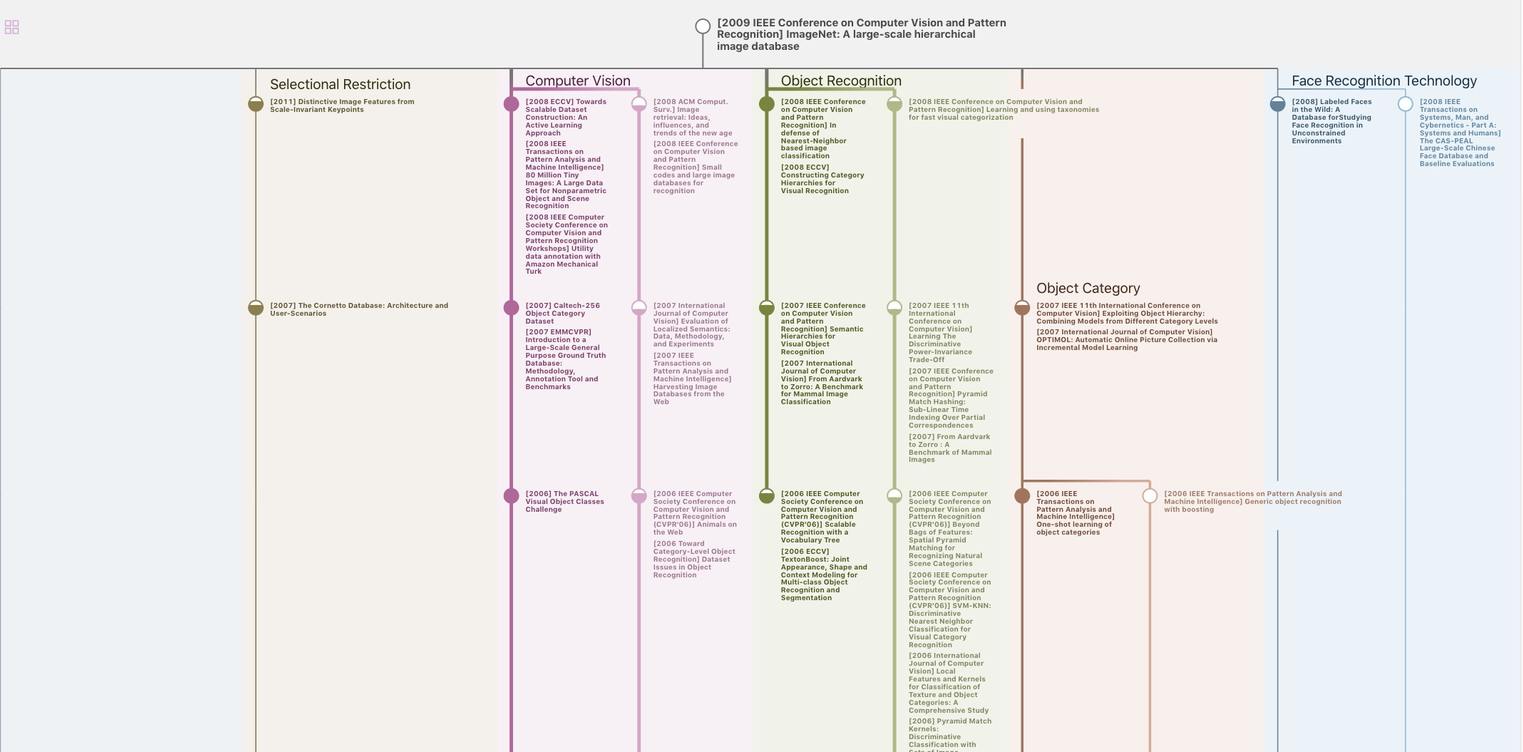
生成溯源树,研究论文发展脉络
Chat Paper
正在生成论文摘要