Unsupervised Face Detection in the Dark
IEEE Transactions on Pattern Analysis and Machine Intelligence(2023)
摘要
Low-light face detection is challenging but critical for real-world applications, such as nighttime autonomous driving and city surveillance. Current face detection models rely on extensive annotations and lack generality and flexibility. In this paper, we explore how to learn face detectors without low-light annotations. Fully exploiting existing normal light data, we propose adapting face detectors from normal light to low light. This task is difficult because the gap between brightness and darkness is too large and complicated at the object level and pixel level. Accordingly, the performance of current low-light enhancement or adaptation methods is unsatisfactory. To solve this problem, we propose a joint High-Low Adaptation (HLA) framework. We design bidirectional low-level adaptation and multitask high-level adaptation. For low-level, we enhance the dark images and degrade the normal-light images, making both domains move toward each other. For high-level, we combine context-based and contrastive learning to comprehensively close the features on different domains. Experiments show that our HLA-Face v2 model obtains superior low-light face detection performance even without the use of low-light annotations. Moreover, our adaptation scheme can be extended to a wide range of applications, such as improving supervised learning and generic object detection. Project publicly available at:
https://daooshee.github.io/HLA-Face-v2-Website/
.
更多查看译文
关键词
Low-light,domain adaptation,illumination enhancement,high-level,low-level,face detection
AI 理解论文
溯源树
样例
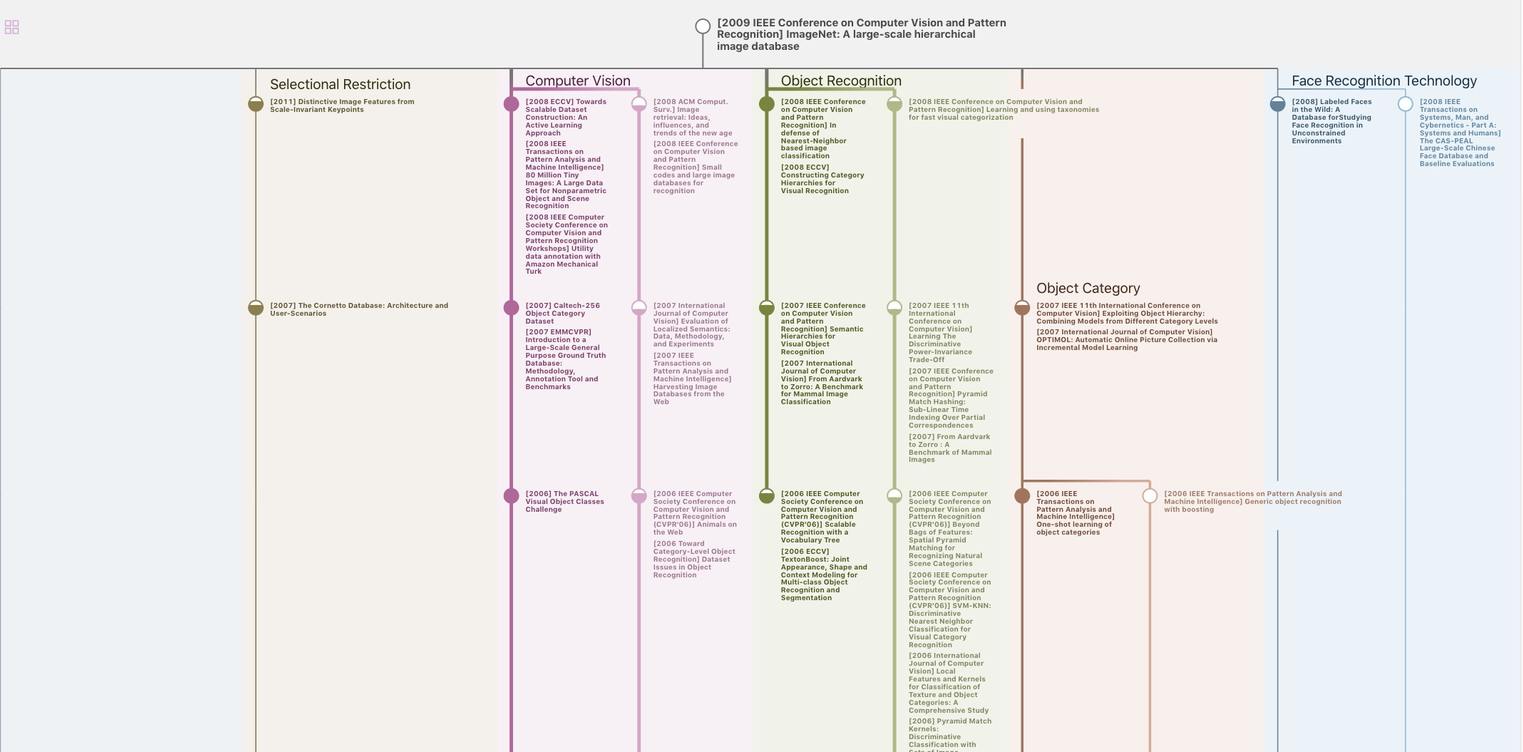
生成溯源树,研究论文发展脉络
Chat Paper
正在生成论文摘要