Rolling bearing fault diagnosis based on SSA optimized self-adaptive DBN
ISA Transactions(2022)
Abstract
Due to the structure of rolling bearings and the complexity of the operating environment, collected vibration signals tend to show strong non-stationary and time-varying characteristics. Extracting useful fault feature information from actual bearing vibration signals and identifying bearing faults is challenging. In this paper, an innovative optimized adaptive deep belief network (SADBN) is proposed to address the problem of rolling bearing fault identification. The DBN is pre-trained by the minimum batch stochastic gradient descent. Then, a back propagation neural network and conjugate gradient descent are used to supervise and fine-tune the entire DBN model, which effectively improve the classification accuracy of the DBN. The salp swarm algorithm, an intelligent optimization method, is used to optimize the DBN. Then, the experience of deep learning network structure is summarized. Finally, a series of simulations based on the experimental data verify the effectiveness of the proposed method.
MoreTranslated text
Key words
Deep belief network,Optimization design,Rolling bearing fault diagnosis,Salp swarm algorithm
AI Read Science
Must-Reading Tree
Example
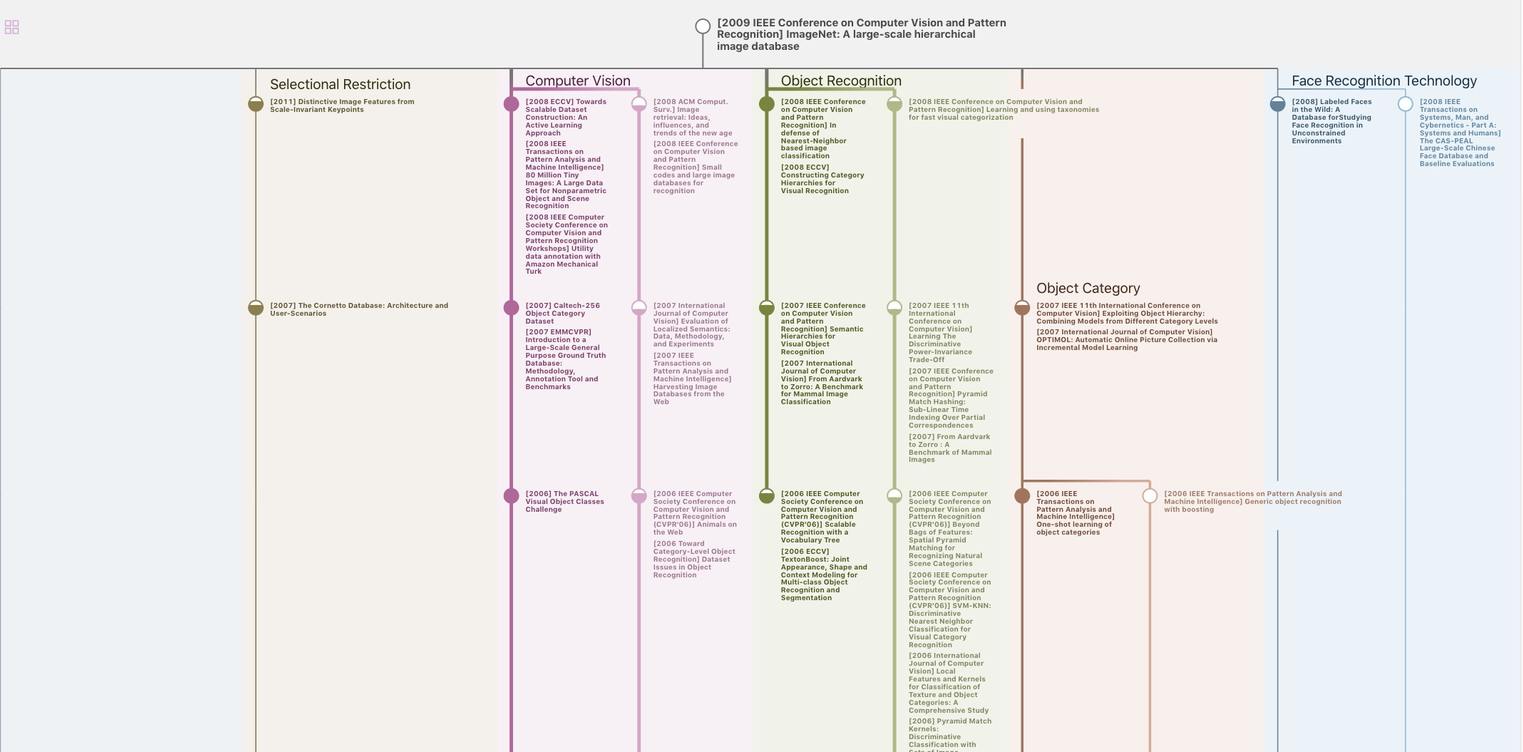
Generate MRT to find the research sequence of this paper
Chat Paper
Summary is being generated by the instructions you defined