The Data Efficiency of Deep Learning Is Degraded by Unnecessary Input Dimensions.
FRONTIERS IN COMPUTATIONAL NEUROSCIENCE(2022)
摘要
Biological learning systems are outstanding in their ability to learn from limited training data compared to the most successful learning machines, i.e., Deep Neural Networks (DNNs). What are the key aspects that underlie this data efficiency gap is an unresolved question at the core of biological and artificial intelligence. We hypothesize that one important aspect is that biological systems rely on mechanisms such as foveations in order to reduce unnecessary input dimensions for the task at hand, e.g., background in object recognition, while state-of-the-art DNNs do not. Datasets to train DNNs often contain such unnecessary input dimensions, and these lead to more trainable parameters. Yet, it is not clear whether this affects the DNNs' data efficiency because DNNs are robust to increasing the number of parameters in the hidden layers, and it is uncertain whether this holds true for the input layer. In this paper, we investigate the impact of unnecessary input dimensions on the DNNs data efficiency, namely, the amount of examples needed to achieve certain generalization performance. Our results show that unnecessary input dimensions that are task-unrelated substantially degrade data efficiency. This highlights the need for mechanisms that remove task-unrelated dimensions, such as foveation for image classification, in order to enable data efficiency gains.
更多查看译文
关键词
data efficiency, overparameterization, object recognition, object background, unnecessary input dimensions, deep learning
AI 理解论文
溯源树
样例
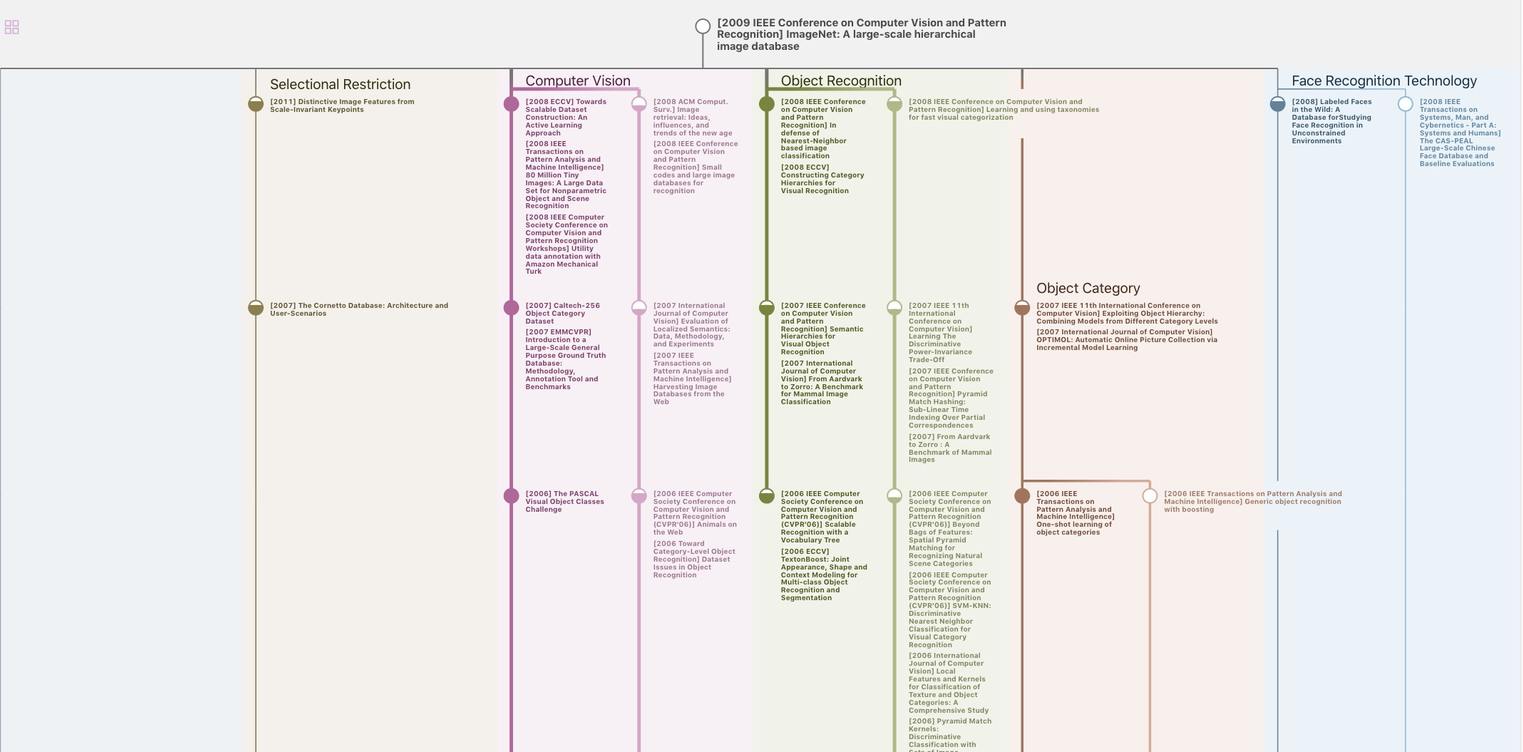
生成溯源树,研究论文发展脉络
Chat Paper
正在生成论文摘要