Recurrent Neural Networks Are Universal Approximators With Stochastic Inputs
IEEE transactions on neural networks and learning systems(2023)
摘要
In this article, we investigate the approximation ability of recurrent neural networks (RNNs) with stochastic inputs in state space model form. More explicitly, we prove that open dynamical systems with stochastic inputs can be well-approximated by a special class of RNNs under some natural assumptions, and the asymptotic approximation error has also been delicately analyzed as time goes to infinity. In addition, as an important application of this result, we construct an RNN-based filter and prove that it can well-approximate finite dimensional filters which include Kalman filter (KF) and Beneš filter as special cases. The efficiency of RNN-based filter has also been verified by two numerical experiments compared with optimal KF.
更多查看译文
关键词
Recurrent neural networks,Dynamical systems,Stochastic processes,Kalman filters,Electronic mail,Delays,Speech recognition,Dynamical systems with stochastic inputs,finite dimensional filter (FDF),Kalman filter (KF),recurrent neural networks (RNNs)
AI 理解论文
溯源树
样例
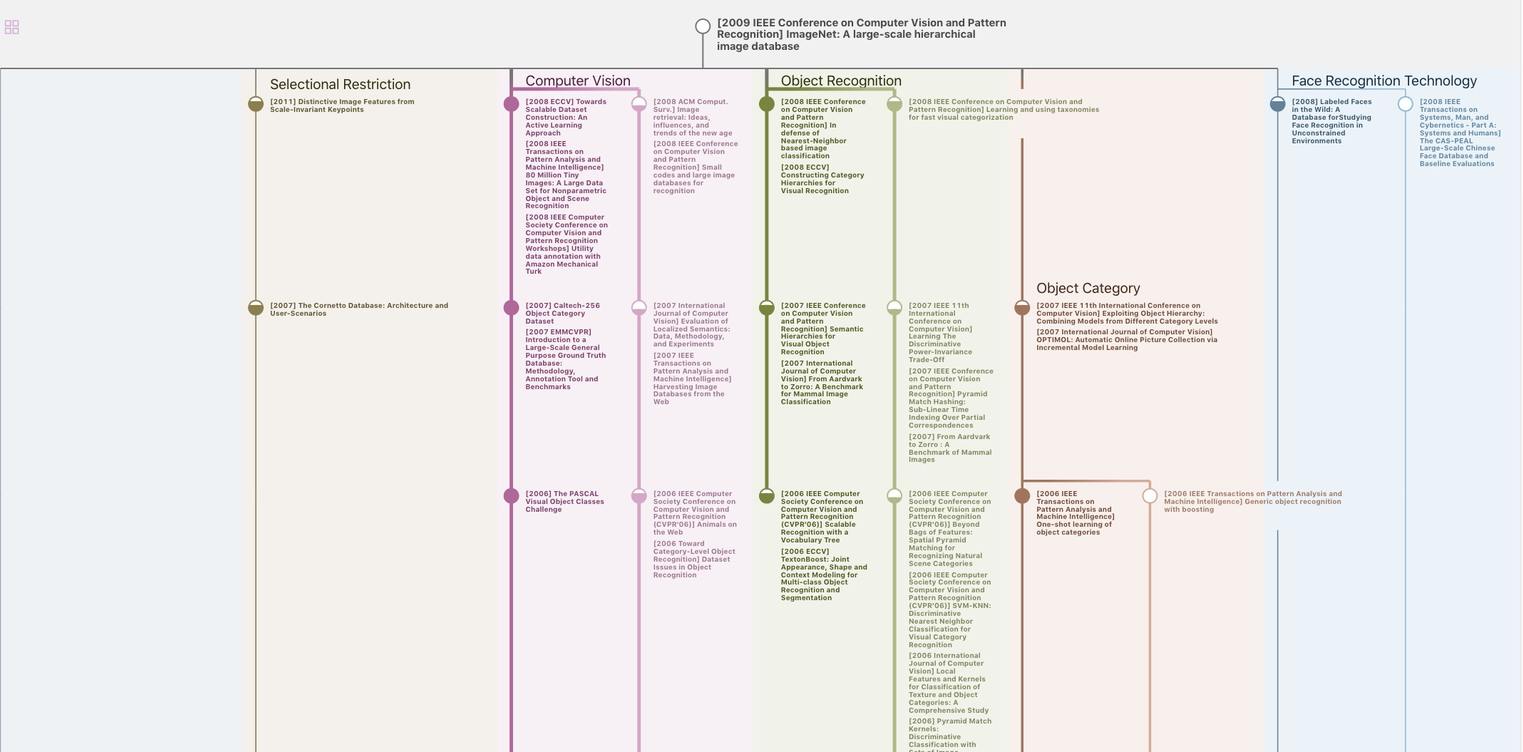
生成溯源树,研究论文发展脉络
Chat Paper
正在生成论文摘要