Conjugate priors and bias reduction for logistic regression models
arxiv(2022)
摘要
Logistic regression models for binomial responses are routinely used in statistical practice. However, the maximum likelihood estimate may not exist due to data separability. We address this issue by considering a conjugate prior penalty which always produces finite estimates. Such a specification has a clear Bayesian interpretation and enjoys several invariance properties, making it an appealing prior choice. We show that the proposed method leads to an accurate approximation of the reduced-bias approach of Firth (1993), resulting in estimators with smaller asymptotic bias than the maximum-likelihood and whose existence is always guaranteed. Moreover, the considered penalized likelihood can be expressed as a genuine likelihood, in which the original data are replaced with a collection of pseudo-counts. Hence, our approach may leverage well established and scalable algorithms for logistic regression. We compare our estimator with alternative reduced-bias methods, vastly improving their computational performance and achieving appealing inferential results.
更多查看译文
关键词
conjugate priors,bias reduction,models
AI 理解论文
溯源树
样例
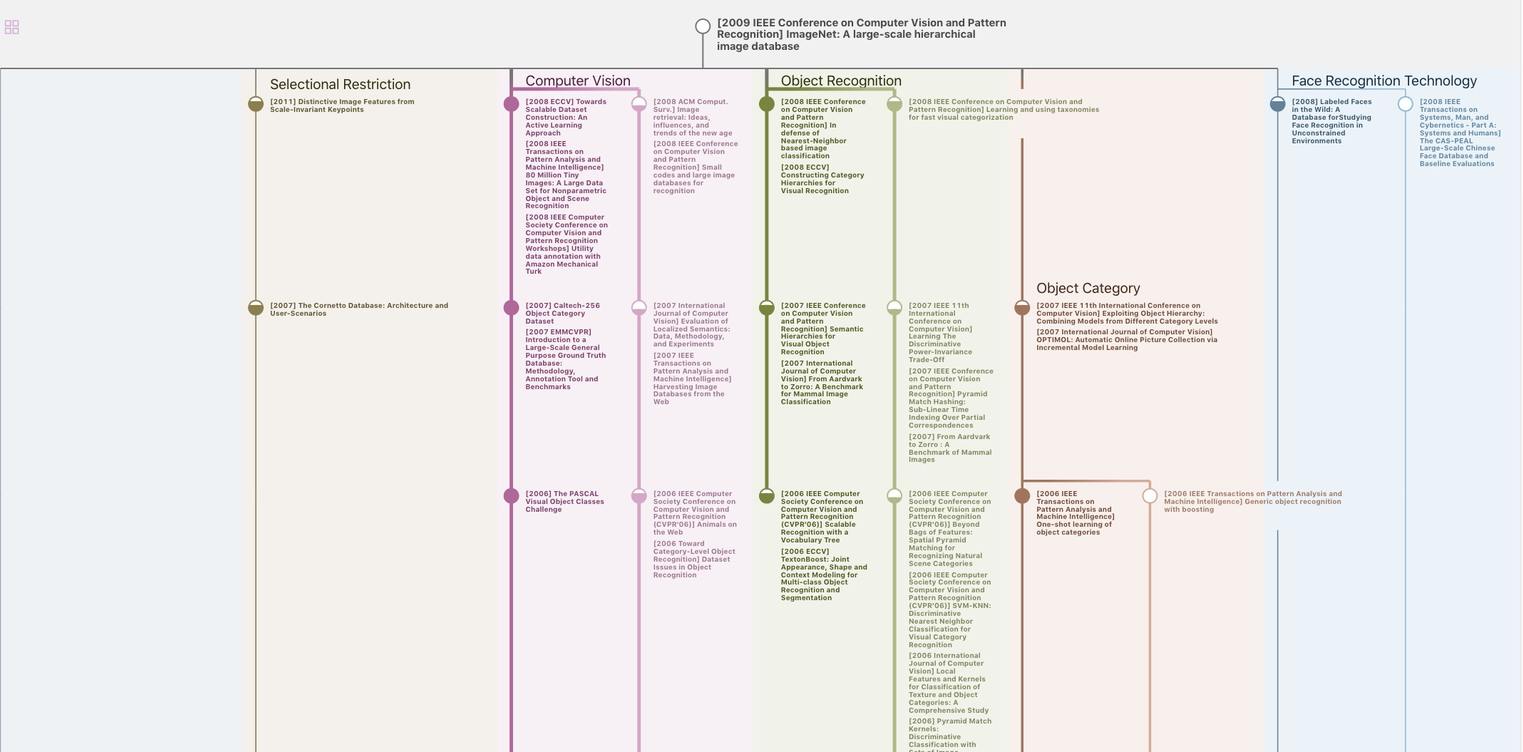
生成溯源树,研究论文发展脉络
Chat Paper
正在生成论文摘要