WiBFall: A Device-Free Fall Detection Model for Bathroom
MOBILE NETWORKS AND MANAGEMENT, MONAMI 2021(2022)
Abstract
Falling detection, especially for elderly people in confined areas such as bathrooms is vital for timely rescue. The mainstream vision-based fall detection approaches however are not applicable here for strong privacy concerns. It is therefore necessary to design a privacy-preserving fall detection model that utilizes other signals such as widely existed Wi-Fi for this scenario. Existing Wi-Fi based fall detection approaches often suffer from environment noise removal, resulting in moderate accuracy. In this paper, a Wi-Fi based fall detection model for bathroom environment, termed WiBFall, is proposed. Firstly, time series CSI data is reconstructed into a two-dimensional frequency energy map structure to obtain more feature capacity. Secondly, the reconstructed CSI data stream is filtered by Butterworth filter for noise elimination. Finally, the filtered data is used to train the established deep learning network to get a high accuracy fall detection model for bathroom. The experimental results show that the WiBFall not only reaches a fall detection accuracy of up to 99.63% in home bathroom environment, but also enjoys high robustness comparing to other schemes in different bathroom settings.
MoreTranslated text
Key words
Fall detection, Channel state information, Deep neural network
AI Read Science
Must-Reading Tree
Example
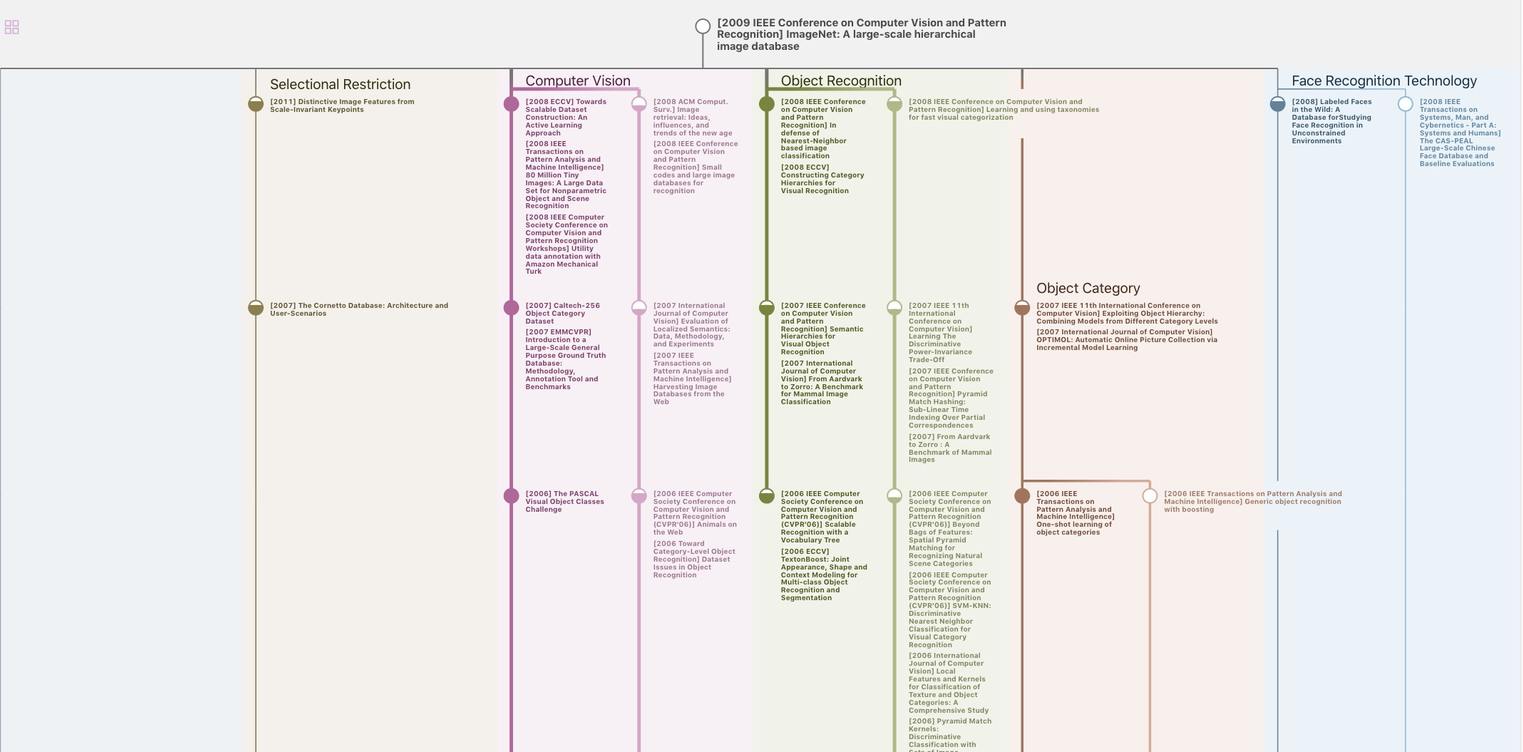
Generate MRT to find the research sequence of this paper
Chat Paper
Summary is being generated by the instructions you defined