DhakaNet: Unstructured Vehicle Detection using Limited Computational Resources
2021 21ST IEEE INTERNATIONAL CONFERENCE ON DATA MINING (ICDM 2021)(2021)
摘要
Inefficient traffic signal control system is one of the most important causes of traffic congestion in the cities of developing countries such as Bangladesh, India, Kenya, etc. This can be mitigated by adopting a decentralized traffic-responsive signal system, where vehicle detection is performed on the road through different image-based deep learning architectures amenable to limited-resource embedded platforms as available in developing countries. Deep learning architectures currently available in this regard demand high computational resources to achieve higher inference speed and better accuracy. Besides, the few existing limited-resource deep learning architectural alternatives neither attain higher inference speed nor substantial accuracy due to not overcoming the inherent limitations. To this extent, in this study, we propose a novel limited-resource deep learning architecture, namely DhakaNet, for real-time vehicle detection in on-road (street-view) traffic images. Our proposed architecture leverages enhancing Cross-Stage Partial Network and Path Aggregation Network to build the backbone and head networks, respectively. Besides, we develop a novel multi-scale attention module to extract multi-scale meaningful features from the images, where the developed multi-scale attention module boosts the detection accuracy at the cost of small overhead. Rigorous experimental evaluation of our proposed DhakaNet over three benchmark street-view traffic datasets such as DhakaAI, IITM-HeTra-A, and IITM-HeTra-B shows up to 51% faster inference speed at a similar accuracy, or up to 13% higher accuracy at a similar inference speed compared to other state-of-the-art limited-resource deep learning architectural alternatives.
更多查看译文
关键词
Traffic congestion, Vehicle detection, Deep learning, Computer vision, Embedded systems
AI 理解论文
溯源树
样例
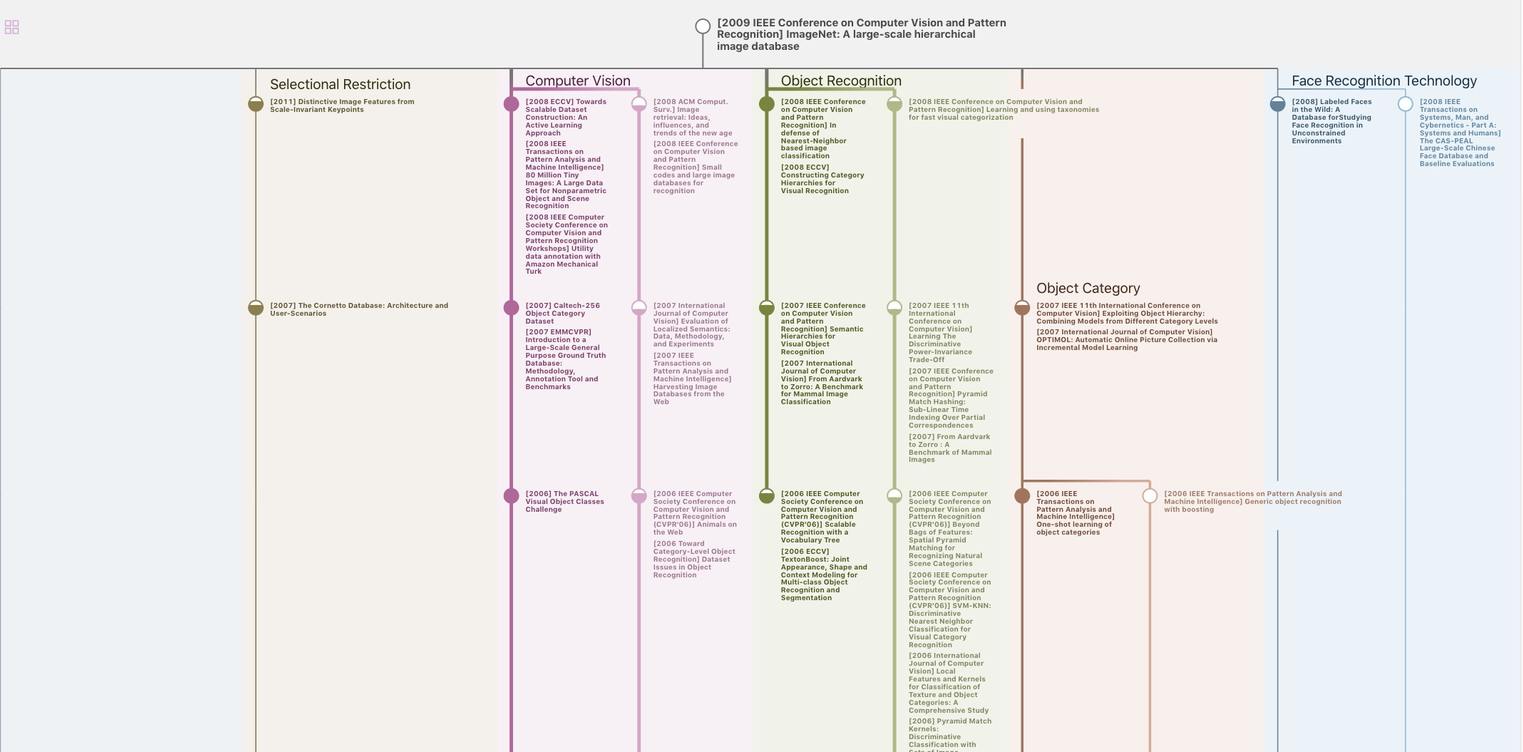
生成溯源树,研究论文发展脉络
Chat Paper
正在生成论文摘要