Performance Comparison of 1D and 2D Convolutional Neural Networks for Real-Time Classification of Time Series Sensor Data
36TH INTERNATIONAL CONFERENCE ON INFORMATION NETWORKING (ICOIN 2022)(2022)
摘要
Two-dimensional (2D) convolutional neural networks (CNNs) are implemented for machinery fault diagnosis owing to CNN's capability of feature extraction from input data. One-dimensional (1D) signals are converted to two-dimensional (2D) data because 2D image data is a much more powerful information representation. However, the computation complexity of 2D CNN is high due to the 2D operation on many stages, and it requires more data to achieve higher training performance. 1D CNNs are implemented to utilize 1D signal directly, which reduces the computational complexity and enables real-time classification. In this paper, we compare the performance of the 1D CNN and 2D CNN for the multi-class classification of time-series sensor data. The architecture of each CNN considered in this work has a single convolutional layer and one fully connected layer. Real measured data are used for the training and testing of the models. 1D signal extracted from sensor signal is converted into 2D data array for the input of the 2D CNN. Classification accuracy and time complexity of both CNNs are evaluated for a given dataset. Via simulations, we show that both CNNs classify the time series data with high accuracy, achieve approximately the same classification performance.
更多查看译文
关键词
convolutional neural network, classification, time-series data
AI 理解论文
溯源树
样例
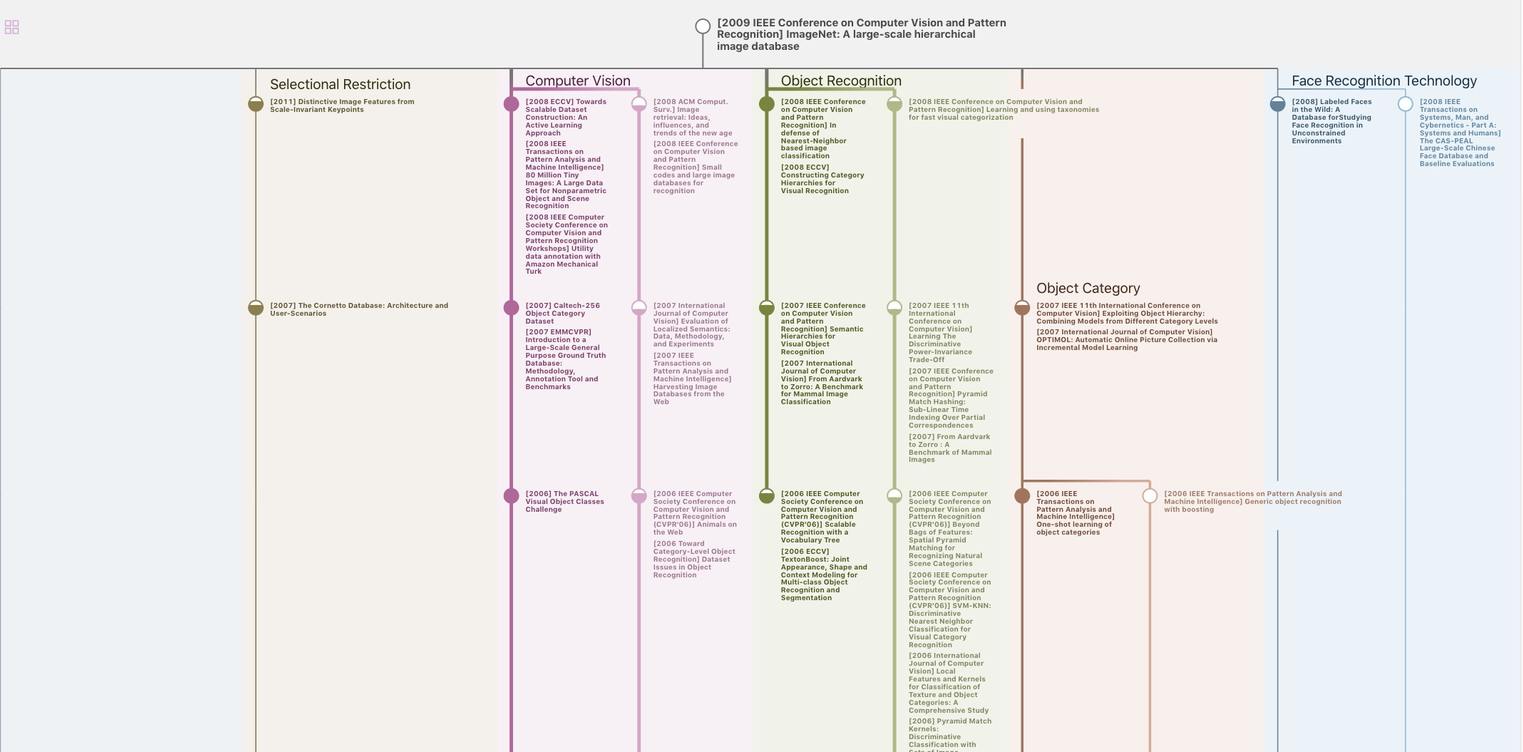
生成溯源树,研究论文发展脉络
Chat Paper
正在生成论文摘要