Cross Network Representation Matching with Outliers.
ICDM(2021)
摘要
Research has revealed the effectiveness of network representation techniques in handling diverse downstream machine learning tasks upon graph structured data. However, most network representation methods only seek to learn information in a single network, which fails to learn knowledge across different networks. Moreover, outliers in real-world networks pose great challenges to match distribution shift of learned embeddings. In this paper, we propose a novel joint learning framework, called CrossOSR, to learn network-invariant embeddings across different networks in the presence of outliers in the source network. To learn outlier-aware representations, a modified graph convolutional network (GCN) layer is designed to indicate the potential outliers. To learn more fine-grained information between different domains, a subdomain matching is adopted to align the shift distribution of learned vectors. To learn robust network representations, the learned indicator is utilized to smooth the noise effect from source domain to target domain. Extensive experimental results on three real-world datasets in the node classification task show that the proposed framework yields state-of-the-art cross network representation matching performance with outliers in the source network.
更多查看译文
关键词
network representation,subdomain matching,outlier,graph data,graph learning
AI 理解论文
溯源树
样例
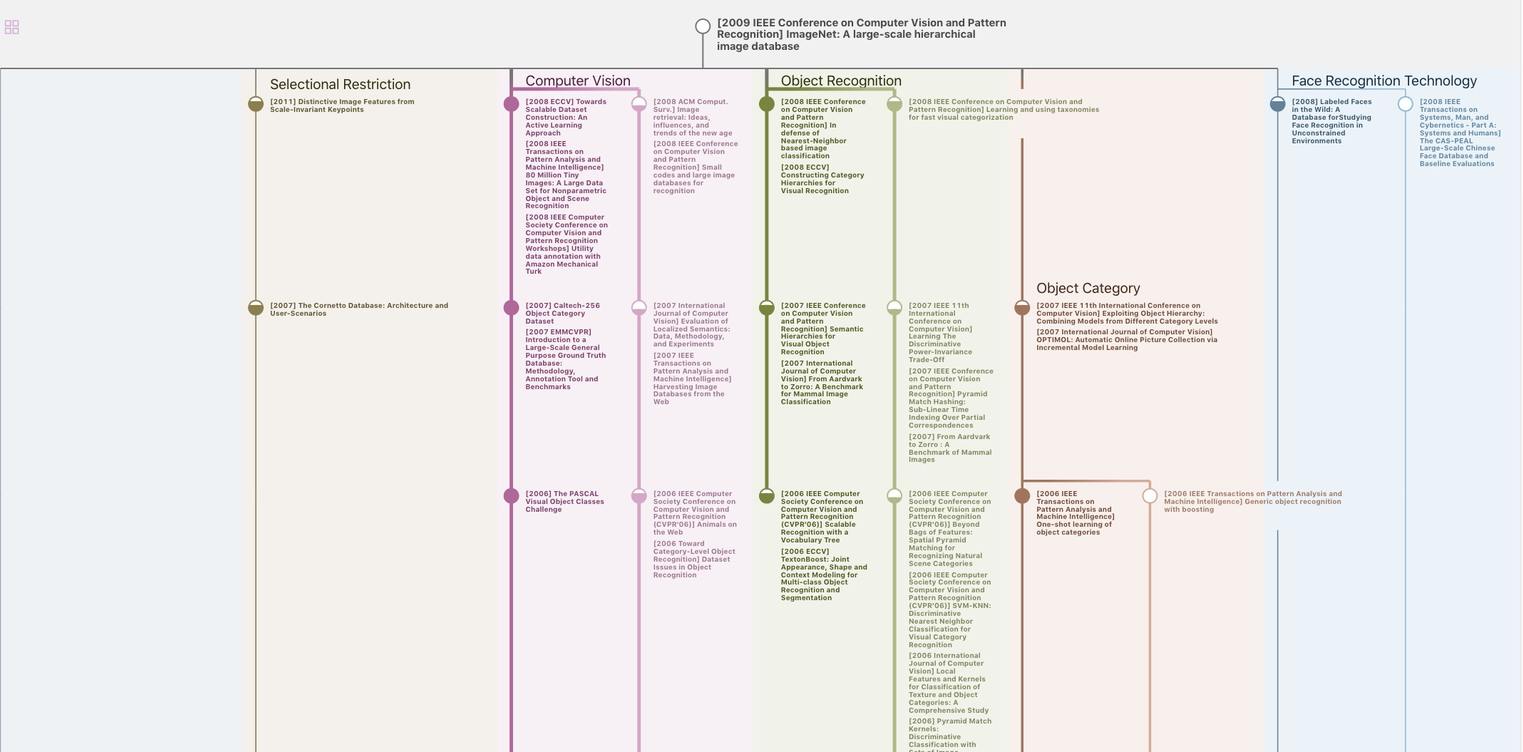
生成溯源树,研究论文发展脉络
Chat Paper
正在生成论文摘要