Multi-objective Mobile Charging Scheduling on the Internet of Electric Vehicles: a DRL Approach
2021 IEEE GLOBAL COMMUNICATIONS CONFERENCE (GLOBECOM)(2021)
摘要
Mobile charging services (MCSs) have been developed as a supplement charging method for electric vehicles (EVs), wherein energy replenishment is provided by mobile charging vehicles (MCVs). An MCV has an internal storage system employed to replenish the energy of a certain number of EVs. Charging scheduling of MCV is one of the key issues on the Internet of EVs for providing efficient and convenient charging services, which requires determining the charging sequence and the amount of energy when serving multiple EVs by one MCV. In this paper, a multi-objective MCV scheduling problem is investigated. By optimizing the charging sequence and the actual amount of energy being charged, the proposed framework aims to minimize the EV waiting time while simultaneously to maximize the charging benefits of all EVs. To solve the multi-objective optimization problem (MOP), a deep reinforcement learning (DRL) based framework is further explored. The MOP is first decomposed into a set of subproblems. Each subproblem is modelled as a neural network, wherein an actor-critic algorithm and a modified pointer network are adopted to solve each subproblem. Pareto optimal solutions can be directly obtained through the trained models. The experimental results demonstrate that the proposed method can efficiently and effectively solve the MCV scheduling problem and outperform NSGA-II and MOEA/D in terms of solution convergence, solution diversity, and computing time. In addition, the trained model can be applied to newly encountered problems without retraining.
更多查看译文
关键词
internal storage system,multiobjective MCV scheduling problem,EV waiting time,multiobjective optimization problem,deep reinforcement learning based framework,pareto optimal solutions,multiobjective mobile charging scheduling,electric vehicles,DRL approach,deep reinforcement learning,neural network
AI 理解论文
溯源树
样例
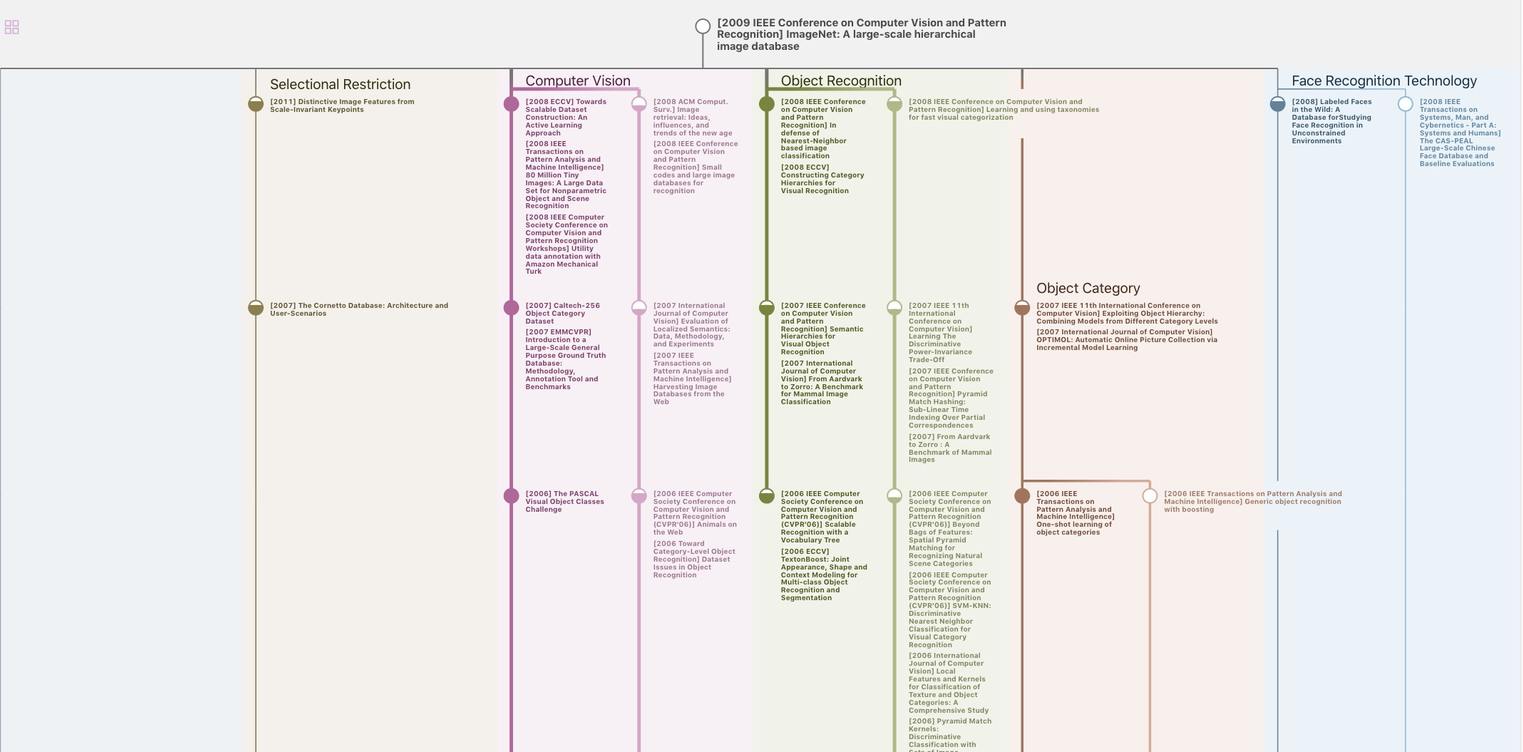
生成溯源树,研究论文发展脉络
Chat Paper
正在生成论文摘要