Deep Learning-based QoS Prediction with Innate Knowledge of the Radio Access Network
2021 IEEE GLOBAL COMMUNICATIONS CONFERENCE (GLOBECOM)(2021)
摘要
To enable safe and advanced cooperative driving, quality of service (QoS) prediction in the radio access network has triggered recent attention to gracefully adapt vehicle-to-everything (V2X) applications to conform to expected network performance prior to the actual change in QoS. However, the communication and computation overhead combined with additional factors such as privacy may affect training data availability. Innate knowledge of wireless communication in the QoS prediction model could help improve prediction performance in environments with reduced training data availability. This paper presents a heuristic approach to explicitly incorporate prior knowledge to a deep learning-based QoS prediction model in the form of wireless communication-based penalties in the cost function of a deep neural network. System level simulations for the teleoperated driving (TOD) use case are used to evaluate our proposal. Results show that incorporating cell-load, channel and uplink inter-cell interference penalties in a deep neural network (DNN) improve uplink data rate prediction performance in scenarios with reduced amount of training data compared to an off-the-shelf DNN.
更多查看译文
关键词
QoS prediction, deep learning, machine learning, prior knowledge, V2X
AI 理解论文
溯源树
样例
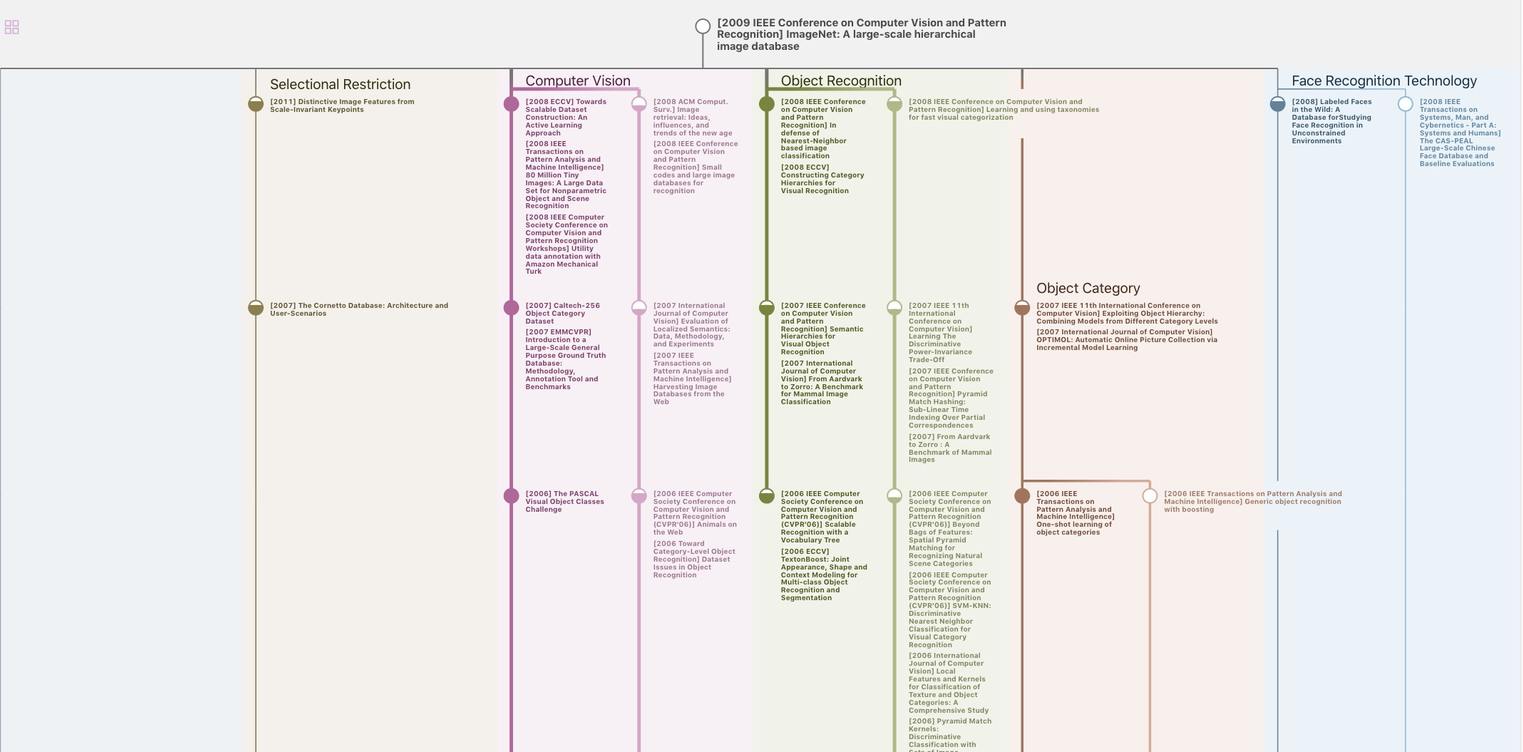
生成溯源树,研究论文发展脉络
Chat Paper
正在生成论文摘要