Adversarial Learning for Hiding Wireless Signals
2021 IEEE GLOBAL COMMUNICATIONS CONFERENCE (GLOBECOM)(2021)
Abstract
Spectrum access in the next generation congested cognitive wireless networks will be vulnerable to malicious intents of strong adversaries. One of the ways to obscure an adversary is to hide a signal to avoid the threats of being intercepted or jammed. In this paper, we present such a wireless steganography technique, where we hide the secret message as a form of noise. We use adversarial learning model to transform the domain of secret message, from bits to complex signal that is statistically identical to hardware noise of a transmitter. A three-node neural network, an encoder, a decoder, and a critic (steganalyzer), is optimized jointly to encode and decode a secret message while adhering to statistically identical properties of the encoded covert signal and hardware generated noise. Once the domain transfer is complete, this covert signal can be carried by any cover signal, independent of its waveform or modulation order. We provide an information-theoretic analysis to evaluate the embedding capability of the learning model. Our results show that the covert signal achieves a throughput of 12Mbps at 12dB SNR and is resilient to different levels of hardware noise.
MoreTranslated text
Key words
Wireless steganography, Adversarial Learning, Information Theoretic analysis
AI Read Science
Must-Reading Tree
Example
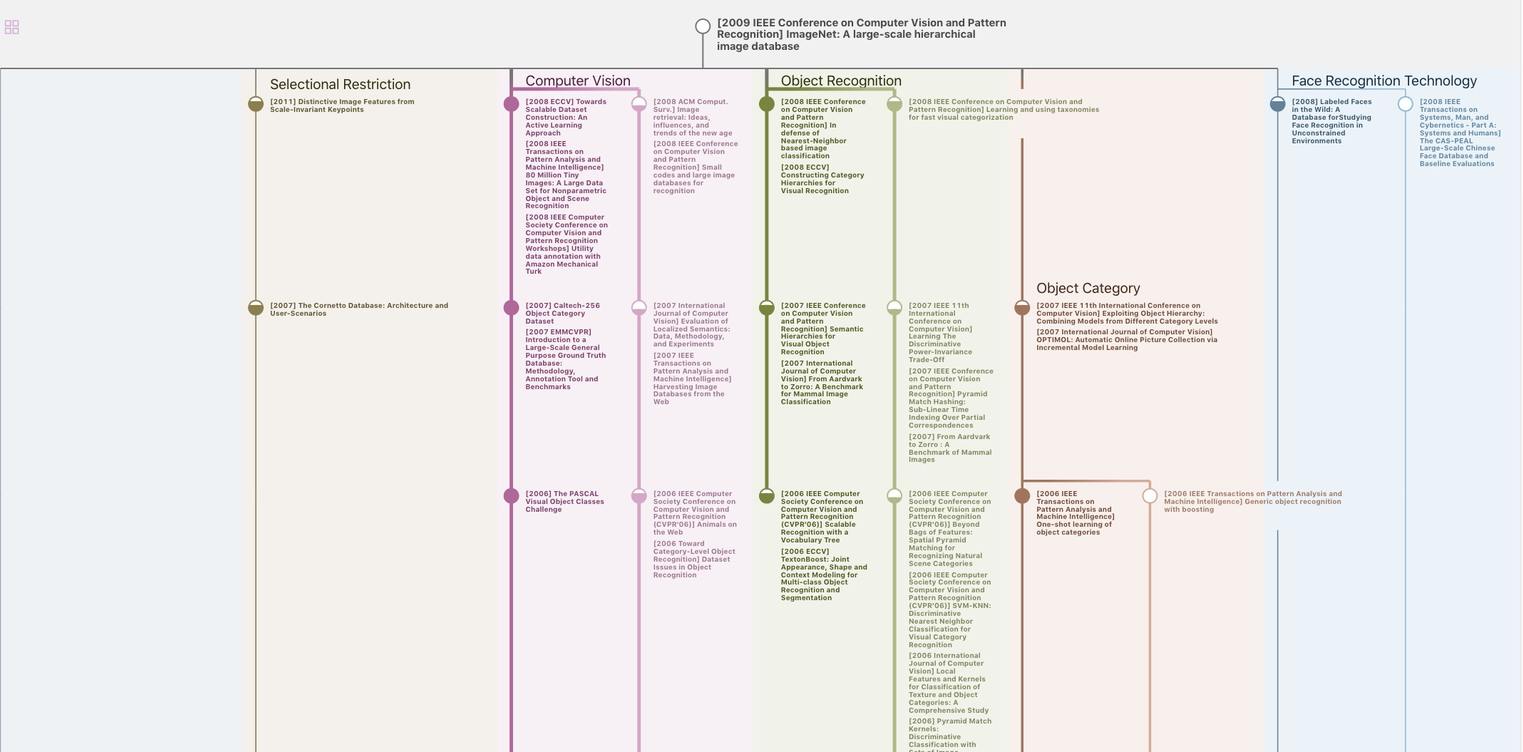
Generate MRT to find the research sequence of this paper
Chat Paper
Summary is being generated by the instructions you defined