A Deep Learning Framework for Distributed Channel Selection in a Congested Uncooperative Spectrum
2021 IEEE GLOBAL COMMUNICATIONS CONFERENCE (GLOBECOM)(2021)
摘要
In this paper, a deep learning framework for distributed interference assessment and channel selection in a congested, uncooperative spectrum is proposed. We present a deep learning based spectrum sensing model that is trained to estimate channel quality. Such training is conducted offline in a supervised manner using labeled channel energy captures and their associated packet error rate measurements. Packet error rate estimates are derived from numerical simulations of the system model. These estimates can be used to take action on network channel selection. Distributed application of our model is efficiently realized through online training at the level of edge devices and then the trained local models are periodically aggregated using federated learning. The model views packet error rate assessment as a classification problem, where the packet error rate is assigned a class based on a set of classes with upper and lower bounds. The trained models are tested against two metrics: classification accuracy and the mean ratio of error rate in the optimal operating channel to that in a channel selected based on classification inference. In the simulation scenarios, the model when trained in a centralized manner has a classification accuracy of 91.9% and a mean selection error rate ratio 91.3%, averaged over all scenarios. When trained in a distributed manner, the model has a classification accuracy of 83.3% and a mean selection error rate ratio of 88.6% The distributed approach is estimated to reduce the communications overhead by two orders of magnitude to approximately 34 MB per edge device.
更多查看译文
关键词
Interference mitigation,Channel selection,Deep learning,Uncooperative spectrum,Federated learning
AI 理解论文
溯源树
样例
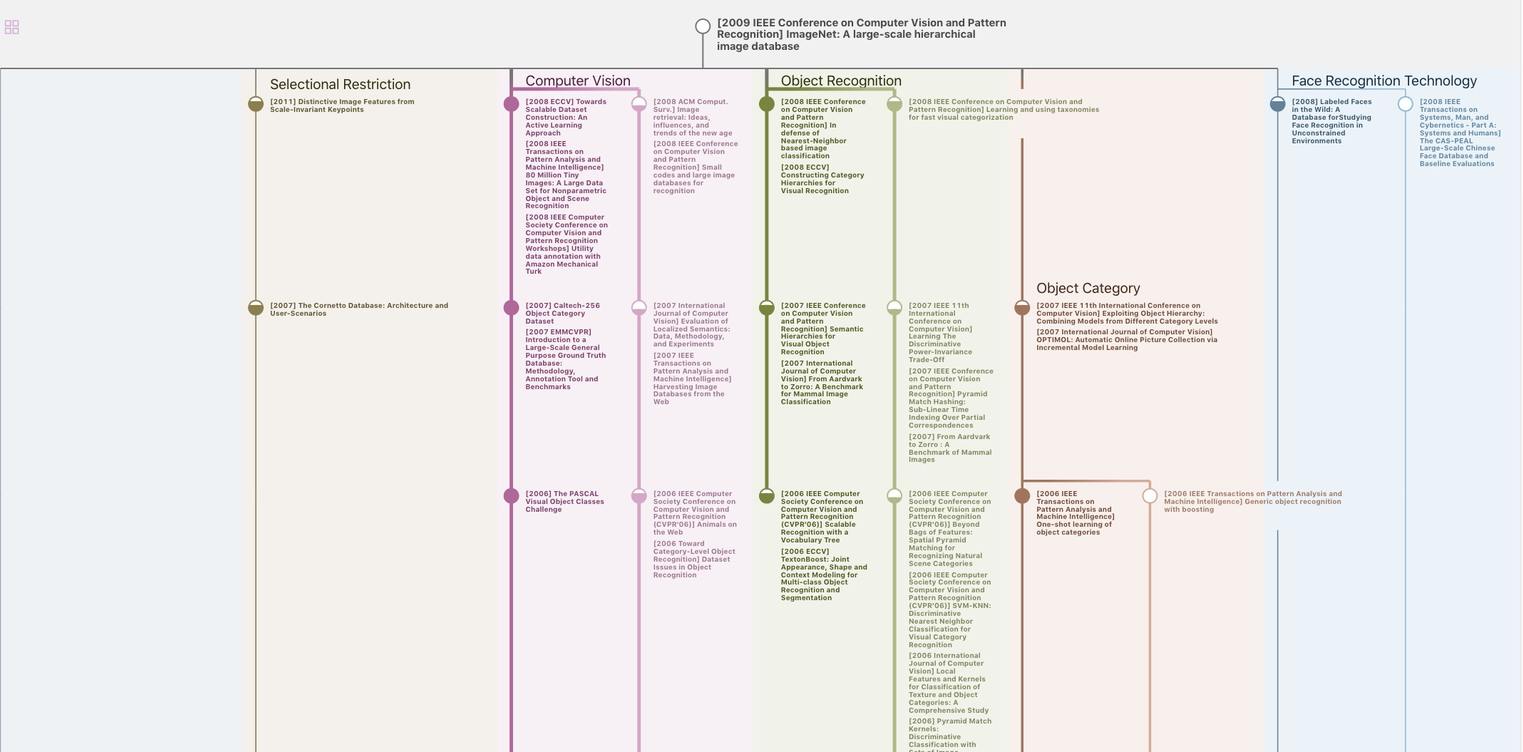
生成溯源树,研究论文发展脉络
Chat Paper
正在生成论文摘要