A Privacy-preserved D2D Caching Scheme Underpinned by Blockchain-enabled Federated Learning
2021 IEEE GLOBAL COMMUNICATIONS CONFERENCE (GLOBECOM)(2021)
Abstract
Cache-enabled device-to-device (D2D) communication has been widely deemed as a promising approach to tackle the unprecedented growth of wireless traffic demands. Recently, tremendous efforts have been put into designing an efficient caching policy to provide users better quality of service. However, public concerns of data privacy still remain in D2D cache sharing networks, which thus arises an urgent need for a privacy-preserved caching scheme. In this study, we propose a double-layer blockchain-based federated learning (DBFL) scheme with the aim of minimizing the download latency for all users in a privacy-preserving manner. Specifically, in the sublayer, the devices within the same coverage area run a federated learning (FL) to train the caching scheme model for each area separately without exchange of local data. The model parameters for each area are recorded in sublayer chains with Raft consensus mechanism. Meanwhile, in the main layer, a mainchain based on practical Byzantine fault tolerance (PBFT) mechanism is used to resist faults and attacks, thus securing the reliability of FL updates. Only the reliable area models authorized by the mainchain are utilized to update the global model in the main layer. Numerical results show the convergence, as well as the gain of download latency of the proposed DBFL caching scheme when compared with several traditional schemes.
MoreTranslated text
Key words
D2D Caching, Federated Learning, Blockchain
AI Read Science
Must-Reading Tree
Example
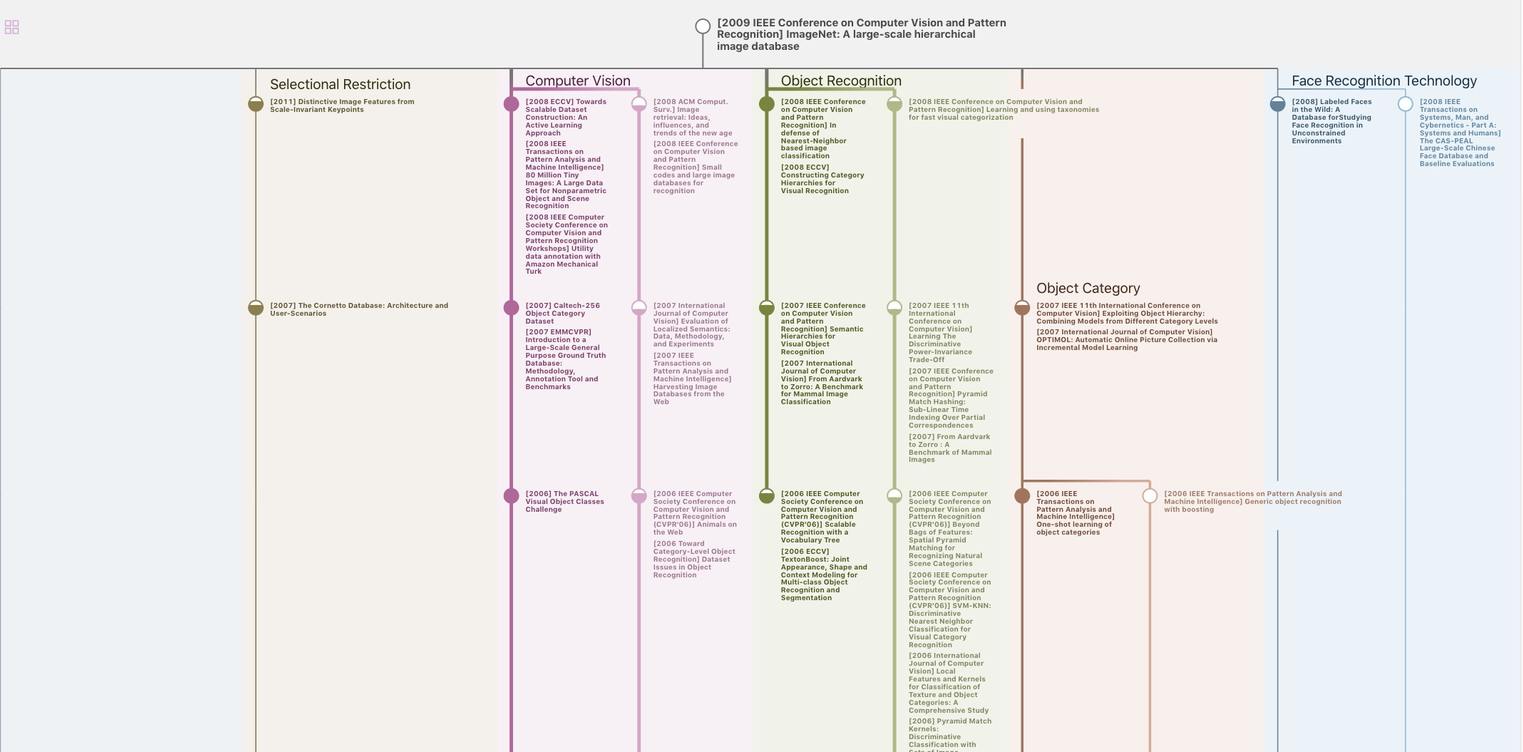
Generate MRT to find the research sequence of this paper
Chat Paper
Summary is being generated by the instructions you defined