All Predict Wisest Decides: A Novel Ensemble Method to Detect Intrusive Traffic in IoT Networks
2021 IEEE GLOBAL COMMUNICATIONS CONFERENCE (GLOBECOM)(2021)
摘要
Internet of things (IoT) networks confront various network intrusion threats due to massively interconnected nodes that form an extensive attack surface for adversaries. Machine learning (ML)-based approaches are widely investigated to address network intrusions. It becomes further challenging to achieve promising performance for multi-class classification so to identify each attack type rather than detection of the presence of intrusion, which involves binary classification. ML models perform divergent detection performance in each class, so it is challenging to select one ML model applicable to all classes prediction. With this in mind, we propose an innovative ensemble learning framework, namely All Predict Wisest Decides (APWD) that builds on training of multiple ML models and testing them independently so to obtain prediction performance for all classes. For each attack category, an expert (i.e., wisest) model that performs the best F1 score, accuracy, lowest false detection rate is determined according to individual model results. The aggregation module makes decisions relying upon the wisest model determined for each class. APWD is a generic framework, and the types of MLs and the number of MLs can be customized in APWD. Experiments under a popular public dataset, NSL-KDD verify the proposed approach APWD by demonstrating that APWD boosts overall accuracy to 0.797, comparing 0.772 by XGBoost, 0.758 by RF, and 0.584 by Adaboost. Moreover, in certain attack types R2L, APWD increases F1 score by a factor of 18, from 0.022 by RF to 0.421.
更多查看译文
关键词
Internet of Things, Intrusion Detection, Machine Learning, Ensemble Learning
AI 理解论文
溯源树
样例
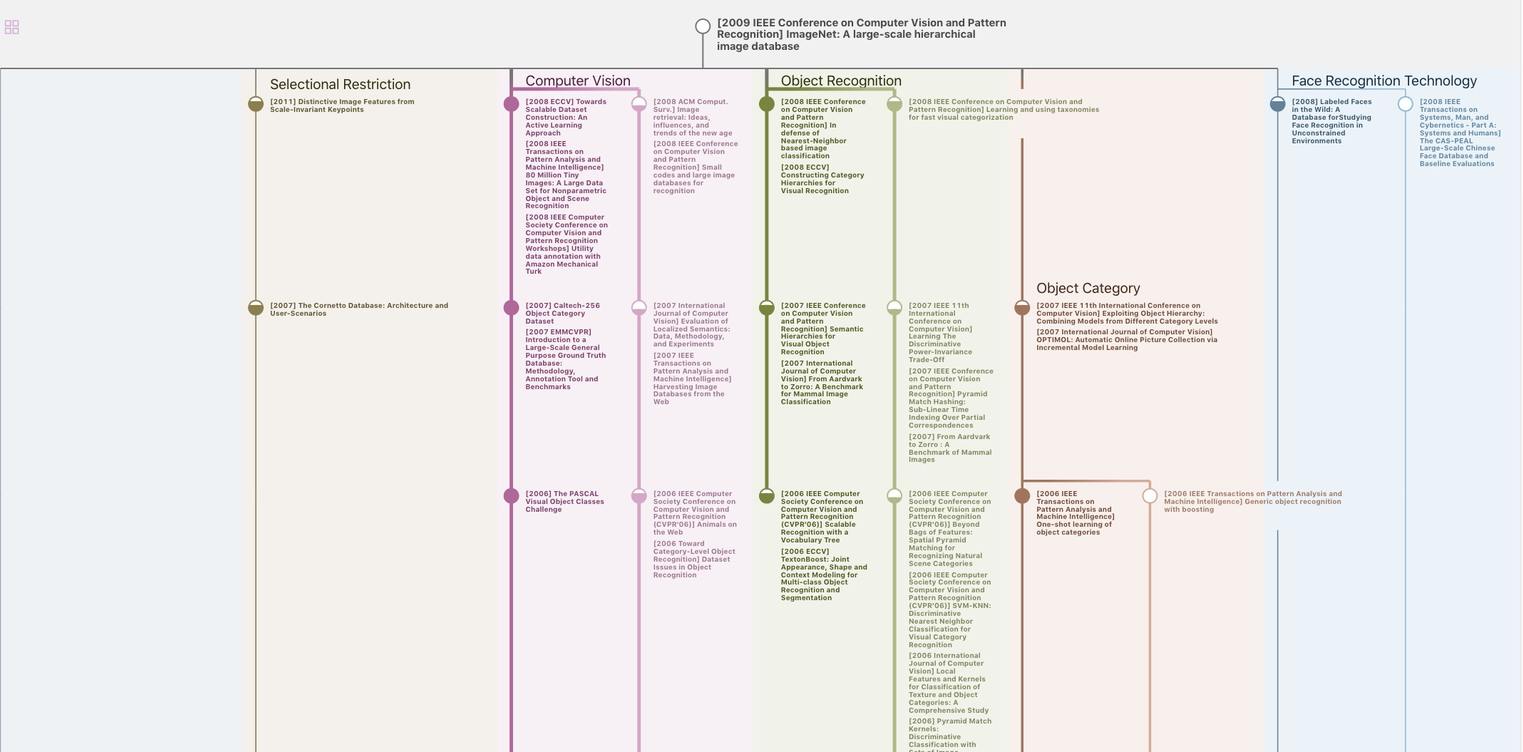
生成溯源树,研究论文发展脉络
Chat Paper
正在生成论文摘要