Pri-PGD: Forging privacy-preserving graph towards spectral-based graph neural network
2021 IEEE GLOBAL COMMUNICATIONS CONFERENCE (GLOBECOM)(2021)
摘要
The development of Graph Neural Network (GNN) enables people to explore the value of graph data better. Additionally, graph data often reveals more private information than data in Euclidean space. Some scholars have proposed that differential privacy can be used to add noise to the graph. However, the availability of the data will decrease with the increase of privacy. Some work utilize graph data through federated learning, which is computationally complex and heavily burdened by communication. Therefore, based on the principle of the spectral-based GNN model (Graph convolutional network, GCN), we proposed a method (Pri-PGD) to forge a privacy-preserving graph by disturbing. We set a user-selectable parameter m to convert the first m-order neighbor nodes to the first-order neighbor for each node. Pri-PGD achieves two goals: preserving convolution basis space and preserving first-order neighbor information of the real graph. This allows Pri-PGD to guarantee the privacy of the graph data while keeping the availability of it. We evaluated the effectiveness of our algorithm on three classical graph datasets (Cora, Citeseer, Pubmed). The accuracy of our method on the GCN model for the three datasets decreases only by about 1% when the parameter m is 2. This verifies the validity of the forged graph obtained by Pri-PGD. As the experiment shows, our method is also valid for the Graph attention network model that is sensitive to first-order neighbor nodes.
更多查看译文
关键词
privacy-preserving graph, Graph neural network, spectral
AI 理解论文
溯源树
样例
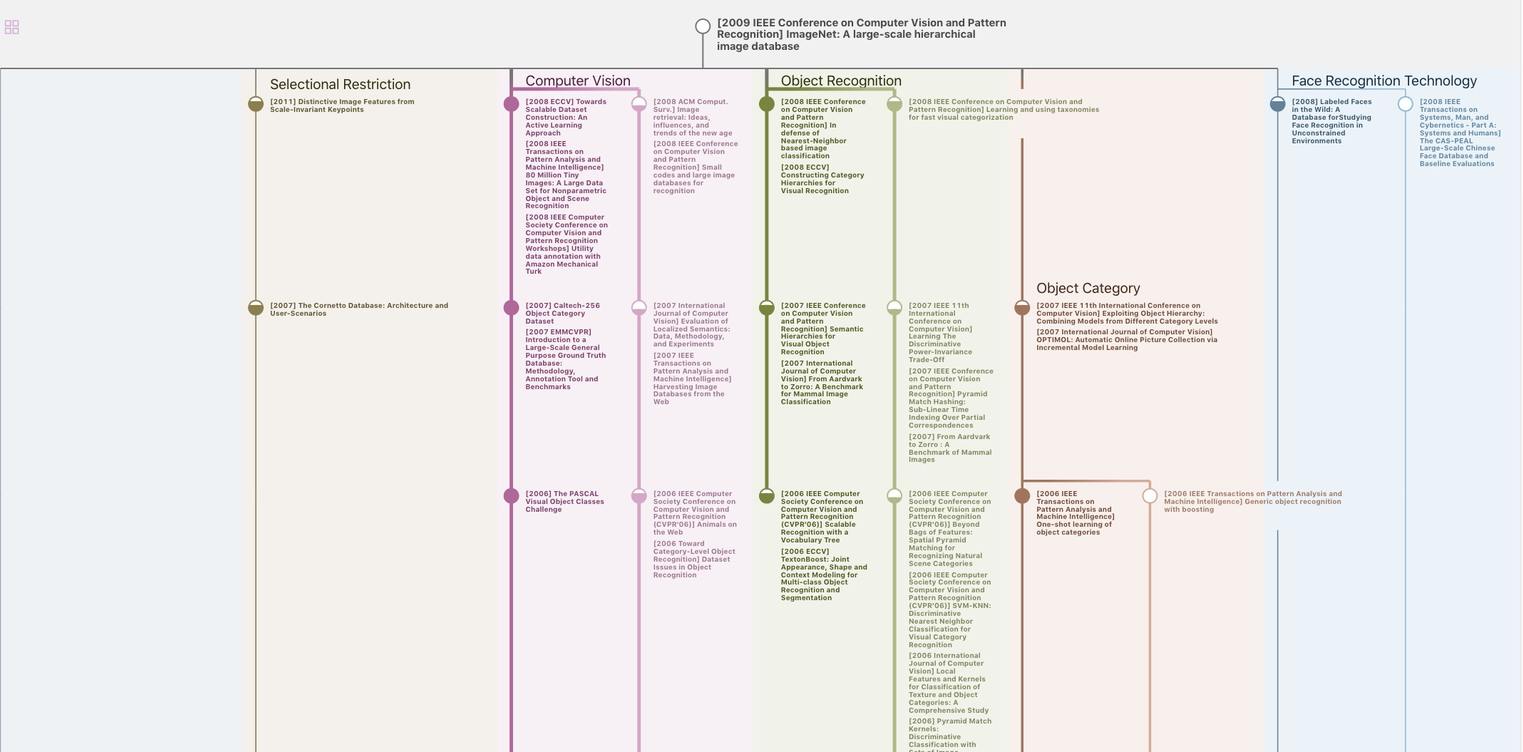
生成溯源树,研究论文发展脉络
Chat Paper
正在生成论文摘要