Deep Features Fusion with Mutual Attention Transformer for Skin Lesion Diagnosis.
ICIP(2021)
摘要
Early skin lesion diagnosis is crucial to prevent skin cancer, and deep learning (DL) based methods are well exploited to support dermatologists' diagnosis. The data for the diagnosis tasks include dermoscopic lesion images and textual information. It is a challenge to learn features from the multi-modal data to improve diagnostic quality. Inspired by the vision and language integration models in Visual Question Answer (VQA), we present an end-to-end neural network model for skin lesion diagnosis using both images and textual information simultaneously. Specifically, we fine-grained features from the two modalities (image and text) of the dataset by the pre-trained DL models. We propose a novel approach named Mutual Attention Transformer (MAT), which consists of self-attention blocks and guided-attention blocks, to enable the interactions between the features from both modalities concurrently. We then develop a fusion mechanism to integrate the represented features before the final classification output layer. The experimental results on the HAM10000 dataset demonstrate that the proposed method outperforms the state-of-art methods for skin lesion diagnosis.
更多查看译文
关键词
skin lesion classification,attention mechanism,transformer,deep learning
AI 理解论文
溯源树
样例
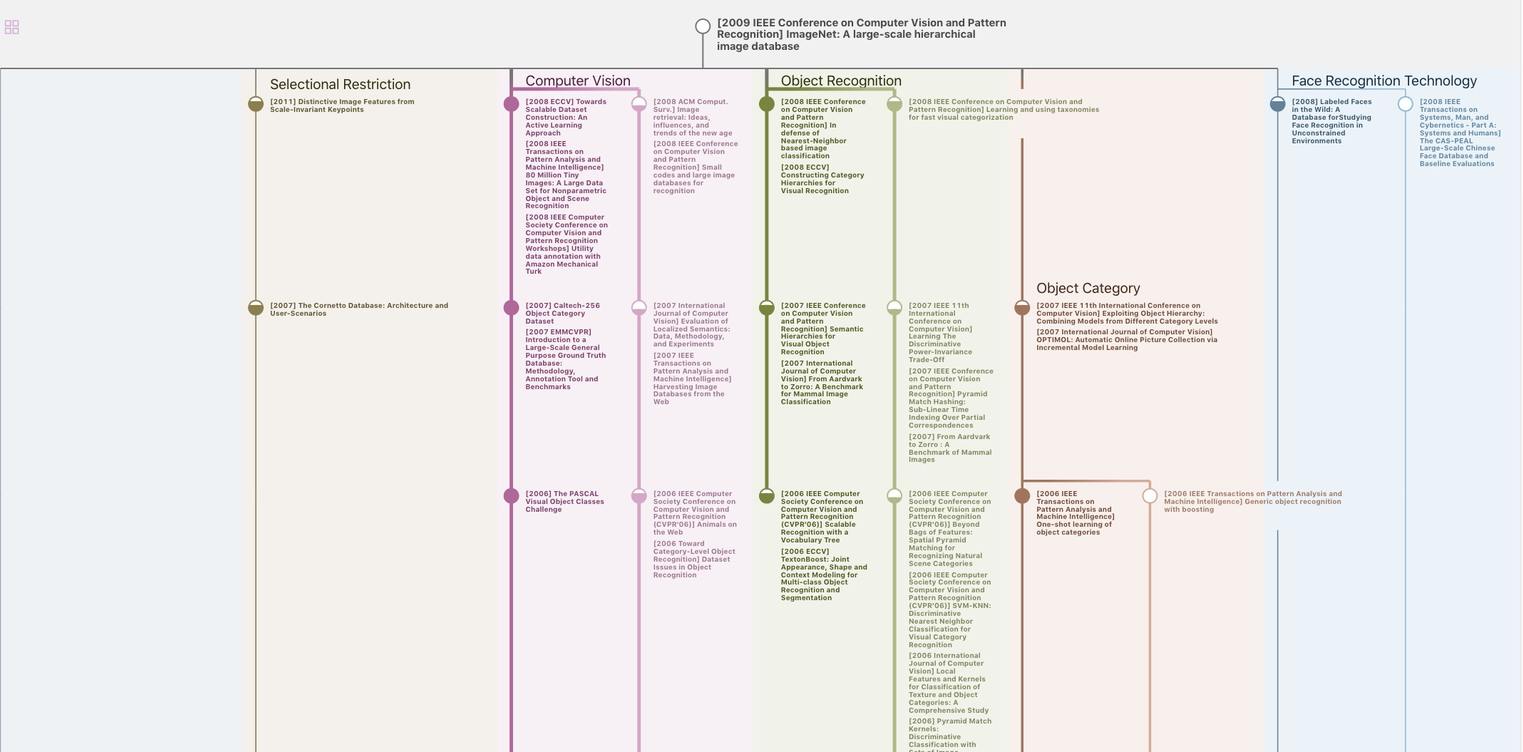
生成溯源树,研究论文发展脉络
Chat Paper
正在生成论文摘要