Enhancing Multi-Step Action Prediction for Active Object Detection.
ICIP(2021)
摘要
Active vision for robots is one promising solution to open world visual detection problems. A fundamental issue is view planning, i.e., predicting next best views to capture images of interest to reduce uncertainty. While multi-step action in a reinforcement learning (RL) setup can boost the efficiency of view planning, existing methods suffer from unstable detection outcome when the Q-values of multiple branches of action advantages (i.e., action range and action type) are combined naively. To tackle this issue, we propose a novel mechanism to disentangle action range from action type through a two-stage training strategy on a deep Q-network. It combines well-crafted loss functions with respect to action range and action type to enforce separated training of these two branches. We evaluate our method on two public datasets and show that it facilitates substantial gain in view planning efficiency, while enhancing detection accuracy.
更多查看译文
关键词
active object detection,reinforcement learning,view planning,deep q-learning network (DQN)
AI 理解论文
溯源树
样例
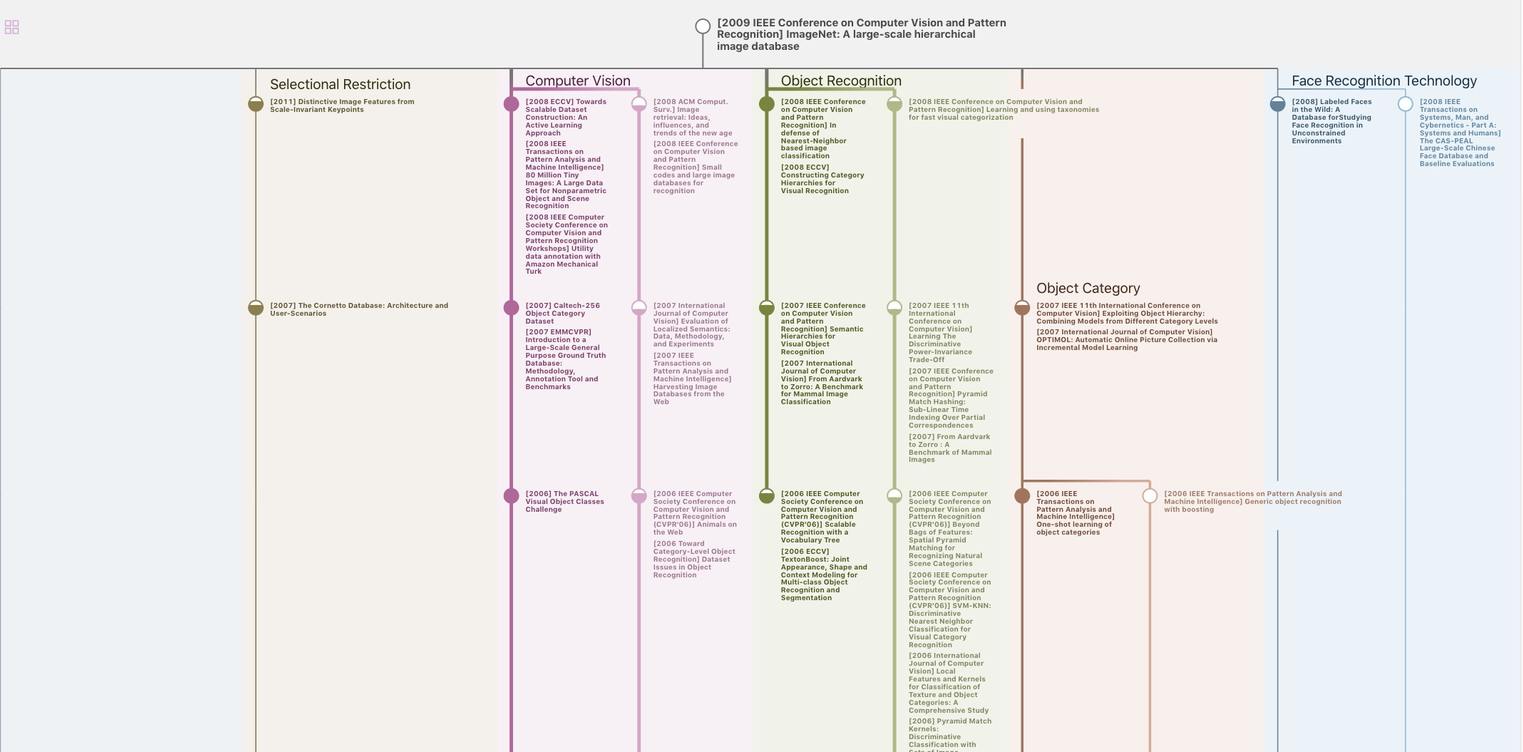
生成溯源树,研究论文发展脉络
Chat Paper
正在生成论文摘要