An Optimized Training Dynamic for Data Streams.
SSCI(2021)
Abstract
There are severe computational restrictions that are seen in Online Learning events. Nowadays, many researchers have engaged in this learning mostly due to the significance of real-world applications that deal with context changes over time on data streams. The adaptation of the current model is a strategy to deal with concept drifts in data streams, so at every concept drift, the old model is replaced by a new one. In this article, an optimized training dynamic that can choose if it is better to use or ignore detectors' Warning signals is presented to ensure a minimum amount of training or the traditional Warning instances to improve the manner of change's adaption. The strategy admits a fair evaluation connecting improvements in Prequential accuracy and concept drift signals, in addition, increases the concept drift detection methods' performance rate. The proposal algorithm's results indicate an improvement contribution to the Prequential accuracy. As well, this paper allows us to notice a better performance with Warning signals application if compared to not Warning signals application, showing their critical role to these contexts. Here, it could be seen the evaluation with DDM, FHDDM, and RDDM methods, the evaluations frequently have statistical superiorities to the optimized dynamic and its choices power if compared to the traditional approach that only contemplates the Warning signals. Although, in some cases, RDDM did not have the same behavior.
MoreTranslated text
Key words
Prequential Evaluation,Concept Drift,Data Stream,Online Learning,Warning
AI Read Science
Must-Reading Tree
Example
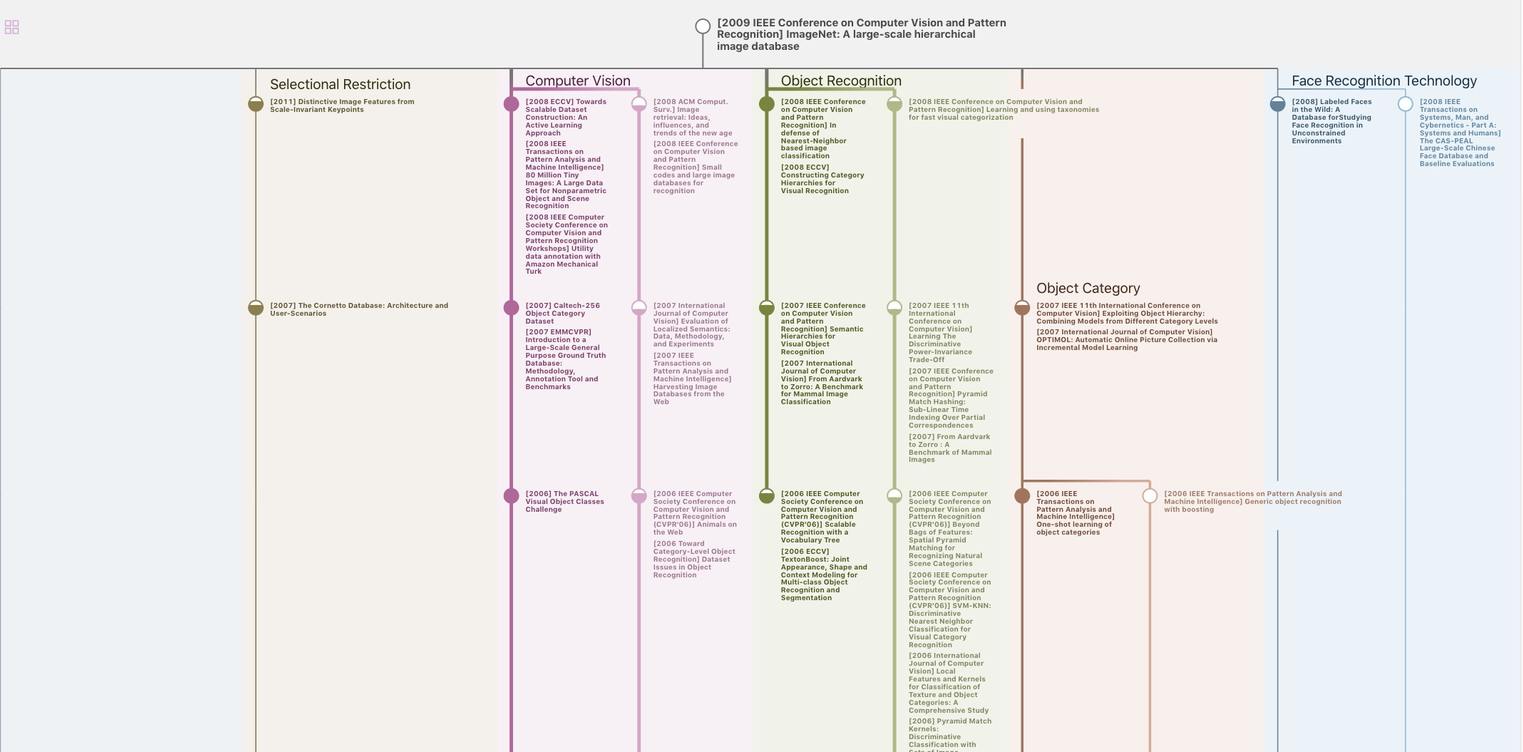
Generate MRT to find the research sequence of this paper
Chat Paper
Summary is being generated by the instructions you defined