Neural Metric Learning using Contrastive Anchors to Enforce Feature Separability.
SSCI(2021)
摘要
Deep metric learning is a paradigm to organize feature space such that dataset classes have small intra-class distance and large inter-class distance. While this domain has produced state-of-the-art results for challenging problems like facial recognition, one major limitation is the complexity of training these techniques. Methods like pairwise and triplet learning require heavy micromanagement with respect to intelligent sampling. Furthermore, methods like center loss and SphereFace require precise parameter selection to consistently ensure algorithm convergence. Herein, we propose Contrastive Anchor Network (CAN), a deep neural network that uses stationary anchors to enforce feature separability. CAN does not require intelligent sampling, can be trained with minimum parameter tuning, and uses a generalization of contrastive learning as its objective function. Experiments provide insight to CAN through qualitative feature embeddings and quantitative performance on popular benchmark datasets.
更多查看译文
关键词
Deep learning,metric learning,artificial intelligence,similarity,dissimilarity,feature learning
AI 理解论文
溯源树
样例
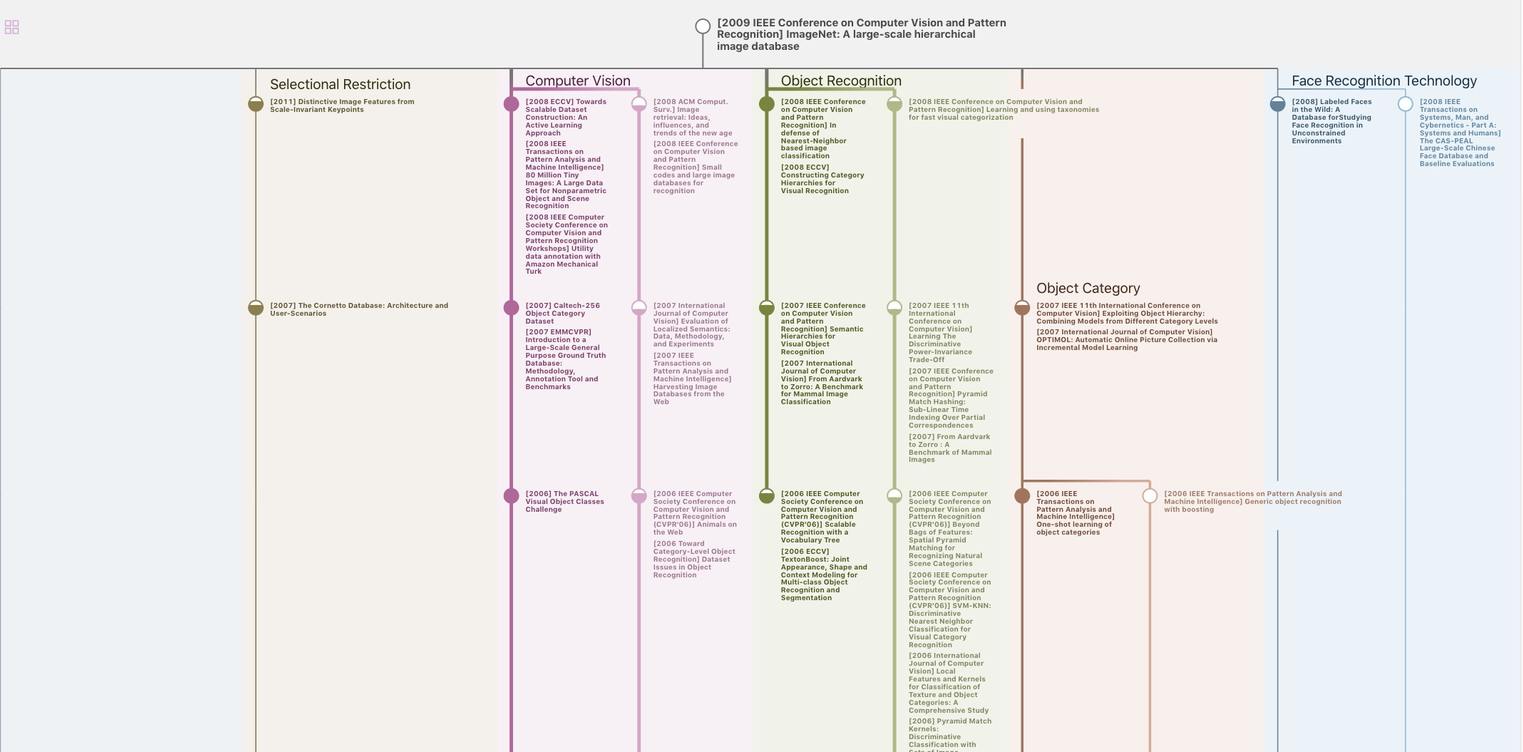
生成溯源树,研究论文发展脉络
Chat Paper
正在生成论文摘要