Unsupervised Ensemble Learning Via Network Generation.
ICME(2021)
摘要
In this work, we propose an unsupervised ensemble learning method via network generation, referred to as UELNG. Specifically, we first generate weights of clustering ensemble models by adopting HyperGAN, and obtain diverse partitions for data. With these partitions, we can easily identify high-confident pseudo-labels as supervised information to predict low-entropy labels for unlabeled augmented data, thereby enhancing the quality of pseudo-labels and clustering accuracy. We conduct experiments on multiple data sets. Experimental results indicate that our method outperforms state-of-the-art methods by 0.3%, 1.8%, 5.7%, 3.2% and 2.4% on MNIST, STL-10, CIFAR-10, Reuters and 20News, respectively, which demonstrates the effectiveness of our proposed UELNG.
更多查看译文
关键词
Unsupervised feature learning,generative adversarial networks,data augmentation,ensemble learning
AI 理解论文
溯源树
样例
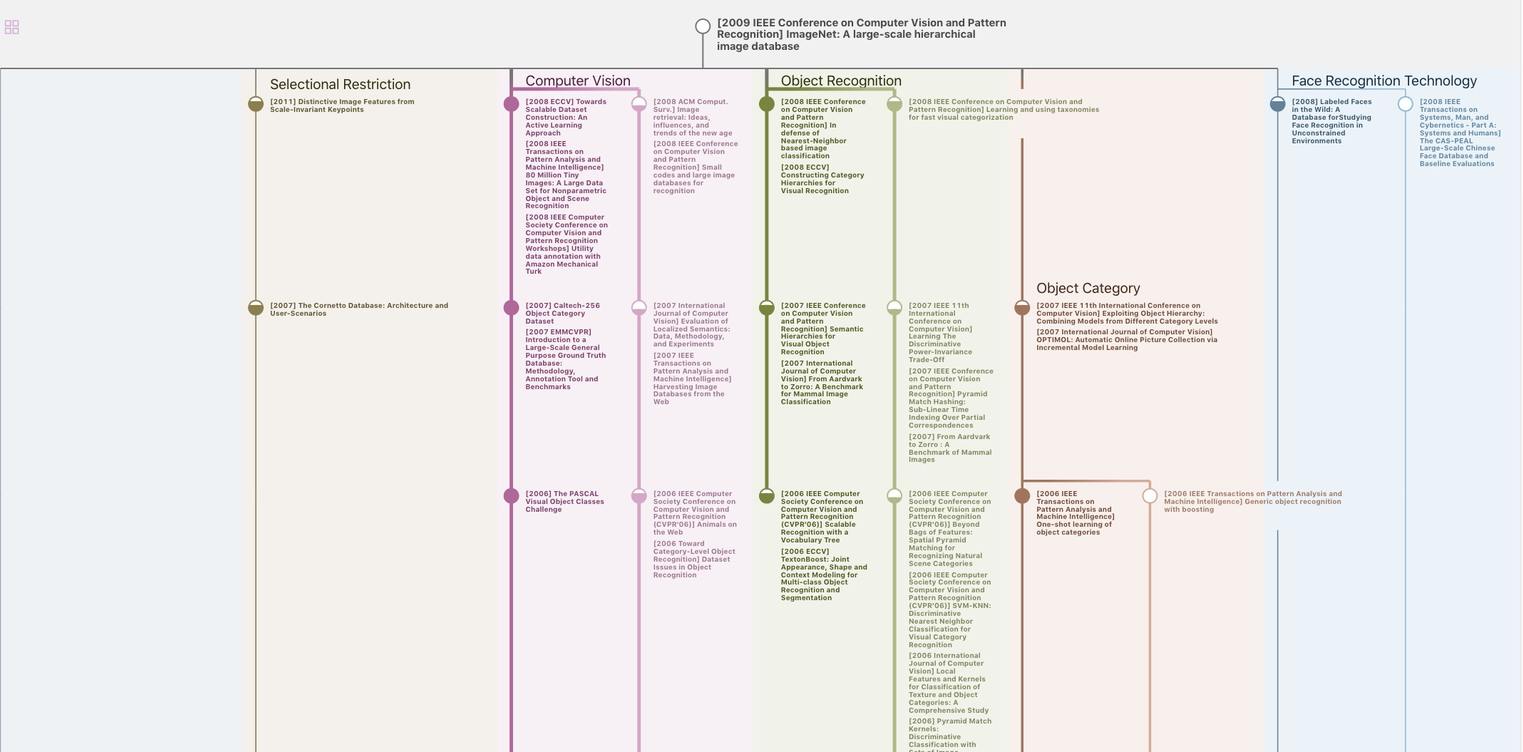
生成溯源树,研究论文发展脉络
Chat Paper
正在生成论文摘要