Statistical file-matching of non-Gaussian data: A game theoretic approach
Computational Statistics & Data Analysis(2022)
Abstract
The statistical file-matching problem is a data integration problem with structured missing data. The general form involves the analysis of multiple datasets that only have a strict subset of variables jointly observed across all datasets. Missing-data imputation is complicated by the fact that the joint distribution of the variables is nonidentifiable as there are no completely observed cases. Nonparametric imputation methods typically involve an implicit conditional independence assumption that is forced by the missing-data pattern. Parametric imputation does not require conditional independence assumptions, but can be challenging due to identifiability issues and the difficulty of parameter estimation. The identification problem can be studied using game theory, and it is possible to establish a general characterization of the minimax optimal strategy under negative log likelihood loss. For non-Gaussian models, imputation using the minimax optimal strategy can lead to different results compared to generic methods. Computationally feasible procedures for parameter estimation can be implemented using data augmentation schemes and the EM algorithm. Comparisons of the minimax optimal imputation scheme to standard algorithms on real data from flow cytometry show that minimax strategies can better preserve the joint distribution of the variables.
MoreTranslated text
Key words
Statistical file-matching,Game theory,Maximum entropy,Minimax theorem,Data integration
AI Read Science
Must-Reading Tree
Example
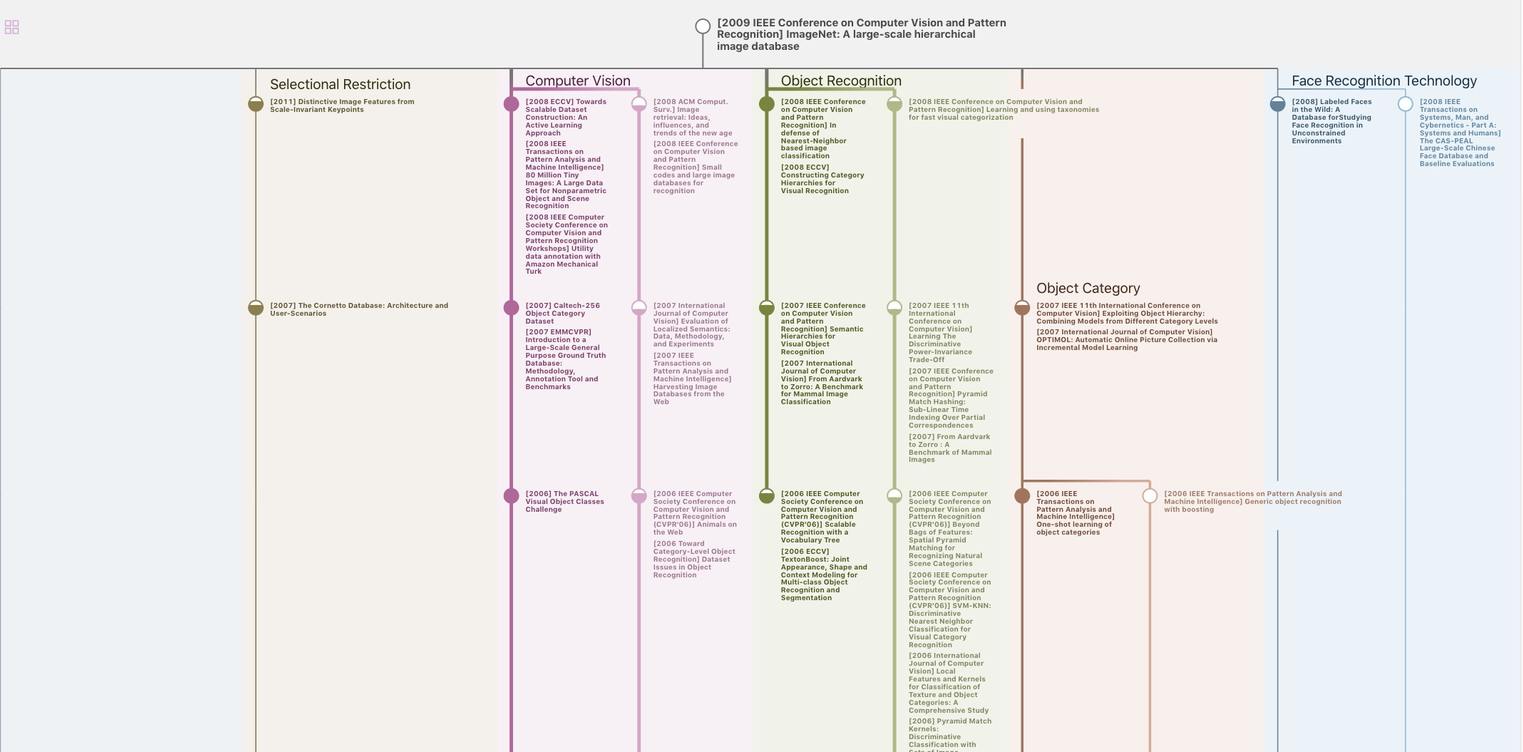
Generate MRT to find the research sequence of this paper
Chat Paper
Summary is being generated by the instructions you defined