Response time and energy consumption co-offloading with SLRTA algorithm in cloud–edge collaborative computing
Future Generation Computer Systems(2022)
摘要
Mobile edge computing (MEC) has emerged as a computing model that provides services near data generation sources. However, although MEC servers have more computing capability and resources than local, they cannot support the processing of too many user equipment (UE). In contrast, cloud servers have at least several times the computing capability and resources of MEC servers, so they can perform more tasks in parallel and with faster processing speeds. Therefore, the cloud–edge collaborative computing model combines respective advantages of MEC and cloud, and it is thus highly suitable for processing various types of tasks. How to offload tasks to the computing nodes efficiently and allocate resources optimally is an important research question. In this paper, we propose a task offloading and resource allocation algorithm with resource and reliability constraints in the cloud–edge collaborative computing environment. Three computing nodes can be used to process the task data, including UE, the MEC server and the cloud server, and the computing nodes are constrained by their computing capability, resources, and reliability. An online learning algorithm based on deep reinforcement learning (DRL) is designed for the objective optimization problem. Through the evaluation and verification of simulation experiments, the proposed algorithm is shown to be capable of effectively improving the quality of the user experience and reducing the energy consumption of the system.
更多查看译文
关键词
Deep reinforcement learning (DRL),Mobile cloud–edge collaborative,Resource allocation,Task offloading
AI 理解论文
溯源树
样例
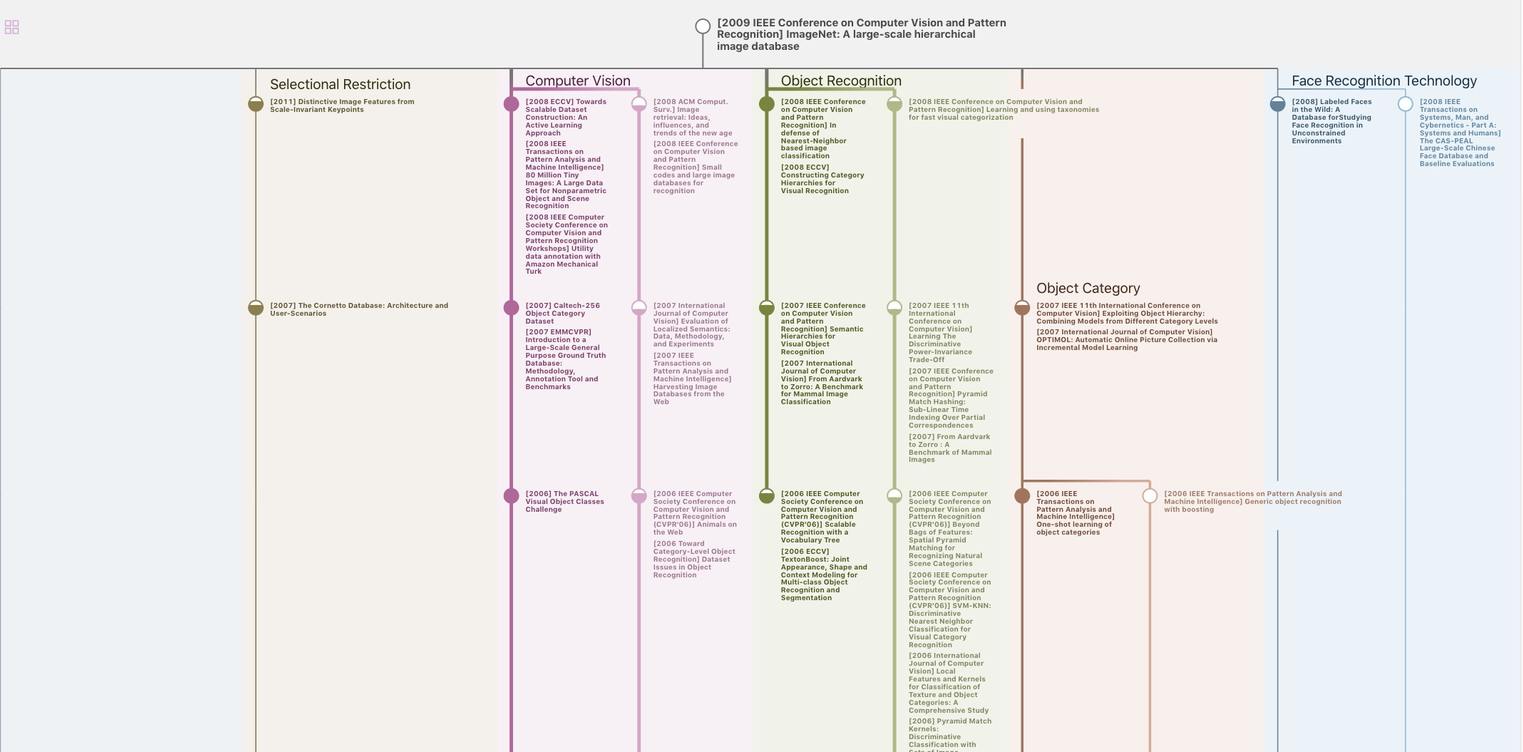
生成溯源树,研究论文发展脉络
Chat Paper
正在生成论文摘要