Person re-identification using adversarial haze attack and defense: A deep learning framework
COMPUTERS & ELECTRICAL ENGINEERING(2021)
摘要
In this paper, the adversarial haze attack problem is addressed using the dark channel prior (DCP) de-hazing method. The adversarial attack affects rank-1 accuracy, where searching a target image against each test image is a specific search problem. To resolve this kind of problem, a feature fusion model is proposed to fuse handcrafted features and a pre-trained network model to obtain robust and discriminative features. The proposed model learns global features using transfer learning architecture whereas local features are obtained using the conventional method. Three pre-trained CNN models (AlexNet, ResNet, and Inception-v3) are used for feature extraction via transfer learning. The experiments are performed on publicly available datasets, achieving 68.6% accuracy in rank-1 with VIPER dataset and 79.6% accuracy with CHUK03 dataset. The proposed model enhances rank-1 accuracy of person re-identification when comparing with other state-ofthe-art methods.
更多查看译文
关键词
Person re-identification, Adversarial haze attack, Dark channel prior (DCP) algorithm, Feature fusion, Deep learning, Transfer learning, Handcrafted model
AI 理解论文
溯源树
样例
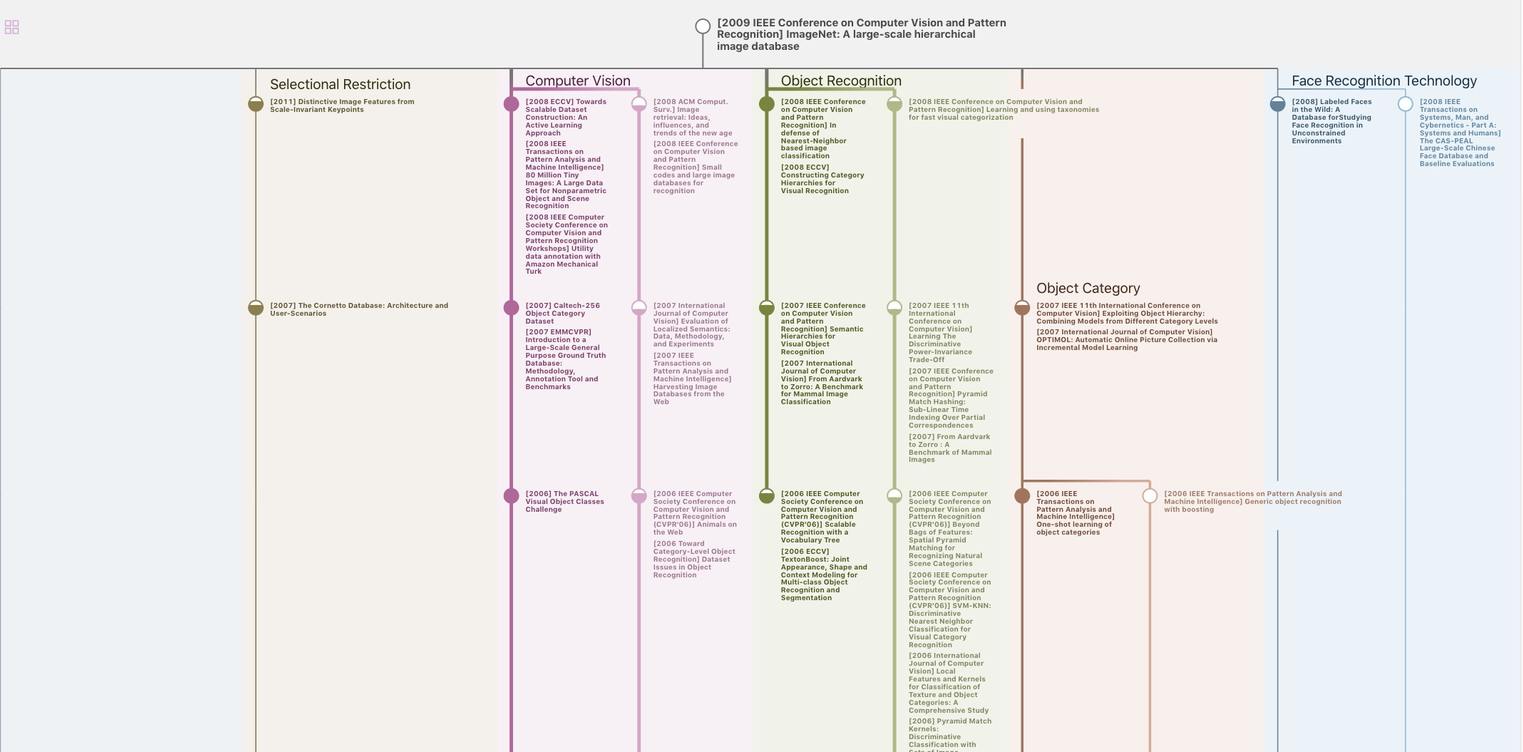
生成溯源树,研究论文发展脉络
Chat Paper
正在生成论文摘要