Evaluation of dimensionality reduction methods for individual tree crown delineation using instance segmentation network and UAV multispectral imagery in urban forest
COMPUTERS AND ELECTRONICS IN AGRICULTURE(2021)
摘要
The diversity of features in urban forest poses challenges to the task of delineation individual tree crowns. Multispectral image helps to improve the accuracy of individual tree crown delineation. It is necessary to reduce the dimensionality of multispectral images, but which dimensionality reduction method is suitable for the individual tree crown task based on deep learning still needs further research. In this study, four dimensionality reduction methods (principal component analysis, independent component analysis, optimum index factor, standard false color composite) were used to reduce the dimensionality of multispectral images. The images after dimensionality reduction were made as dataset for network training. Two instance segmentation networks (BlendMask, Mask R-CNN) were used to delineate the ginkgo tree crowns of UAV multispectral images after dimensionality reduction in urban. The effect of dimensionality reduction methods on two networks was evaluated in detail. The result of experiments presented that the standard false color composite method obtained the best value with 60.0% in average precision, 95.3% in average precision (Intersection over Union = 0.5) and 70.8% in average recall. The feature extraction methods (principal component analysis, independent component analysis) showed a good performance in the simple plot, but failed in the dense plot. The band selection methods (optimum index factor, standard false color composite) were more stable than the feature extraction methods in both plots. This article provides an important reference for related researchers on the choice of dimensionality reduction methods.
更多查看译文
关键词
Dimensionality reduction, Individual tree crown delineation, Instance segmentation, Multispectral imagery
AI 理解论文
溯源树
样例
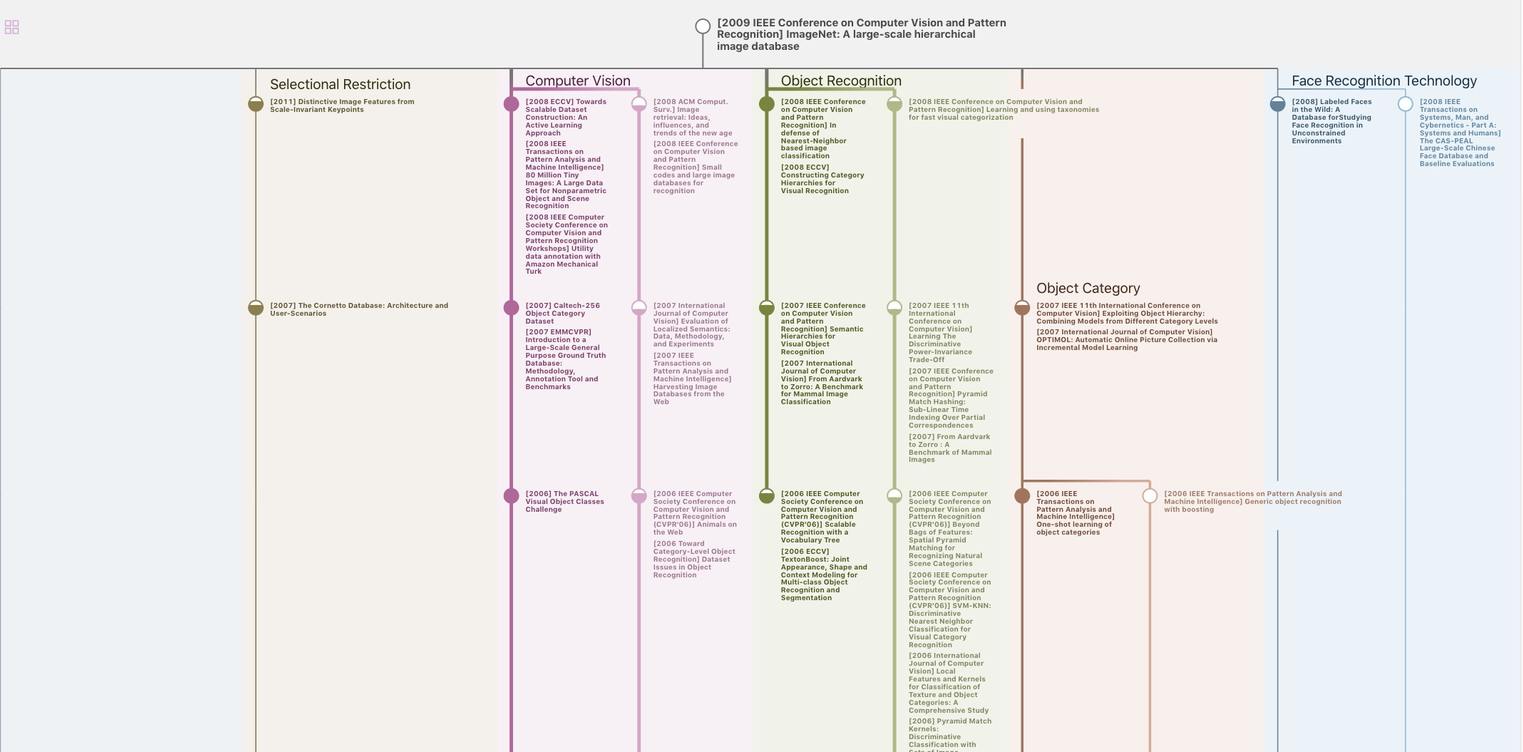
生成溯源树,研究论文发展脉络
Chat Paper
正在生成论文摘要