An adaptive sliding window for anomaly detection of time series in wireless sensor networks
WIRELESS NETWORKS(2022)
摘要
Recent years, anomaly detection for time series in wireless sensor networks has attracted much research attention. Existing anomaly detection methods based on pattern representation ignore the trend features of an original sequence. This leads to low detection accuracy. This paper aims to solve the above problem with an adaptive sliding window for anomaly detection. The advantage of this method is that the size of the sliding window is changed according to the trend of the time series. Thus, the extracted trend feature points can accurately represent an original time series. The pattern representation is vertically divided into several subspaces with equal-size in the time domain. We employ four features which are extracted from each subspace to obtain a pattern matrix. The similarity among different patterns is measured according to the similarity matrix which is formed by pattern matrices. Finally, anomaly scores obtained from similarity matrices are compared with a threshold to detect anomaly. The proposed method is verified on two synthetic datasets and a real-world dataset. Experimental results showed that our method could improve the accuracy and reduce the specificity compared to three classic models.
更多查看译文
关键词
Wireless sensor networks, Anomaly detection, Time series, Adaptive sliding window, Pattern representation
AI 理解论文
溯源树
样例
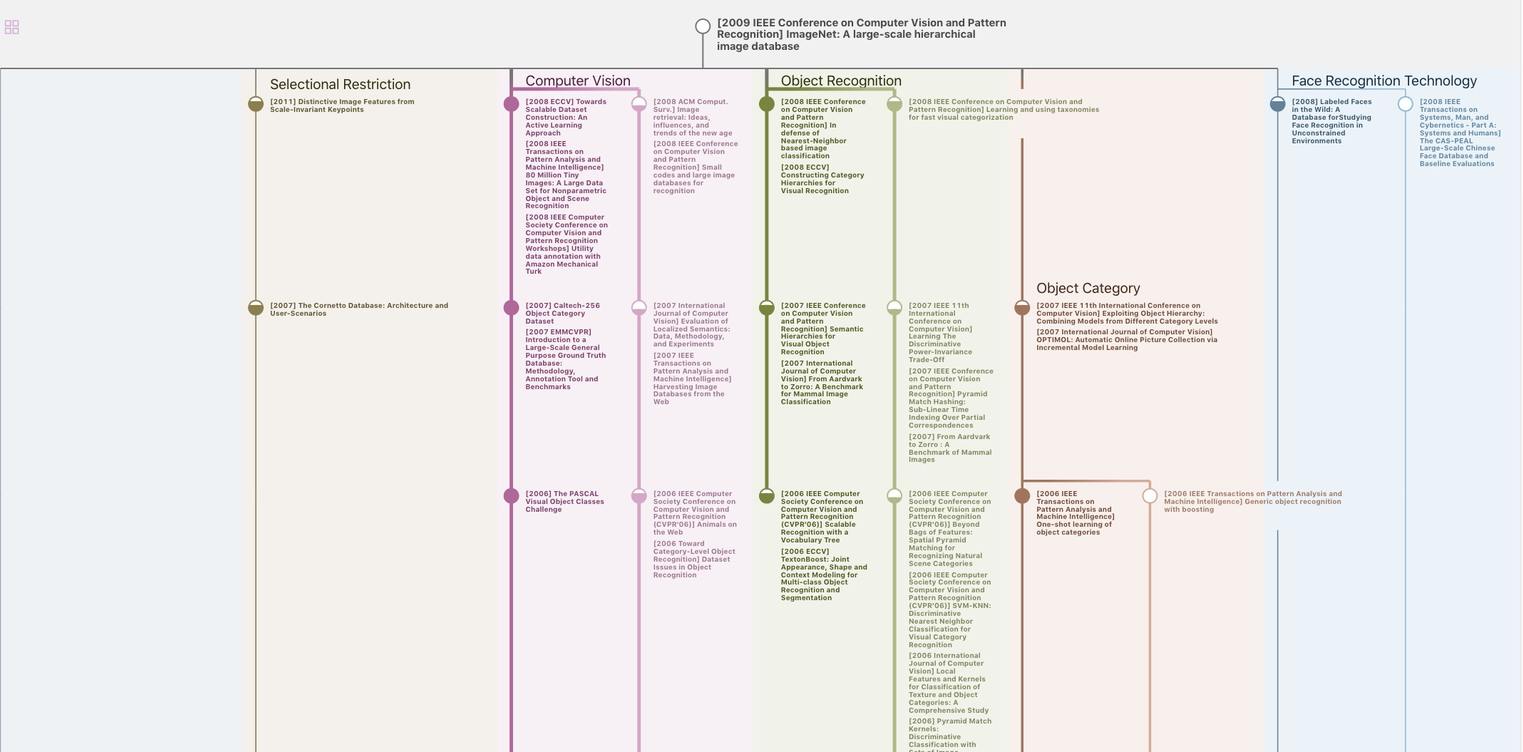
生成溯源树,研究论文发展脉络
Chat Paper
正在生成论文摘要