Forest pest identification based on a new dataset and convolutional neural network model with enhancement strategy
COMPUTERS AND ELECTRONICS IN AGRICULTURE(2022)
Abstract
Due to the lack of samples, deep learning in forest pest identification is severely limited, and classification accuracy and generalization ability are insufficient. To address this issue, we have constructed a new dataset of forest pests containing 67,953 images, enhanced the dataset by Graph-based Visual Saliency, and combined transfer learning and fine-tune to build a twice transfer strategy in the convolutional neural networks (CNNs) for pest recognition. Based on the new dataset and developed model, a new platform for forest pest identification was finally built. Compared with prevalent models including Inception-V3, MobileNet-V2, ResNet-50-V2, InceptionResNet-V2, and Xception, our method improves the accuracy and generalization ability of classification by 6.2% and 7.0%, respectively. Meanwhile, class activation maps show that the model's focus on the target has also been increased by 9.0%. In general, the new proposed dataset and training strategy can greatly improve classification performance of CNNs, which may be helpful to the effective control on forest pests.
MoreTranslated text
Key words
New dataset, Forest pests, Dataset enhancement, Twice transfer strategy, Identification platform
AI Read Science
Must-Reading Tree
Example
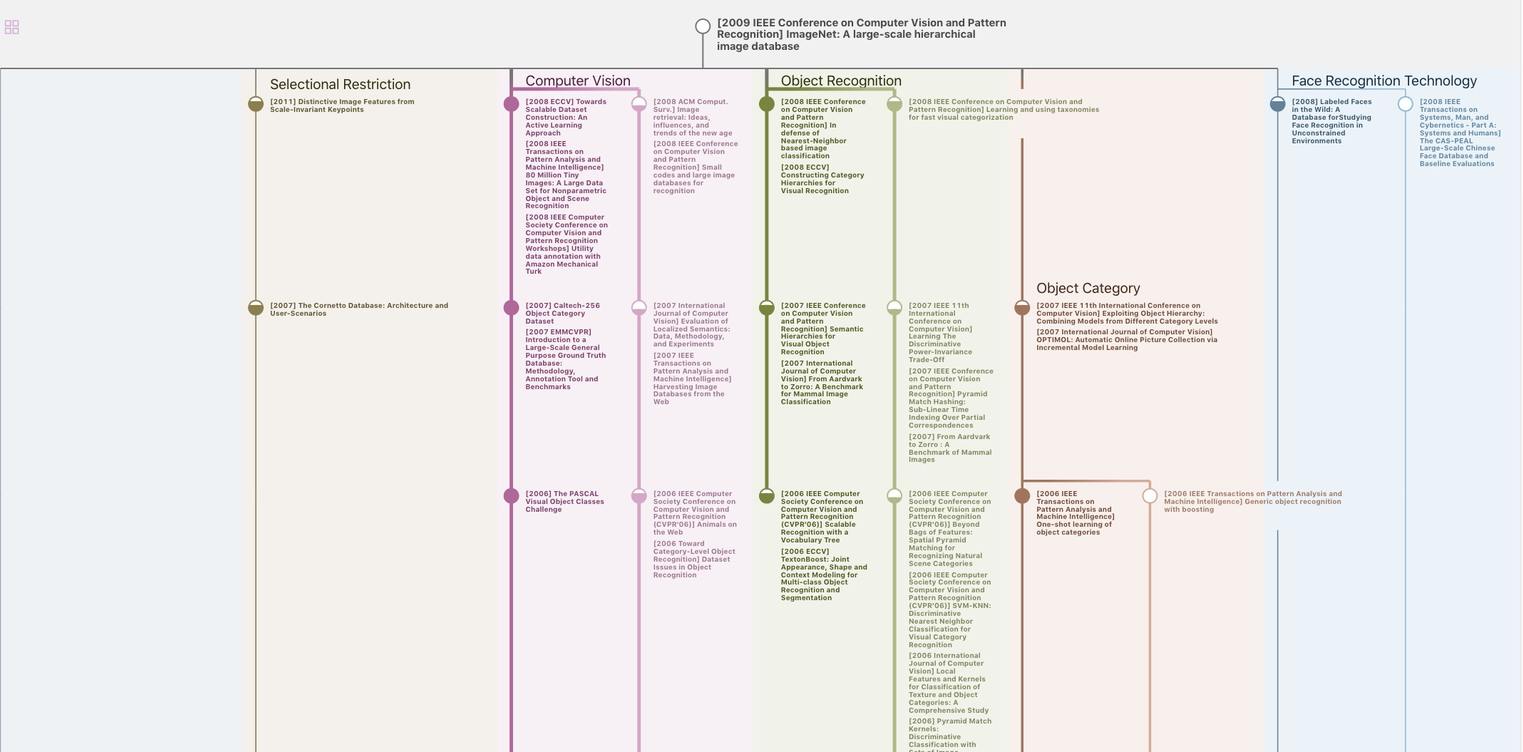
Generate MRT to find the research sequence of this paper
Chat Paper
Summary is being generated by the instructions you defined