A dynamic routing CapsNet based on increment prototype clustering for overcoming catastrophic forgetting
IET COMPUTER VISION(2022)
Abstract
In continual learning, previously learnt knowledge tends to be overlapped by the subsequent training tasks. This bottleneck, known as catastrophic forgetting, has recently been relieved between vision tasks involving pixel shuffles etc. Nevertheless, the challenge lies in the continuous classification of the sequential sets discriminated by global transformations, such as excessively spatial rotations. Aim at this, a novel strategy of dynamic memory routing is proposed to dominate the forward paths of capsule network (CapsNet) according to the current input sets. To recall previous knowledge, a binary routing table is maintained among these sequential tasks. Then, an increment procedure of competitive prototype clustering is integrated to update the routing of the current task. Moreover, a sparsity measurement is employed to decouple the salient routing among the different learnt tasks. The experimental results demonstrate the superiority of the proposed memory network over the state-of-the-art approaches by the recalling evaluations on extended sets of Cifar-100, CelebA and Tiny ImageNet etc.
MoreTranslated text
Key words
capsule network, catastrophic forgetting, continual learning, dynamic routing, prototype clustering
AI Read Science
Must-Reading Tree
Example
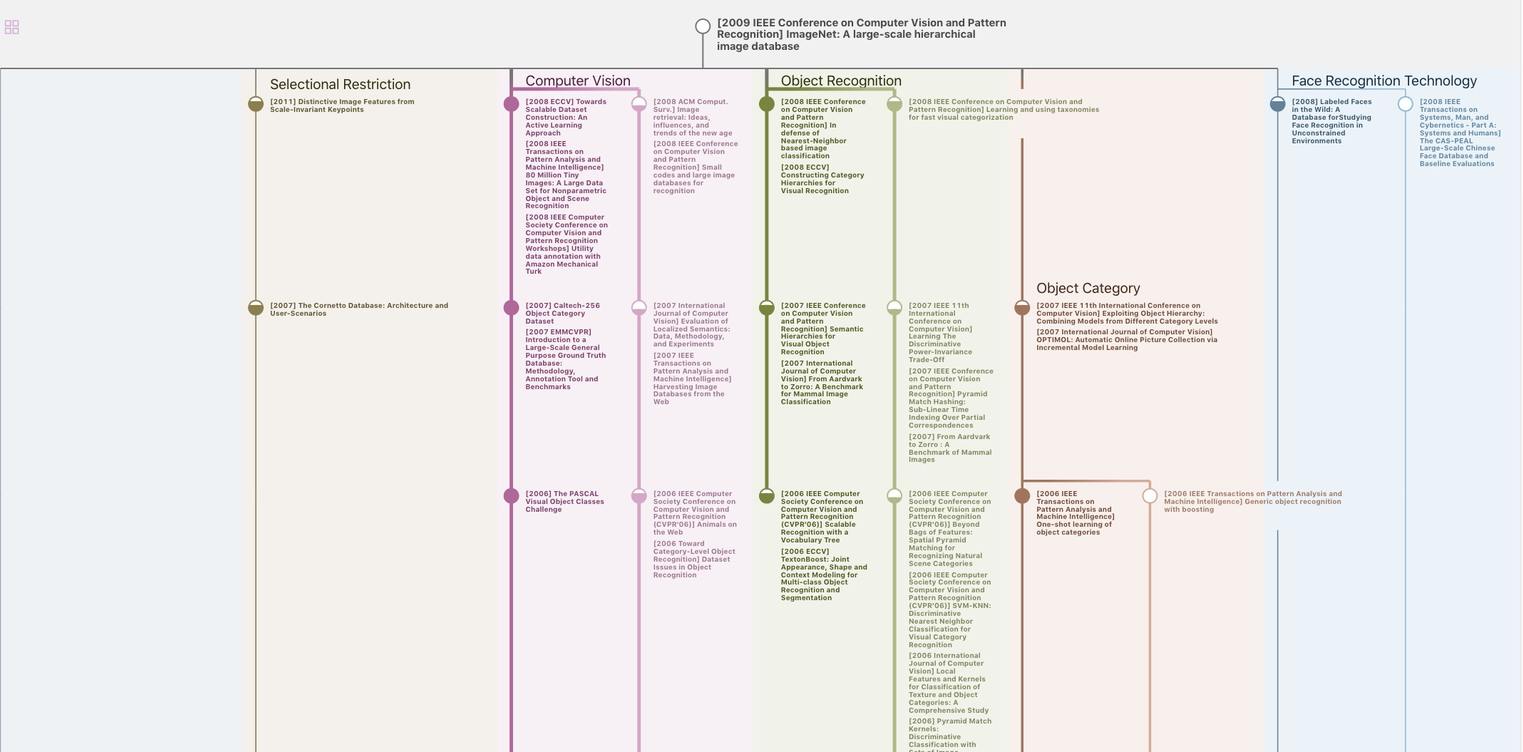
Generate MRT to find the research sequence of this paper
Chat Paper
Summary is being generated by the instructions you defined