An efficient recommender system algorithm using trust data
The Journal of Supercomputing(2021)
Abstract
Smart services are a concept that provides services to the citizens in an efficient manner. The online shopping and recommender system can play an important role for smart cities in providing relevant item recommendations to the users. One of the famous Recommendation System strategies is known as Collaborative Filtering and provides popular suggestions to the users. The recommendation is generated by identifying a set of similar users from a user-item rating matrix using a similarity measure. The problem with the majority of the recommender systems is whether the generated recommendations are good enough because users usually find recommendations from their circle more appealing. It is important to use only those similar users that have some kind of trust among them. The accuracy of the recommendations also gets affected due to the sparsity of the user-item matrix. To handle these problems, a trust-based technique TrustASVD++ is proposed, which combines a user’s trust data in the Matrix Factorization context. The proposed method combines trust values with user ratings for improved recommendations using Pearson Correlation Coefficient (PCC). PCC is compared with other state-of-the-art similarity measures, and the results obtained show that PCC outperforms all the other relevant measures. To assess the efficiency of the offered strategy, testing on numerous datasets has been carried out including Epinions, FilmTrust, and Ciao. The results illustrate the considerable improvement of the proposed method over numerous contemporary techniques.
MoreTranslated text
Key words
Smart services,Collaborative filtering,Recommendation system,Trust data,Matrix factorization
AI Read Science
Must-Reading Tree
Example
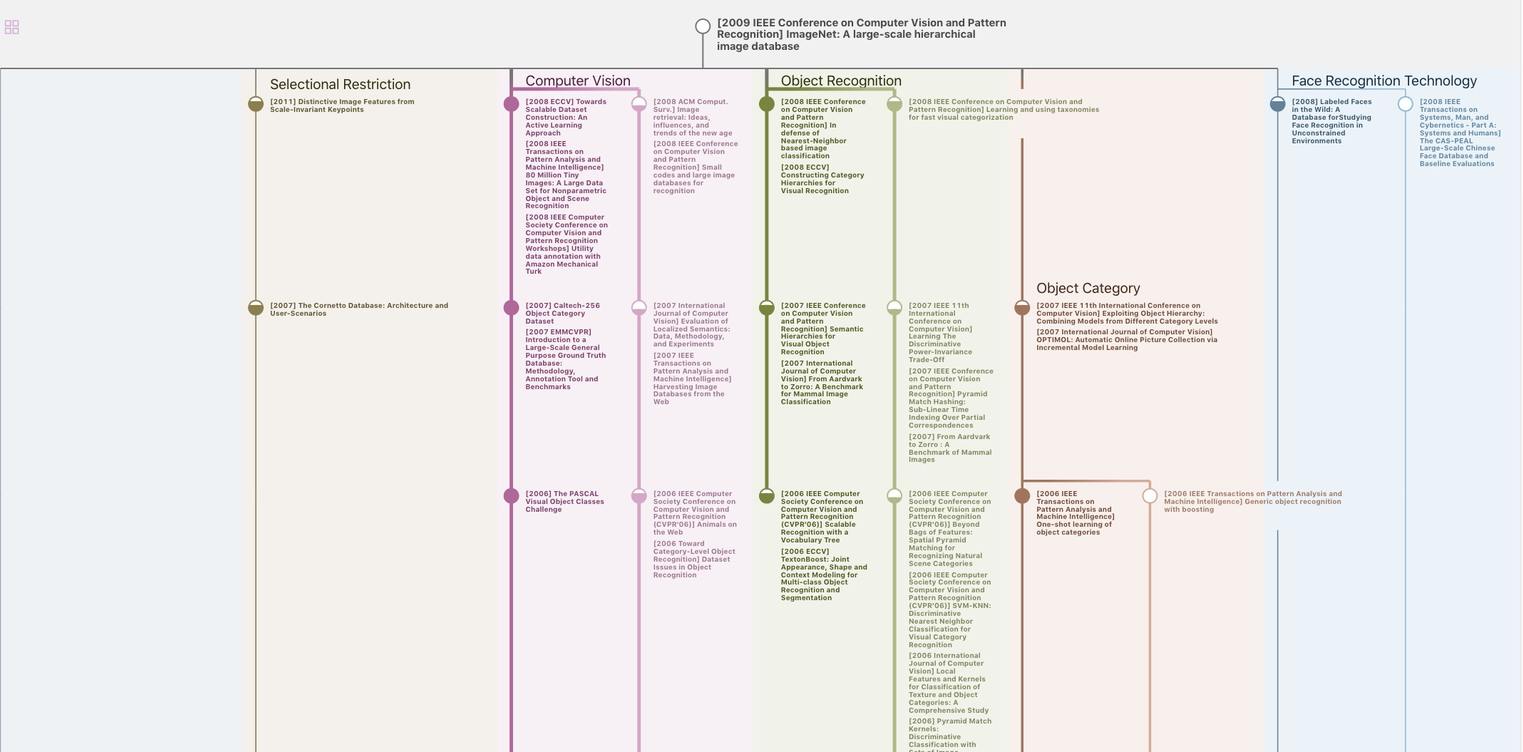
Generate MRT to find the research sequence of this paper
Chat Paper
Summary is being generated by the instructions you defined