Planckian Jitter: countering the color-crippling effects of color jitter on self-supervised training
arxiv(2023)
摘要
Several recent works on self-supervised learning are trained by mapping different augmentations of the same image to the same feature representation. The data augmentations used are of crucial importance to the quality of learned feature representations. In this paper, we analyze how the color jitter traditionally used in data augmentation negatively impacts the quality of the color features in learned feature representations. To address this problem, we propose a more realistic, physics-based color data augmentation - which we call Planckian Jitter - that creates realistic variations in chromaticity and produces a model robust to illumination changes that can be commonly observed in real life, while maintaining the ability to discriminate image content based on color information. Experiments confirm that such a representation is complementary to the representations learned with the currently-used color jitter augmentation and that a simple concatenation leads to significant performance gains on a wide range of downstream datasets. In addition, we present a color sensitivity analysis that documents the impact of different training methods on model neurons and shows that the performance of the learned features is robust with respect to illuminant variations.
更多查看译文
关键词
Contrastive Learning,Self-Supervised Learning,Color Features,Illuminant Invariance
AI 理解论文
溯源树
样例
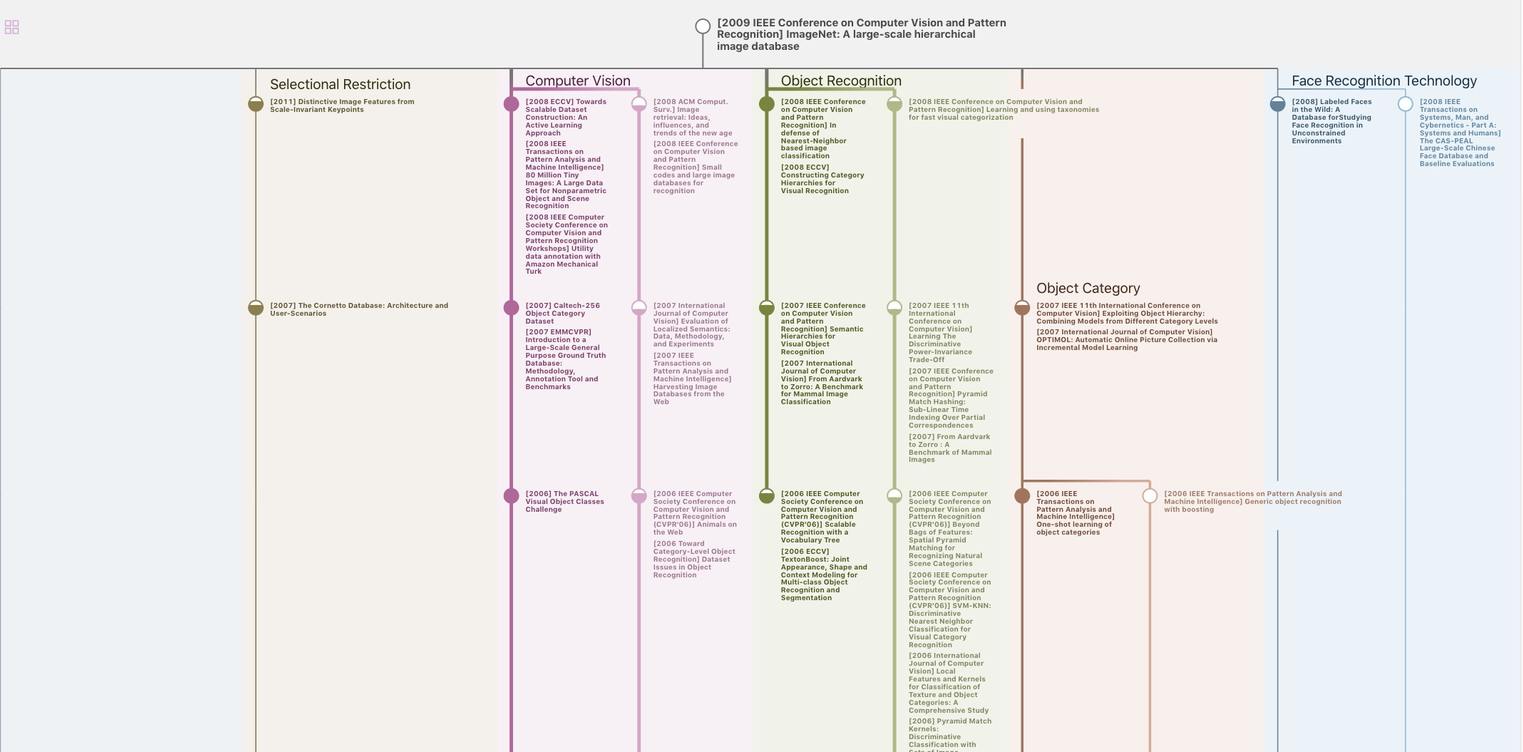
生成溯源树,研究论文发展脉络
Chat Paper
正在生成论文摘要