Don't Lie to Me! Robust and Efficient Explainability with Verified Perturbation Analysis
CVPR(2023)
Abstract
A plethora of attribution methods have recently been developed to explain deep neural networks. These methods use different classes of perturbations (e.g, occlusion, blurring, masking, etc) to estimate the importance of individual image pixels to drive a model's decision. Nevertheless, the space of possible perturbations is vast and current attribution methods typically require significant computation time to accurately sample the space in order to achieve high-quality explanations. In this work, we introduce EVA (Explaining using Verified Perturbation Analysis) - the first explainability method which comes with guarantees that an entire set of possible perturbations has been exhaustively searched. We leverage recent progress in verified perturbation analysis methods to directly propagate bounds through a neural network to exhaustively probe a - potentially infinite-size - set of perturbations in a single forward pass. Our approach takes advantage of the beneficial properties of verified perturbation analysis, i.e., time efficiency and guaranteed complete - sampling agnostic coverage of the perturbation space - to identify image pixels that drive a model's decision. We evaluate EVA systematically and demonstrate state-of-the-art results on multiple benchmarks. Our code is freely available: github.com/deel-ai/formal-explainability
MoreTranslated text
Key words
Explainable computer vision
AI Read Science
Must-Reading Tree
Example
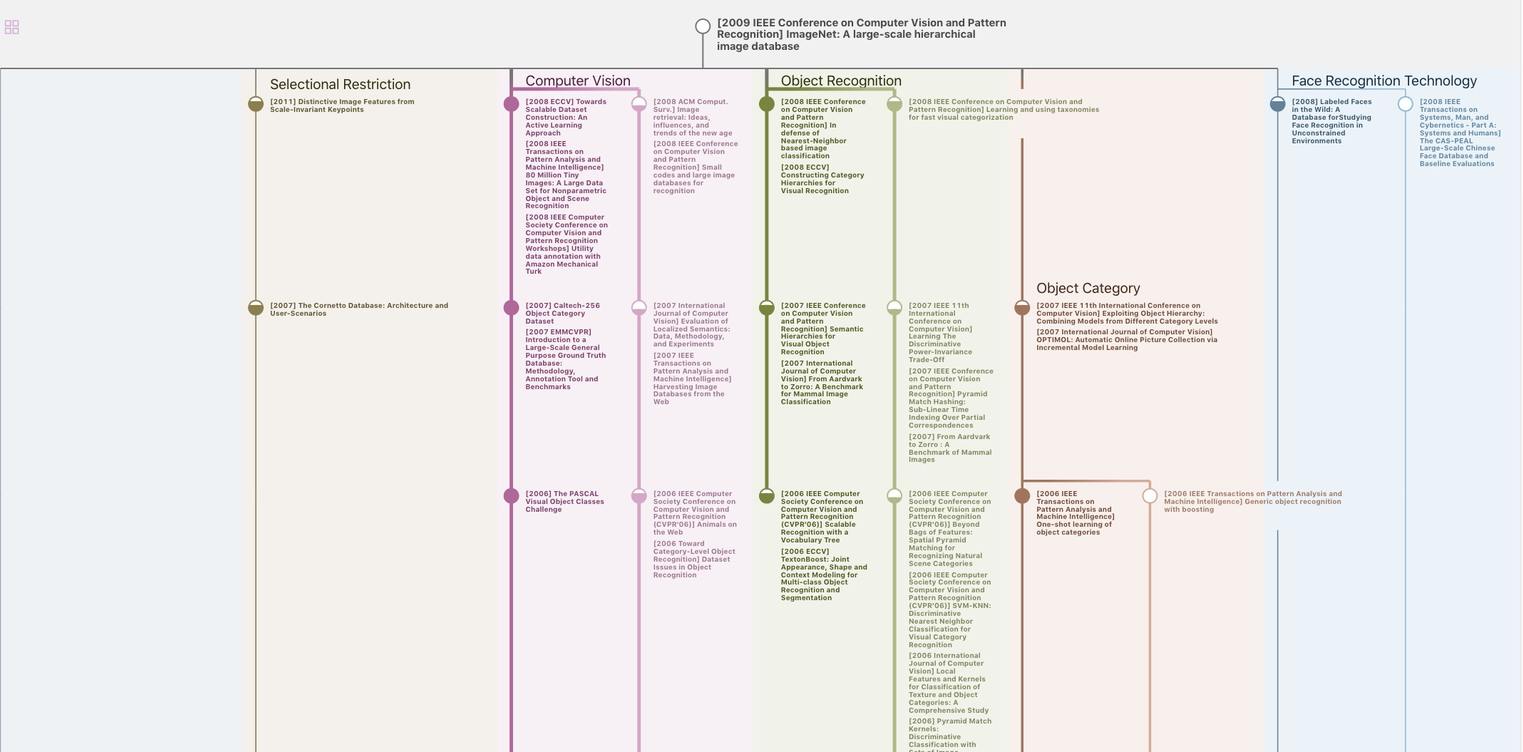
Generate MRT to find the research sequence of this paper
Chat Paper
Summary is being generated by the instructions you defined