A Carbon Nanotube Sensor Array for the Label-Free Discrimination of Live and Dead Cells with Machine Learning
ANALYTICAL CHEMISTRY(2022)
摘要
Developing robust cell recognition strategies is important in biochemical research, but the lack of well-defined target molecules creates a bottleneck in some applications. In this paper, a carbon nanotube sensor array was constructed for the label-free discrimination of live and dead mammalian cells. Three types of carbon nanotube field-effect transistors were fabricated, and different features were extracted from the transfer characteristic curves for model training with linear discriminant analysis (LDA) and support-vector machines (SVM). Live and dead cells were accurately classified in more than 90% of samples in each sensor group using LDA as the algorithm. The recursive feature elimination with cross-validation (RFECV) method was applied to handle the overfitting and optimize the model, and cells could be successfully classified with as few as four features and a higher validation accuracy (up to 97.9%) after model optimization. The RFECV method also revealed the crucial features in the classification, indicating the participation of different sensing mechanisms in the classification. Finally, the optimized LDA model was applied for the prediction of unknown samples with an accuracy of 87.5-93.8%, indicating that live and dead cell samples could be well-recognized with the constructed model.
更多查看译文
关键词
carbon nanotube sensor array,dead cells,label-free
AI 理解论文
溯源树
样例
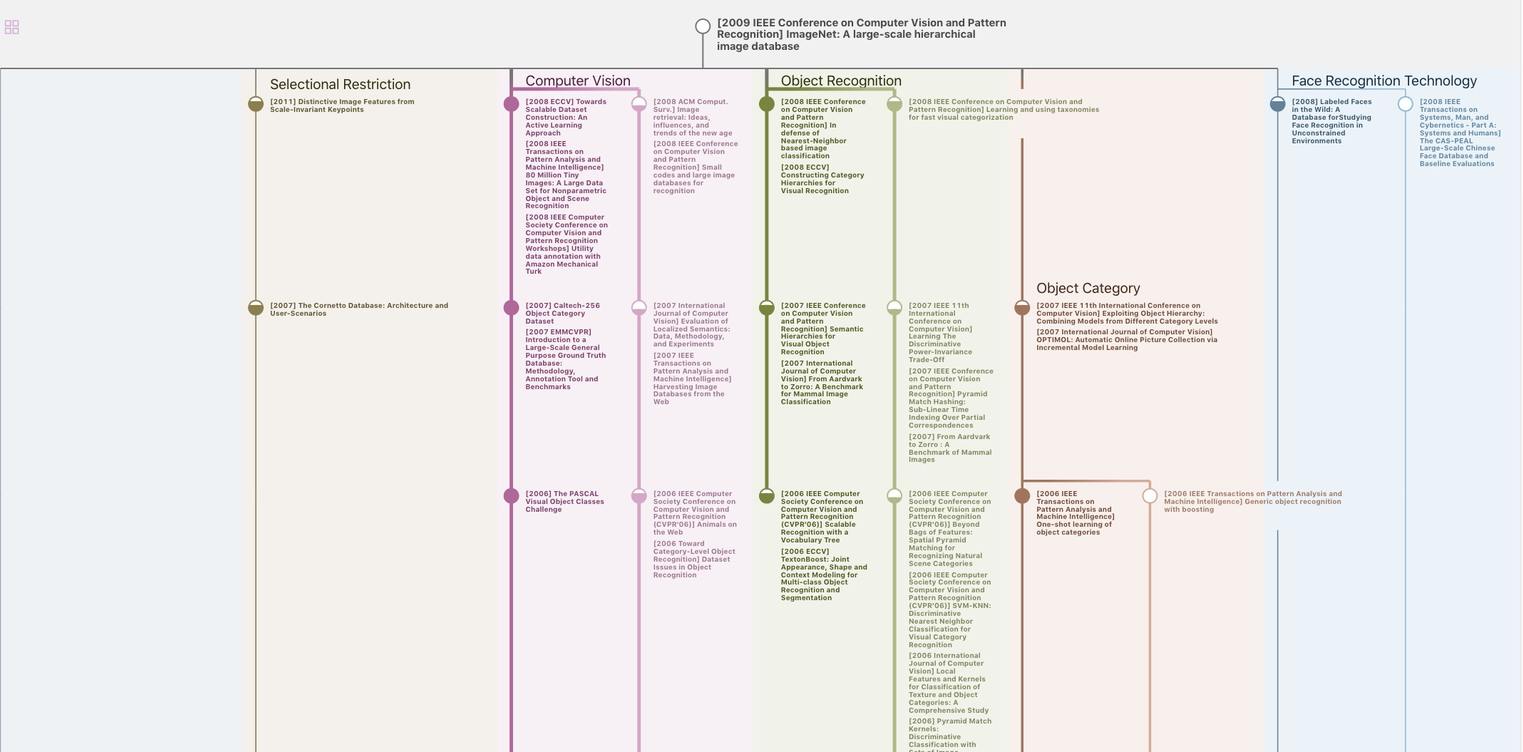
生成溯源树,研究论文发展脉络
Chat Paper
正在生成论文摘要