A Control Method with Reinforcement Learning for Urban Un-Signalized Intersection in Hybrid Traffic Environment
SENSORS(2022)
摘要
To control autonomous vehicles (AVs) in urban unsignalized intersections is a challenging problem, especially in a hybrid traffic environment where self-driving vehicles coexist with human driving vehicles. In this study, a coordinated control method with proximal policy optimization (PPO) in Vehicle-Road-Cloud Integration System (VRCIS) is proposed, where this control problem is formulated as a reinforcement learning (RL) problem. In this system, vehicles and everything (V2X) was used to keep communication between vehicles, and vehicle wireless technology can detect vehicles that use vehicles and infrastructure (V2I) wireless communication, thereby achieving a cost-efficient method. Then, the connected and autonomous vehicle (CAV) defined in the VRCIS learned a policy to adapt to human driving vehicles (HDVs) across the intersection safely by reinforcement learning (RL). We have developed a valid, scalable RL framework, which can communicate topologies that may be dynamic traffic. Then, state, action and reward of RL are designed according to urban unsignalized intersection problem. Finally, how to deploy within the RL framework was described, and several experiments with this framework were undertaken to verify the effectiveness of the proposed method.
更多查看译文
关键词
reinforcement learning, connected and autonomous vehicles, urban unsignalized intersection
AI 理解论文
溯源树
样例
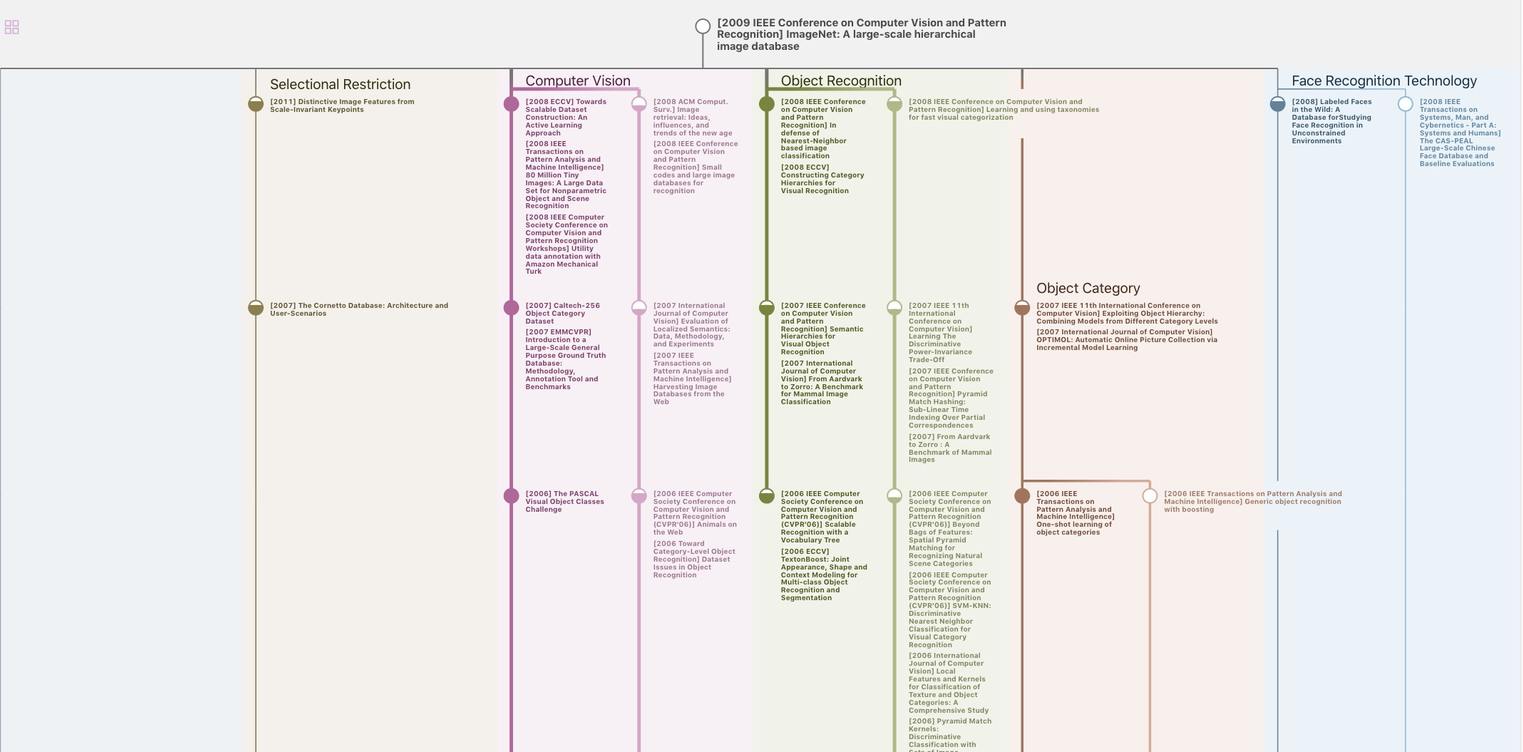
生成溯源树,研究论文发展脉络
Chat Paper
正在生成论文摘要