Predicting Glioblastoma Cellular Motility from In Vivo MRI with a Radiomics Based Regression Model
CANCERS(2022)
Abstract
Simple Summary A diagnosis of glioblastoma carries a uniformly dismal prognosis. Contributing to this is the near certain chance of aggressive tumor spread and recurrence following treatment. Tumor cell motility may provide one way to characterize the tendencies of glioblastomas to spread and recur. We sought to develop a non-invasive technique for assessing tumor cell motility using quantitative features derived from in vivo preoperative magnetic resonance imaging. Our regression model accurately predicted tumor cell motility in a cohort of participants with preoperative imaging who also had mean cellular motility calculated for their resected tumor cells from time-lapse videos. This work establishes the feasibility of non-invasively characterizing the kinetic properties of tumors and could be used to select patients for motility-targeting precision therapies. Characterizing the motile properties of glioblastoma tumor cells could provide a useful way to predict the spread of tumors and to tailor the therapeutic approach. Radiomics has emerged as a diagnostic tool in the classification of tumor grade, stage, and prognosis. The purpose of this work is to examine the potential of radiomics to predict the motility of glioblastoma cells. Tissue specimens were obtained from 31 patients undergoing surgical resection of glioblastoma. Mean tumor cell motility was calculated from time-lapse videos of specimen cells. Manual segmentation was used to define the border of the enhancing tumor T1-weighted MR images, and 107 radiomics features were extracted from the normalized image volumes. Model parameter coefficients were estimated using the adaptive lasso technique validated with leave-one-out cross validation (LOOCV) and permutation tests. The R-squared value for the predictive model was 0.60 with p-values for each individual parameter estimate less than 0.0001. Permutation test models trained with scrambled motility failed to produce a model that out-performed the model trained on the true data. The results of this work suggest that it is possible for a quantitative MRI feature-based regression model to non-invasively predict the cellular motility of glioblastomas.
MoreTranslated text
Key words
glioblastoma,cellular motility,MRI,radiomics
AI Read Science
Must-Reading Tree
Example
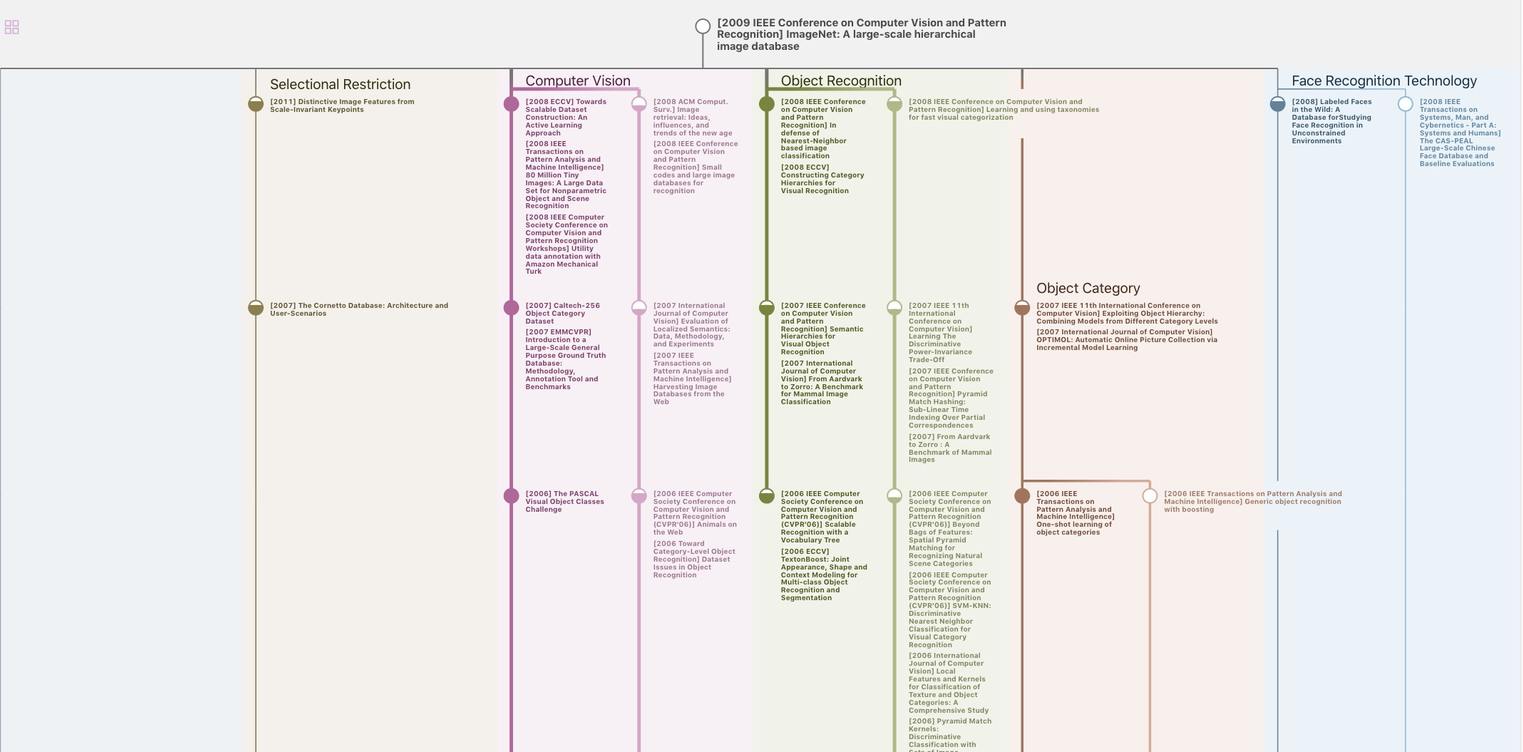
Generate MRT to find the research sequence of this paper
Chat Paper
Summary is being generated by the instructions you defined