Calomplification -- The Power of Generative Calorimeter Models
arxiv(2023)
摘要
Motivated by the high computational costs of classical simulations, machine-learned generative models can be extremely useful in particle physics and elsewhere. They become especially attractive when surrogate models can efficiently learn the underlying distribution, such that a generated sample outperforms a training sample of limited size. This kind of GANplification has been observed for simple Gaussian models. We show the same effect for a physics simulation, specifically photon showers in an electromagnetic calorimeter.
更多查看译文
关键词
Detector modelling and simulations I (interaction of radiation with matter, interaction, of photons with matter, interaction of hadrons with matter, etc),Simulation methods and programs,Analysis and statistical methods,Calorimeter methods
AI 理解论文
溯源树
样例
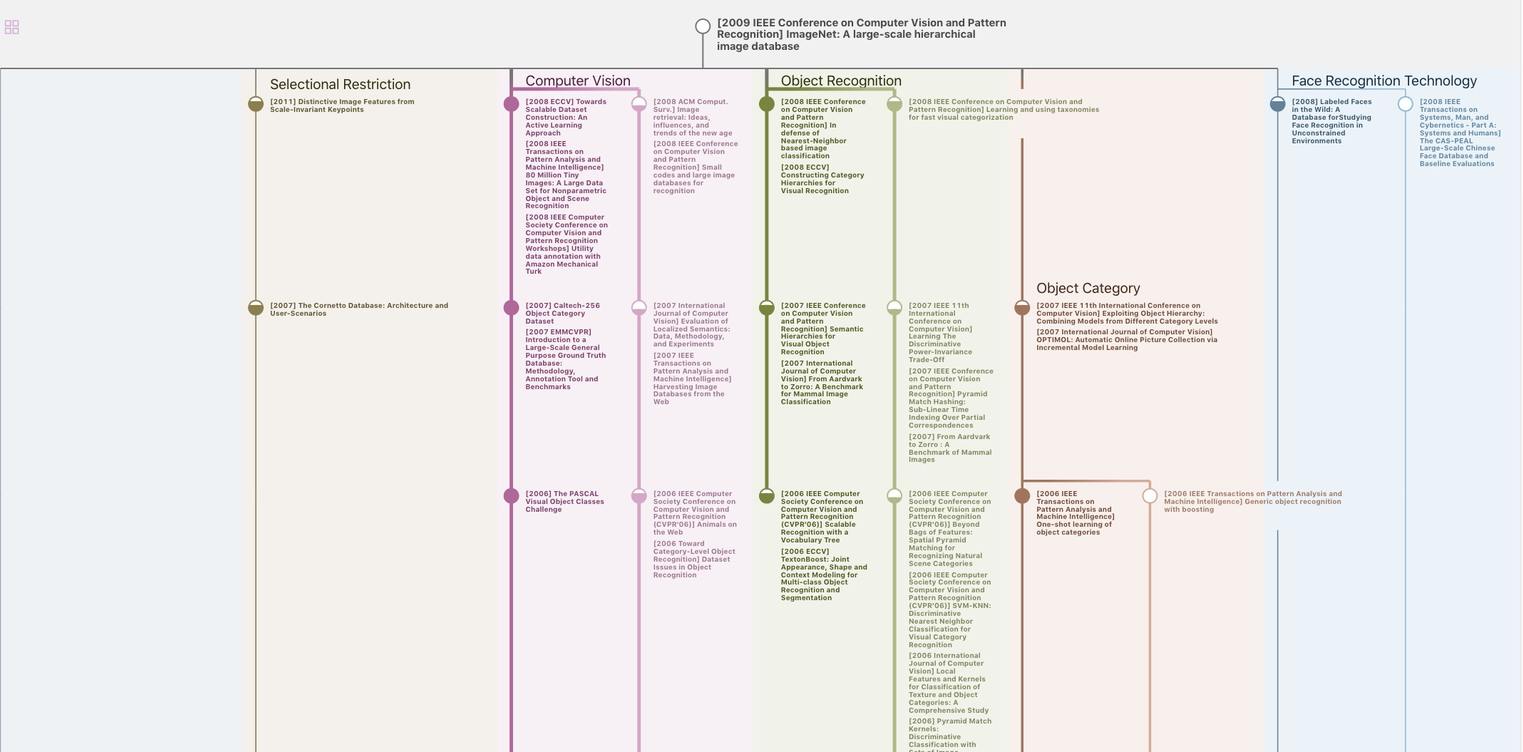
生成溯源树,研究论文发展脉络
Chat Paper
正在生成论文摘要