Learning to Mitigate AI Collusion on Economic Platforms
arxiv(2022)
摘要
Algorithmic pricing on online e-commerce platforms raises the concern of tacit collusion, where reinforcement learning algorithms learn to set collusive prices in a decentralized manner and through nothing more than profit feedback. This raises the question as to whether collusive pricing can be prevented through the design of suitable "buy boxes," i.e., through the design of the rules that govern the elements of e-commerce sites that promote particular products and prices to consumers. In previous work, Johnson et al. (2020) designed hand-crafted buy box rules that use demand-steering, based on the history of pricing by sellers, to prevent collusive behavior. Although effective against price collusion, these rules effect this by imposing severe restrictions on consumer choice and consumer welfare. In this paper, we demonstrate that reinforcement learning (RL) can also be used by platforms to learn buy box rules that are effective in preventing collusion by RL sellers, and to do so without reducing consumer choice. For this, we adopt the methodology of Stackelberg MDPs, and demonstrate success in learning robust rules that continue to provide high consumer welfare together with sellers employing different behavior models or having out-of-distribution costs for goods.
更多查看译文
关键词
Multi-agent Systems,Reinforcement Learning,Platform Design
AI 理解论文
溯源树
样例
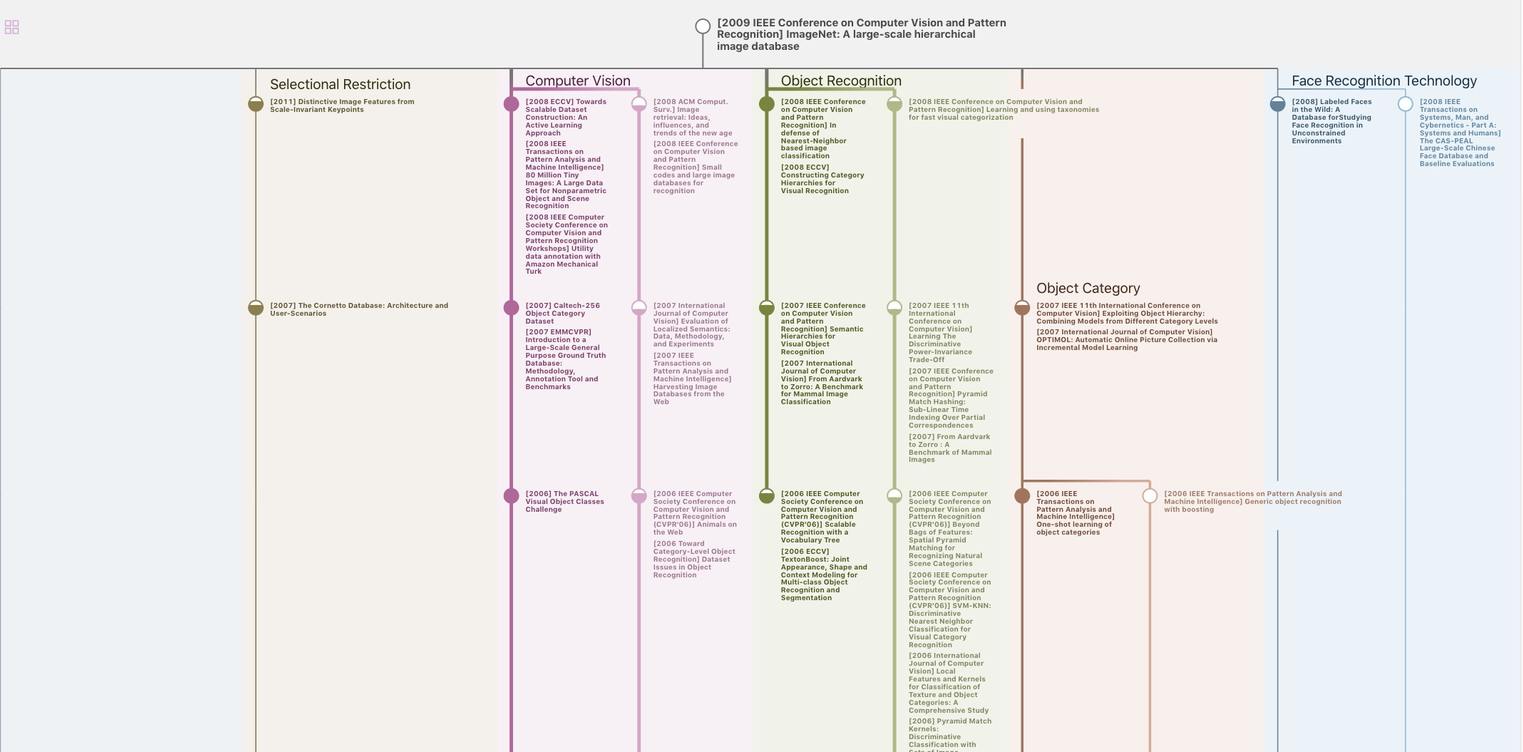
生成溯源树,研究论文发展脉络
Chat Paper
正在生成论文摘要