A spatiotemporal graph generative adversarial networks for short-term passenger flow prediction in urban rail transit systems
arxiv(2023)
摘要
Most short-term passenger flow prediction methods only consider absolute errors as the optimization objective, which fails to account for spatial and temporal constraints on the predictions. To overcome these limitations, we propose a deep learning-based spatiotemporal graph generative adversarial network (STG-GAN) to accurately predict network-wide short-term passenger flows of the urban rail transit with higher efficiency and lower memory occupancy. Our model is optimized in an adversarial learning manner and includes (1) a generator network including gated temporal conventional networks (TCN) and weight sharing graph convolution networks (GCN) to capture structural spatiotemporal dependencies and generate predictions with a small computational burden; (2) a discriminator network including a spatial discriminator and a temporal discriminator to enhance spatial and temporal constraints of the predictions. The STG-GAN is evaluated on two datasets from Beijing Subway. Results illustrate its superiority and robustness.
更多查看译文
关键词
Deep learning,short-term passenger flow prediction,GCN,GAN,urban rail transit
AI 理解论文
溯源树
样例
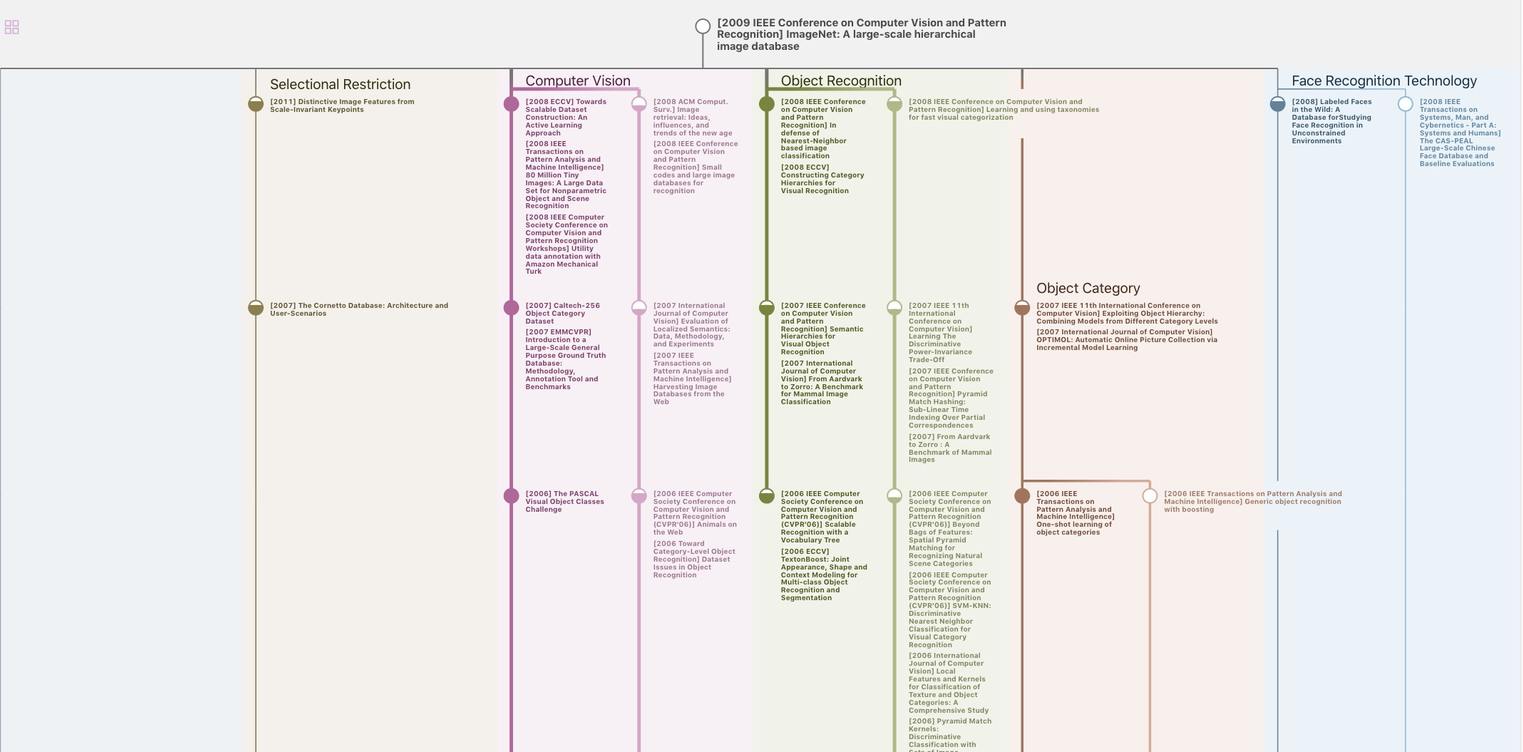
生成溯源树,研究论文发展脉络
Chat Paper
正在生成论文摘要