Saute RL: Almost Surely Safe Reinforcement Learning Using State Augmentation.
International Conference on Machine Learning(2022)
摘要
Satisfying safety constraints almost surely (or with probability one) can be critical for the deployment of Reinforcement Learning (RL) in real-life applications. For example, plane landing and take-off should ideally occur with probability one. We address the problem by introducing Safety Augmented (Saute) Markov Decision Processes (MDPs), where the safety constraints are eliminated by augmenting them into the state-space and reshaping the objective. We show that Saute MDP satisfies the Bellman equation and moves us closer to solving Safe RL with constraints satisfied almost surely. We argue that Saute MDP allows viewing the Safe RL problem from a different perspective enabling new features. For instance, our approach has a plug-and-play nature, i.e., any RL algorithm can be "Sauteed”. Additionally, state augmentation allows for policy generalization across safety constraints. We finally show that Saute RL algorithms can outperform their state-of-the-art counterparts when constraint satisfaction is of high importance.
更多查看译文
关键词
safe reinforcement learning,reinforcement learning,augmentation
AI 理解论文
溯源树
样例
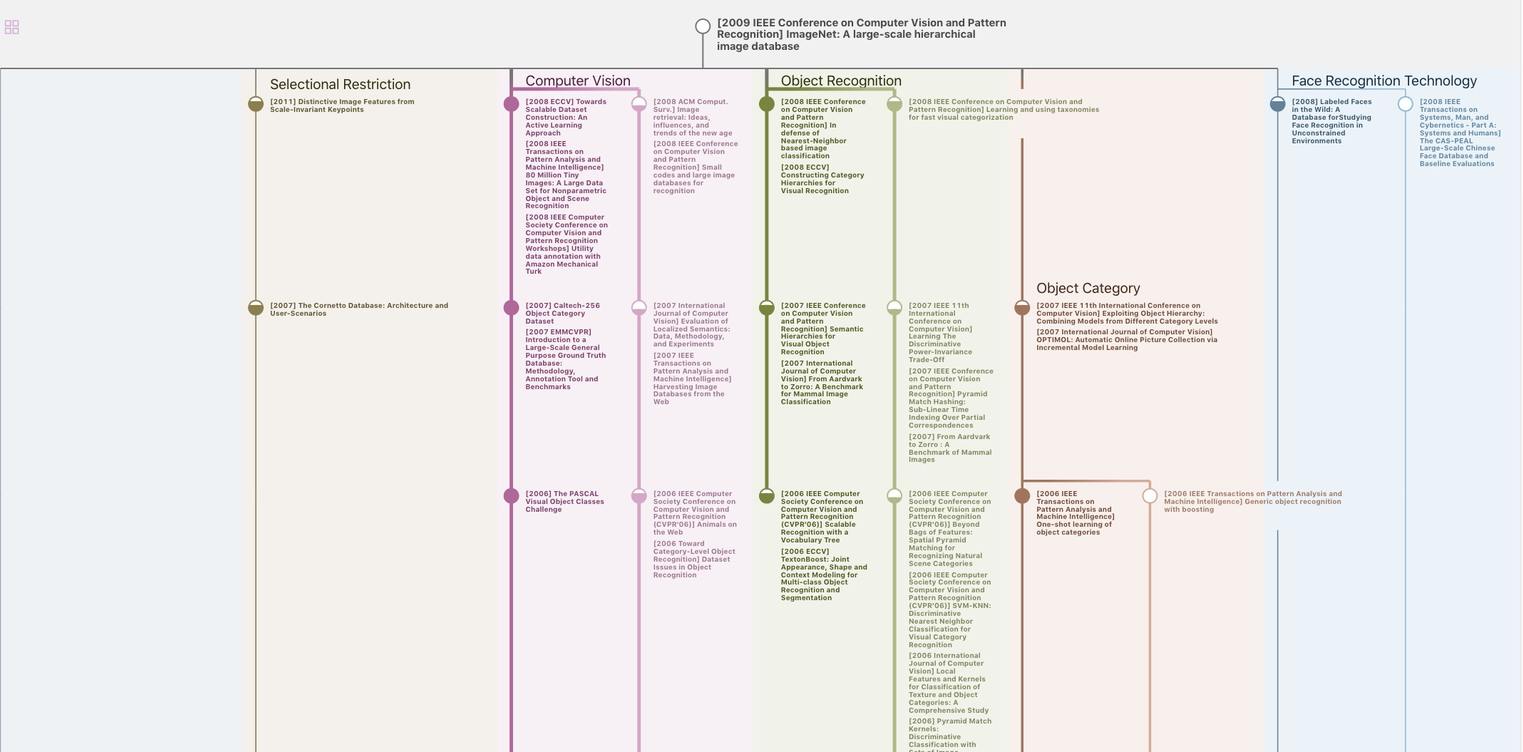
生成溯源树,研究论文发展脉络
Chat Paper
正在生成论文摘要