Defense Strategies Toward Model Poisoning Attacks in Federated Learning: A Survey
2022 IEEE WIRELESS COMMUNICATIONS AND NETWORKING CONFERENCE (WCNC)(2022)
摘要
Advances in distributed machine learning can empower future communications and networking. The emergence of federated learning (FL) has provided an efficient framework for distributed machine learning, which, however, still faces many security challenges. Among them, model poisoning attacks have a significant impact on the security and performance of FL. Given that there have been many studies focusing on defending against model poisoning attacks, it is necessary to survey the existing work and provide insights to inspire future research. In this paper, we first classify defense mechanisms for model poisoning attacks into two categories: evaluation methods for local model updates and aggregation methods for the global model. Then, we analyze some of the existing defense strategies in detail. We also discuss some potential challenges and future research directions. To the best of our knowledge, we are the first to survey defense methods for model poisoning attacks in FL.
更多查看译文
关键词
Federated learning, security, model poisoning attacks, defense
AI 理解论文
溯源树
样例
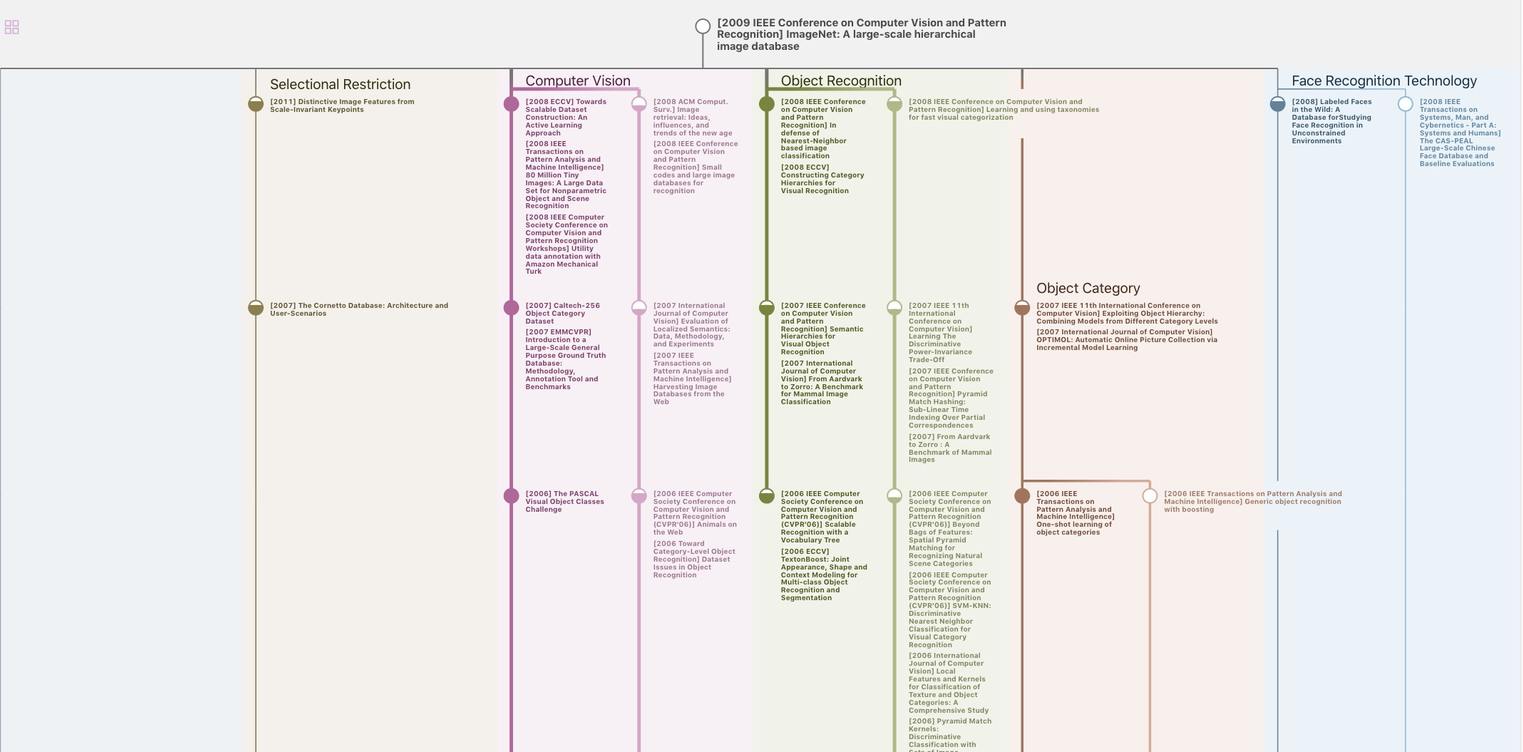
生成溯源树,研究论文发展脉络
Chat Paper
正在生成论文摘要