Gradient-Free Optimization for Non-Smooth Saddle Point Problems under Adversarial Noise
arxiv(2023)
摘要
We consider non-smooth saddle point optimization problems. To solve these problems, we propose a zeroth-order method under bounded or Lipschitz continuous noise, possible adversarial. In contrast to the state-of-the-art algorithms, our algorithm is optimal in terms of both criteria: oracle calls complexity and the maximum value of admissible noise. The proposed method is simple and easy to implement as it is built on zeroth-order version of the stochastic mirror descent. The convergence analysis is given in terms of the average and probability. We also pay special attention to the duality gap $r$-growth condition $(r\geq 1)$, for which we provide a modification of our algorithm using the restart technique. We also comment on infinite noise variance and upper bounds in the case of Lipschitz noise. The results obtained in this paper are significant not only for saddle point problems but also for convex optimization.
更多查看译文
关键词
optimization,noise,gradient-free,non-smooth
AI 理解论文
溯源树
样例
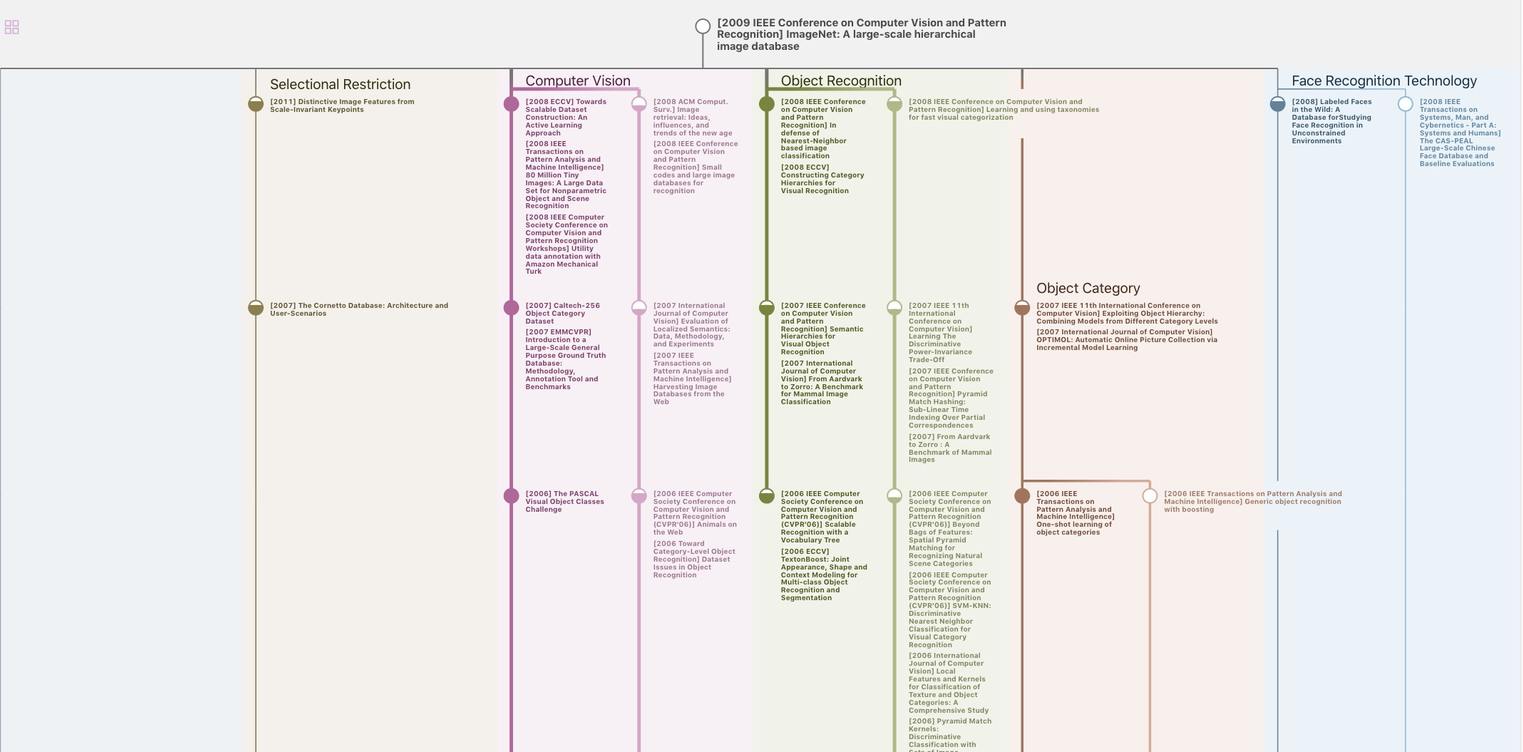
生成溯源树,研究论文发展脉络
Chat Paper
正在生成论文摘要