Assessing Privacy Risks from Feature Vector Reconstruction Attacks
arxiv(2022)
摘要
In deep neural networks for facial recognition, feature vectors are numerical representations that capture the unique features of a given face. While it is known that a version of the original face can be recovered via "feature reconstruction," we lack an understanding of the end-to-end privacy risks produced by these attacks. In this work, we address this shortcoming by developing metrics that meaningfully capture the threat of reconstructed face images. Using end-to-end experiments and user studies, we show that reconstructed face images enable re-identification by both commercial facial recognition systems and humans, at a rate that is at worst, a factor of four times higher than randomized baselines. Our results confirm that feature vectors should be recognized as Personal Identifiable Information (PII) in order to protect user privacy.
更多查看译文
关键词
privacy risks
AI 理解论文
溯源树
样例
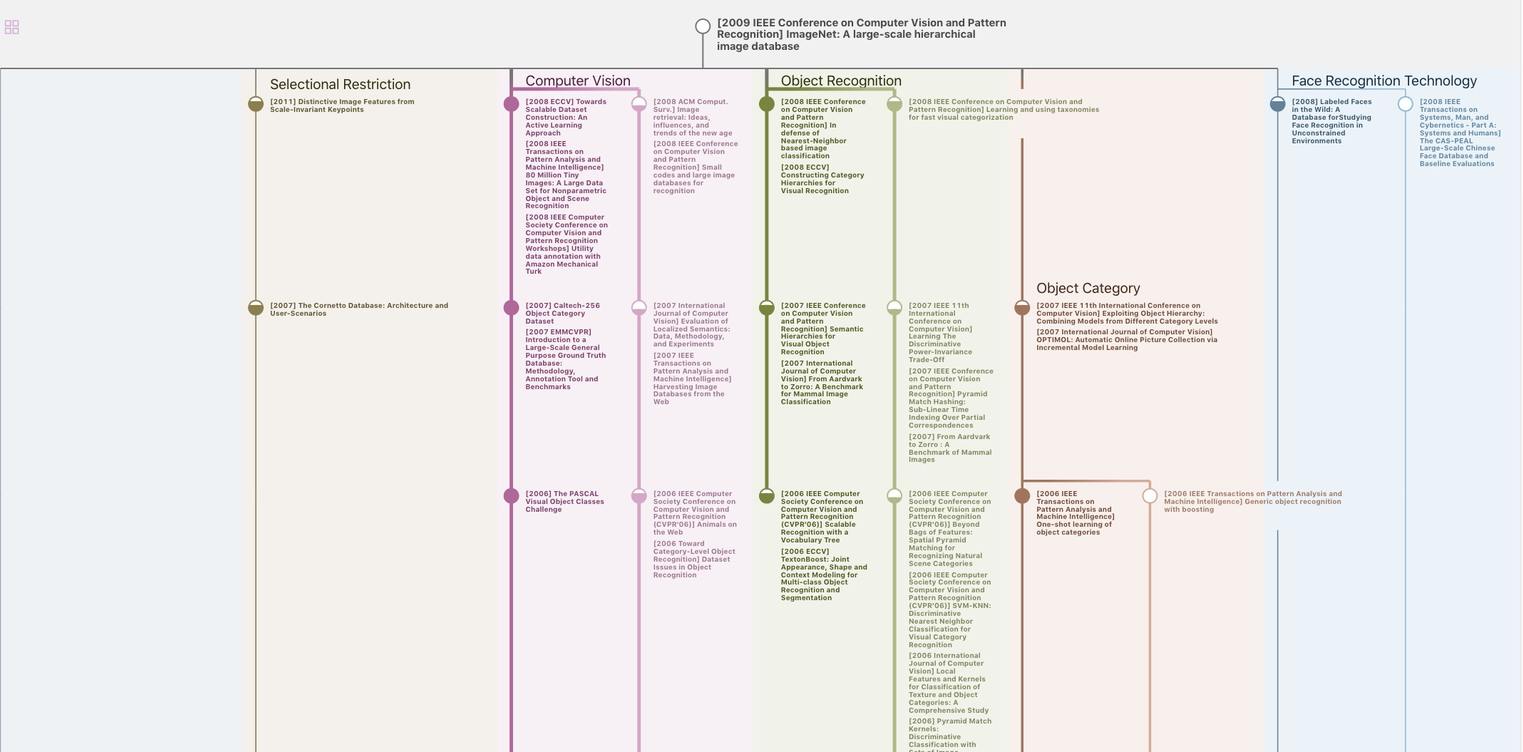
生成溯源树,研究论文发展脉络
Chat Paper
正在生成论文摘要