Bounded nonlinear forecasts of partially observed geophysical systems with physics-constrained deep learning
arxiv(2022)
摘要
The complexity of real-world geophysical systems is often compounded by the fact that the observed measurements depend on hidden variables. These latent variables include unresolved small scales and/or rapidly evolving processes, partially observed couplings, or forcings in coupled systems. This is the case in ocean-atmosphere dynamics, for which unknown interior dynamics can affect surface observations. The identification of computationally-relevant representations of such partially-observed and highly nonlinear systems is thus challenging and often limited to short-term forecast applications. Here, we investigate the physics-constrained learning of implicit dynamical embeddings, leveraging neural ordinary differential equation (NODE) representations. A key objective is to constrain their boundedness, which promotes the generalization of the learned dynamics to arbitrary initial condition. The proposed architecture is implemented within a deep learning framework, and its relevance is demonstrated with respect to state-of-the-art schemes for different case-studies representative of geophysical dynamics.
更多查看译文
关键词
Partially-observed systems,Embedding,Boundedness,Deep learning,Neural ODE,Forecasting
AI 理解论文
溯源树
样例
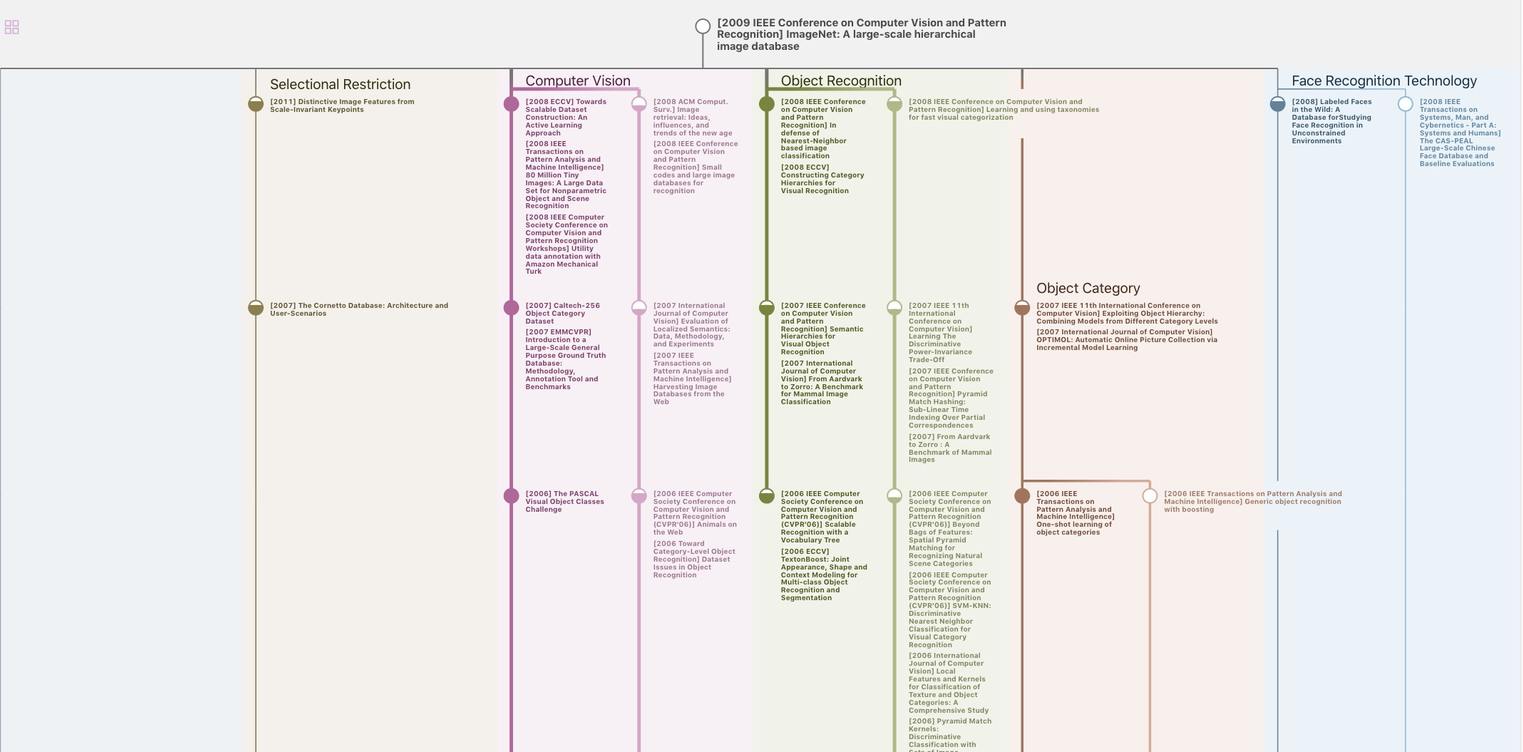
生成溯源树,研究论文发展脉络
Chat Paper
正在生成论文摘要