Machine Learning Revealed Ferroptosis Features and a Novel Ferroptosis-Based Classification for Diagnosis in Acute Myocardial Infarction
FRONTIERS IN GENETICS(2022)
摘要
Acute myocardial infarction (AMI) is a leading cause of death and disability worldwide. Early diagnosis of AMI and interventional treatment can significantly reduce myocardial damage. However, owing to limitations in sensitivity and specificity, existing myocardial markers are not efficient for early identification of AMI. Transcriptome-wide association studies (TWASs) have shown excellent performance in identifying significant gene-trait associations and several cardiovascular diseases (CVDs). Furthermore, ferroptosis is a major driver of ischaemic injury in the heart. However, its specific regulatory mechanisms remain unclear. In this study, we screened three Gene Expression Omnibus (GEO) datasets of peripheral blood samples to assess the efficiency of ferroptosis-related genes (FRGs) for early diagnosis of AMI. To the best of our knowledge, for the first time, TWAS and mRNA expression data were integrated in this study to identify 11 FRGs specifically expressed in the peripheral blood of patients with AMI. Subsequently, using multiple machine learning algorithms, an optimal prediction model for AMI was constructed, which demonstrated satisfactory diagnostic efficiency in the training cohort (area under the curve (AUC) = 0.794) and two external validation cohorts (AUC = 0.745 and 0.711). Our study suggests that FRGs are involved in the progression of AMI, thus providing a new direction for early diagnosis, and offers potential molecular targets for optimal treatment of AMI.
更多查看译文
关键词
machine learning, ferroptosis, acute myocardial infarction, early diagnosis, prediction model
AI 理解论文
溯源树
样例
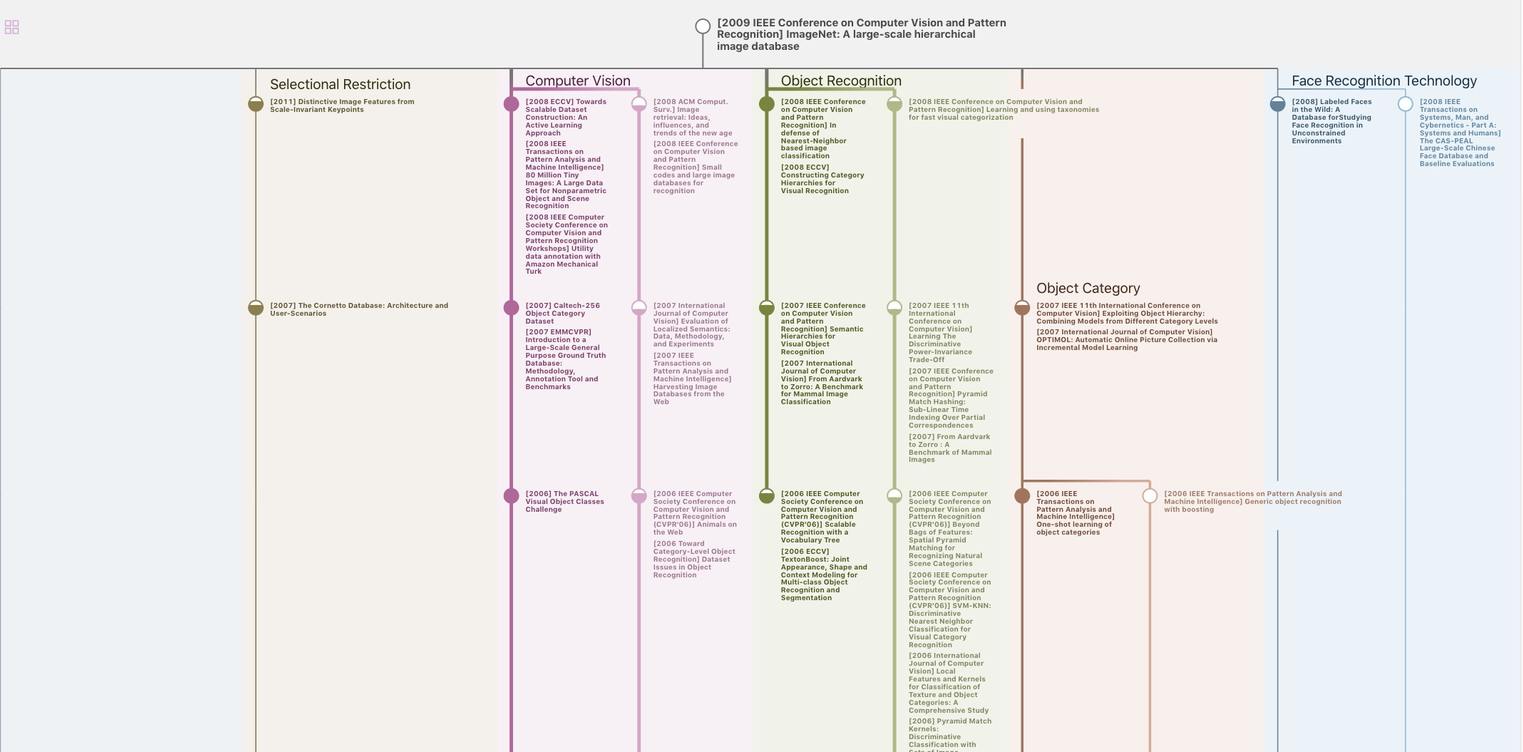
生成溯源树,研究论文发展脉络
Chat Paper
正在生成论文摘要