Polycystic liver: automatic segmentation using deep learning on CT is faster and as accurate compared to manual segmentation
European Radiology(2022)
Abstract
Objective This study aimed to develop and investigate the performance of a deep learning model based on a convolutional neural network (CNN) for the automatic segmentation of polycystic livers at CT imaging. Method This retrospective study used CT images of polycystic livers. To develop the CNN, supervised training and validation phases were performed using 190 CT series. To assess performance, the test phase was performed using 41 CT series. Manual segmentation by an expert radiologist (Rad1a) served as reference for all comparisons. Intra-observer variability was determined by the same reader after 12 weeks (Rad1b), and inter-observer variability by a second reader (Rad2). The Dice similarity coefficient (DSC) evaluated overlap between segmentations. CNN performance was assessed using the concordance correlation coefficient (CCC) and the two-by-two difference between the CCCs; their confidence interval was estimated with bootstrap and Bland-Altman analyses. Liver segmentation time was automatically recorded for each method. Results A total of 231 series from 129 CT examinations on 88 consecutive patients were collected. For the CNN, the DSC was 0.95 ± 0.03 and volume analyses yielded a CCC of 0.995 compared with reference. No statistical difference was observed in the CCC between CNN automatic segmentation and manual segmentations performed to evaluate inter-observer and intra-observer variability. While manual segmentation required 22.4 ± 10.4 min, central and graphics processing units took an average of 5.0 ± 2.1 s and 2.0 ± 1.4 s, respectively. Conclusion Compared with manual segmentation, automated segmentation of polycystic livers using a deep learning method achieved much faster segmentation with similar performance. Key Points • Automatic volumetry of polycystic livers using artificial intelligence method allows much faster segmentation than expert manual segmentation with similar performance. • No statistical difference was observed between automatic segmentation, inter-observer variability, or intra-observer variability.
MoreTranslated text
Key words
Deep learning,Biometry,Liver diseases,Cysts,Tomography, X-ray computed
AI Read Science
Must-Reading Tree
Example
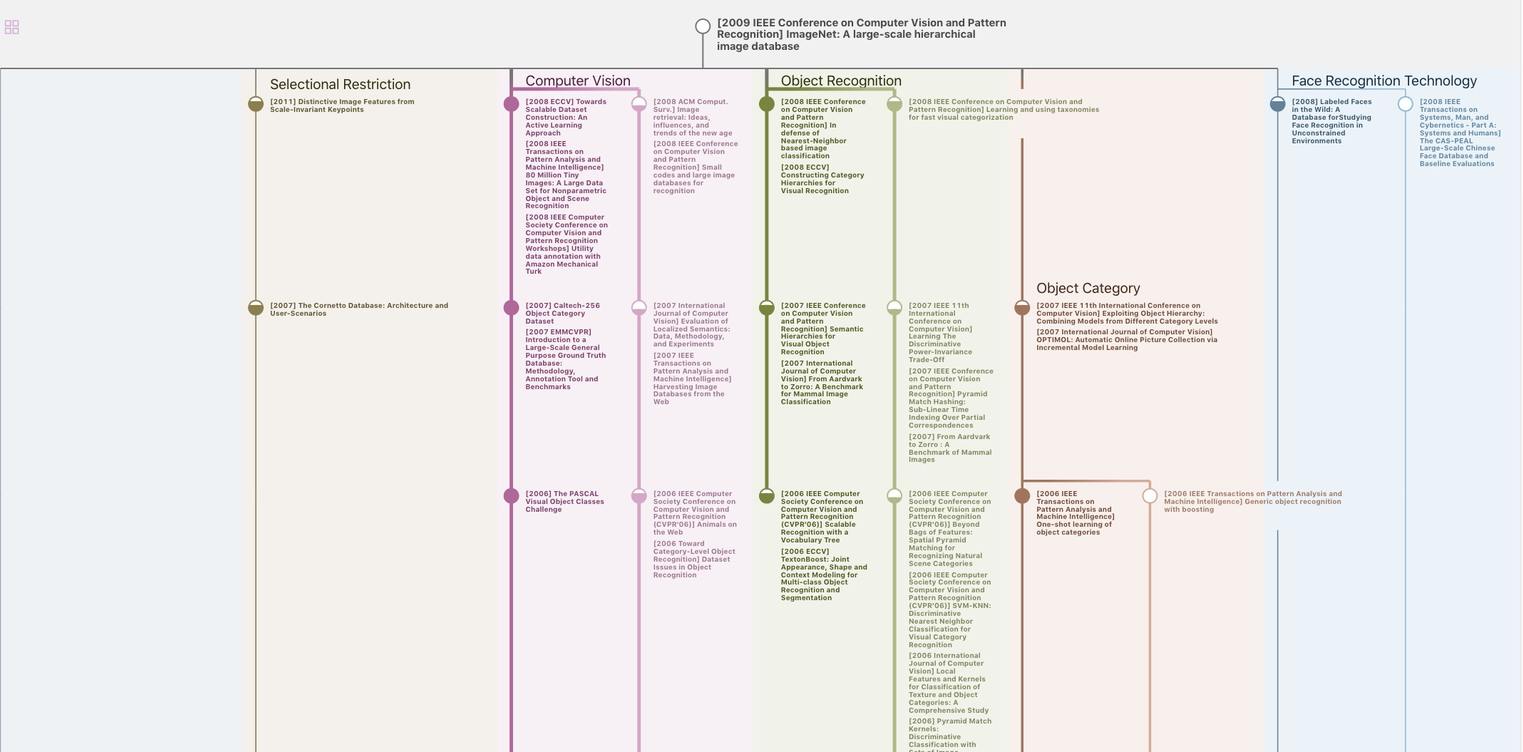
Generate MRT to find the research sequence of this paper
Chat Paper
Summary is being generated by the instructions you defined