WSSNet: Aortic Wall Shear Stress Estimation Using Deep Learning on 4D Flow MRI
FRONTIERS IN CARDIOVASCULAR MEDICINE(2022)
摘要
Wall shear stress (WSS) is an important contributor to vessel wall remodeling and atherosclerosis. However, image-based WSS estimation from 4D Flow MRI underestimates true WSS values, and the accuracy is dependent on spatial resolution, which is limited in 4D Flow MRI. To address this, we present a deep learning algorithm (WSSNet) to estimate WSS trained on aortic computational fluid dynamics (CFD) simulations. The 3D CFD velocity and coordinate point clouds were resampled into a 2D template of 48 x 93 points at two inward distances (randomly varied from 0.3 to 2.0 mm) from the vessel surface ("velocity sheets"). The algorithm was trained on 37 patient-specific geometries and velocity sheets. Results from 6 validation and test cases showed high accuracy against CFD WSS (mean absolute error 0.55 +/- 0.60 Pa, relative error 4.34 +/- 4.14%, 0.92 +/- 0.05 Pearson correlation) and noisy synthetic 4D Flow MRI at 2.4 mm resolution (mean absolute error 0.99 +/- 0.91 Pa, relative error 7.13 +/- 6.27%, and 0.79 +/- 0.10 Pearson correlation). Furthermore, the method was applied on in vivo 4D Flow MRI cases, effectively estimating WSS from standard clinical images. Compared with the existing parabolic fitting method, WSSNet estimates showed 2-3 x higher values, closer to CFD, and a Pearson correlation of 0.68 +/- 0.12. This approach, considering both geometric and velocity information from the image, is capable of estimating spatiotemporal WSS with varying image resolution, and is more accurate than existing methods while still preserving the correct WSS pattern distribution.
更多查看译文
关键词
4D Flow MRI, computational fluid dynamics, deep learning, wall shear stress (WSS), aorta
AI 理解论文
溯源树
样例
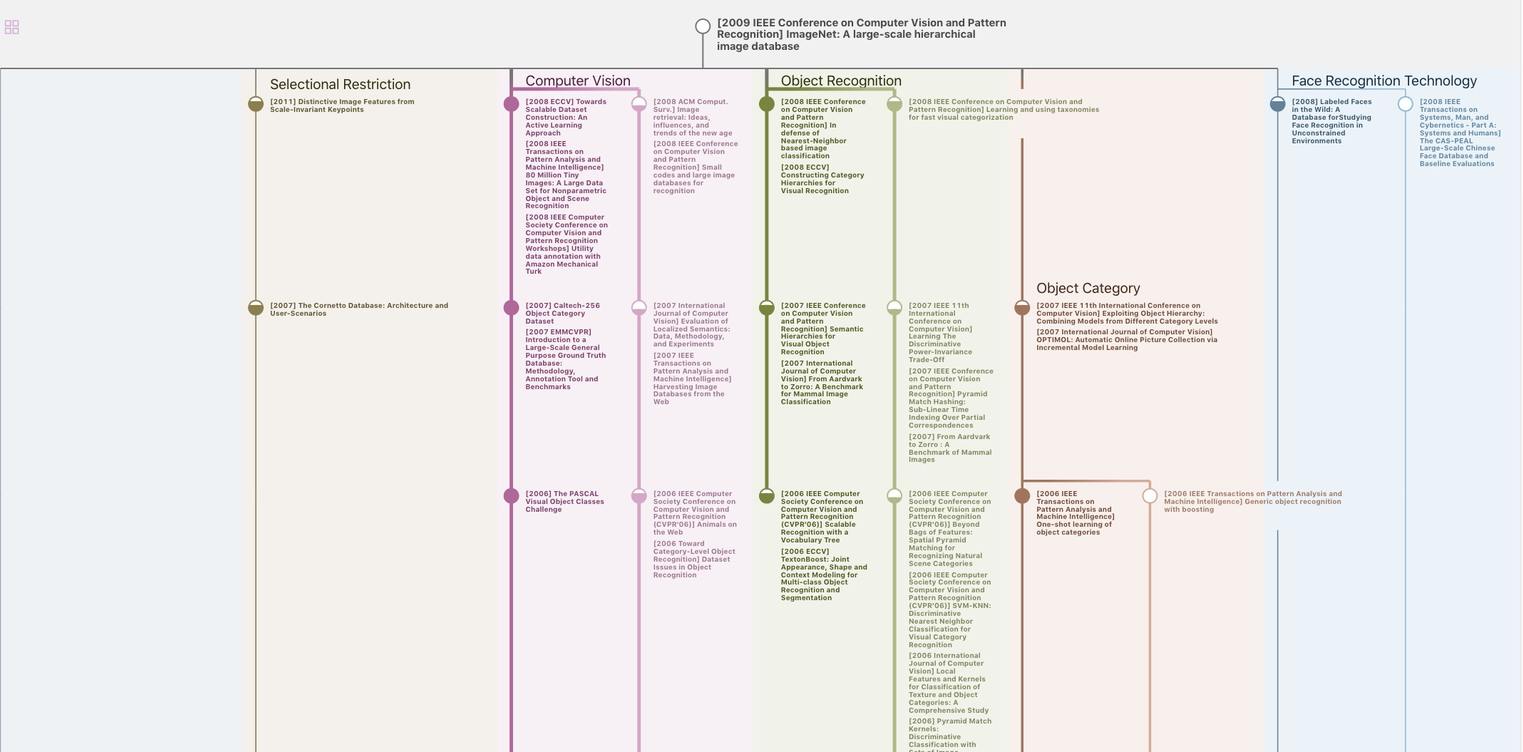
生成溯源树,研究论文发展脉络
Chat Paper
正在生成论文摘要