No-Regret Learning in Dynamic Stackelberg Games
IEEE TRANSACTIONS ON AUTOMATIC CONTROL(2024)
摘要
In a Stackelberg game, a leader commits to a randomized strategy and a follower chooses their best strategy in response. We consider an extension of a standard Stackelberg game, called a discrete-time dynamic Stackelberg game, that has an underlying state space that affects the leader's rewards and available strategies and evolves in a Markovian manner depending on both the leader and follower's selected strategies. Although standard Stackelberg games have been utilized to improve scheduling in security domains, their deployment is often limited by requiring complete information of the follower's utility function. In contrast, we consider scenarios where the follower's utility function is unknown to the leader; however, it can be linearly parameterized. Our objective is then to provide an algorithm that prescribes a randomized strategy to the leader at each step of the game based on observations of how the follower responded in previous steps. We design an online learning algorithm that, with high probability, is no-regret, i.e., achieves a regret bound (when compared to the best policy in hindsight), which is sublinear in the number of time steps; the degree of sublinearity depends on the number of features representing the follower's utility function. The regret of the proposed learning algorithm is independent of the size of the state space and polynomial in the rest of the parameters of the game. We show that the proposed learning algorithm outperforms existing model-free reinforcement learning approaches.
更多查看译文
关键词
Games,Heuristic algorithms,Atmospheric modeling,Standards,Security,Reinforcement learning,Markov processes,Iterative learning control,no-regret learning,online learning,Stackelberg games,stochastic games
AI 理解论文
溯源树
样例
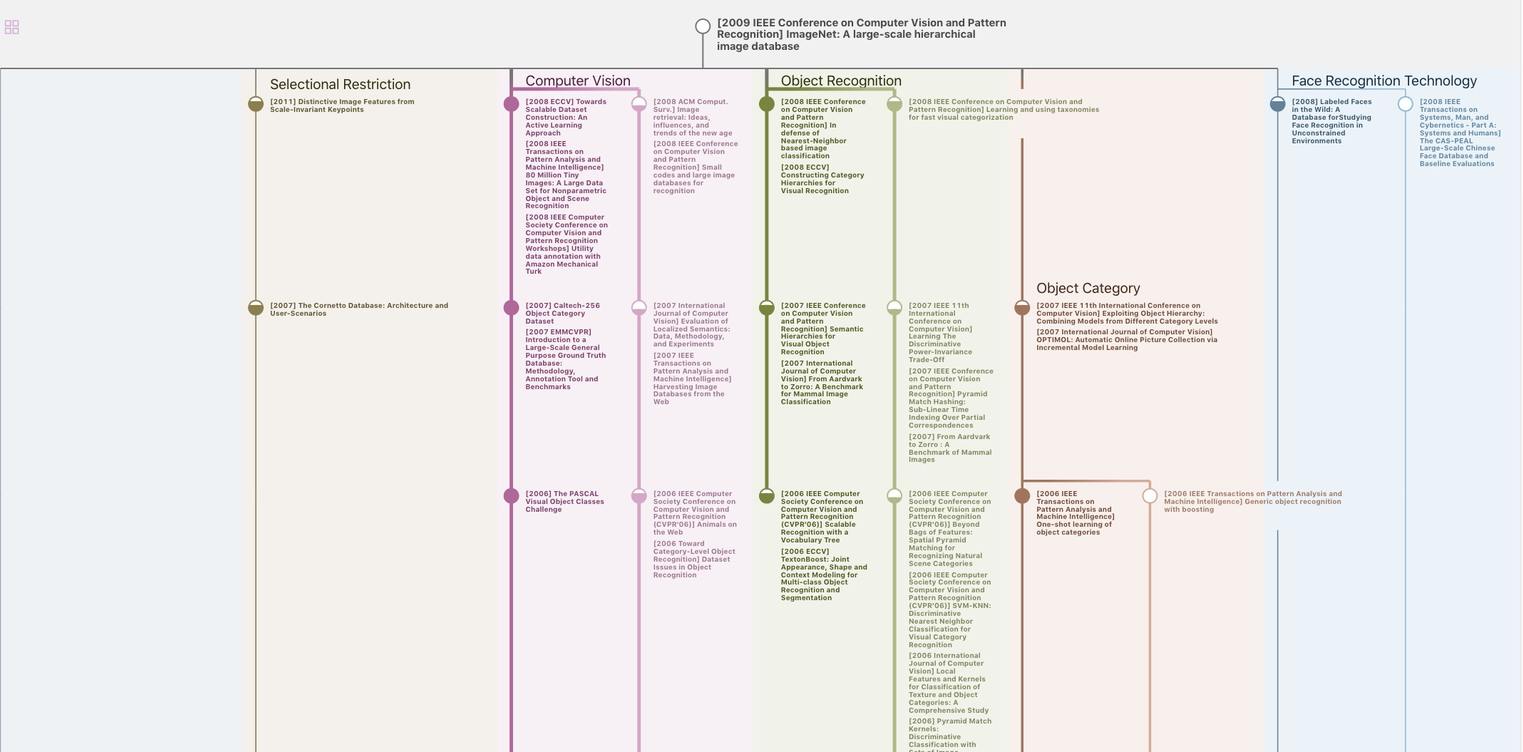
生成溯源树,研究论文发展脉络
Chat Paper
正在生成论文摘要