Obtaining Dyadic Fairness by Optimal Transport
arxiv(2022)
摘要
Fairness has been taken as a critical metric in machine learning models, which is considered as an important component of trustworthy machine learning. In this paper, we focus on obtaining fairness for popular link prediction tasks, which are measured by dyadic fairness. A novel pre-processing methodology is proposed to establish dyadic fairness through data repairing based on optimal transport theory. With the well-established theoretical connection between the dyadic fairness for graph link prediction and a conditional distribution alignment problem, the dyadic repairing scheme can be equivalently transformed into a conditional distribution alignment problem. Furthermore, an optimal transport-based dyadic fairness algorithm called DyadicOT is obtained by efficiently solving the alignment problem, satisfying flexibility and unambiguity requirements. The proposed DyadicOT algorithm shows superior results in obtaining fairness compared to other fairness methods on two benchmark graph datasets.
更多查看译文
关键词
Optimal Transport,Dyadic Fairness,Link Prediction
AI 理解论文
溯源树
样例
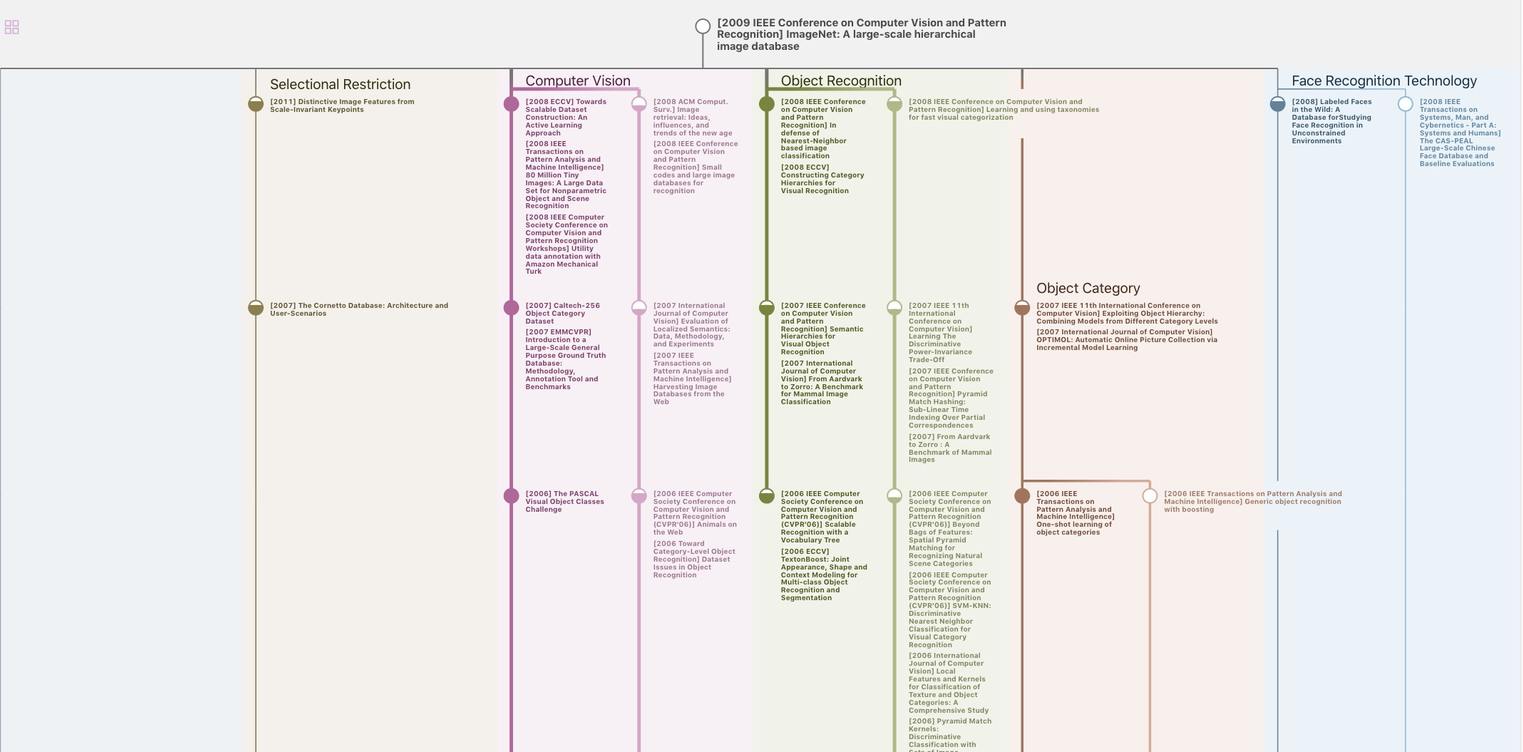
生成溯源树,研究论文发展脉络
Chat Paper
正在生成论文摘要