MBCT: Tree-Based Feature-Aware Binning for Individual Uncertainty Calibration
International World Wide Web Conference(2022)
Abstract
ABSTRACT Most machine learning classifiers only concern classification accuracy, while certain applications (such as medical diagnosis, meteorological forecasting, and computation advertising) require the model to predict the true probability, known as a calibrated estimate. In previous work, researchers have developed several calibration methods to post-process the outputs of a predictor to obtain calibrated values, such as binning and scaling methods. Compared with scaling, binning methods are shown to have distribution-free theoretical guarantees, which motivates us to prefer binning methods for calibration. However, we notice that existing binning methods have several drawbacks: (a) the binning scheme only considers the original prediction values, thus limiting the calibration performance; and (b) the binning approach is non-individual, mapping multiple samples in a bin to the same value, and thus is not suitable for order-sensitive applications. In this paper, we propose a feature-aware binning framework, called Multiple Boosting Calibration Trees (MBCT), along with a multi-view calibration loss to tackle the above issues. Our MBCT optimizes the binning scheme by the tree structures of features, and adopts a linear function in a tree node to achieve individual calibration. Our MBCT is non-monotonic, and has the potential to improve order accuracy, due to its learnable binning scheme and the individual calibration. We conduct comprehensive experiments on three datasets in different fields. Results show that our method outperforms all competing models in terms of both calibration error and order accuracy. We also conduct simulation experiments, justifying that the proposed multi-view calibration loss is a better metric in modeling calibration error. In addition, our approach is deployed in a real-world online advertising platform; an A/B test over two weeks further demonstrates the effectiveness and great business value of our approach.
MoreTranslated text
Key words
Uncertainty calibration, Feature-aware binning, Multiple boosting calibration trees, Multi-view calibration
AI Read Science
Must-Reading Tree
Example
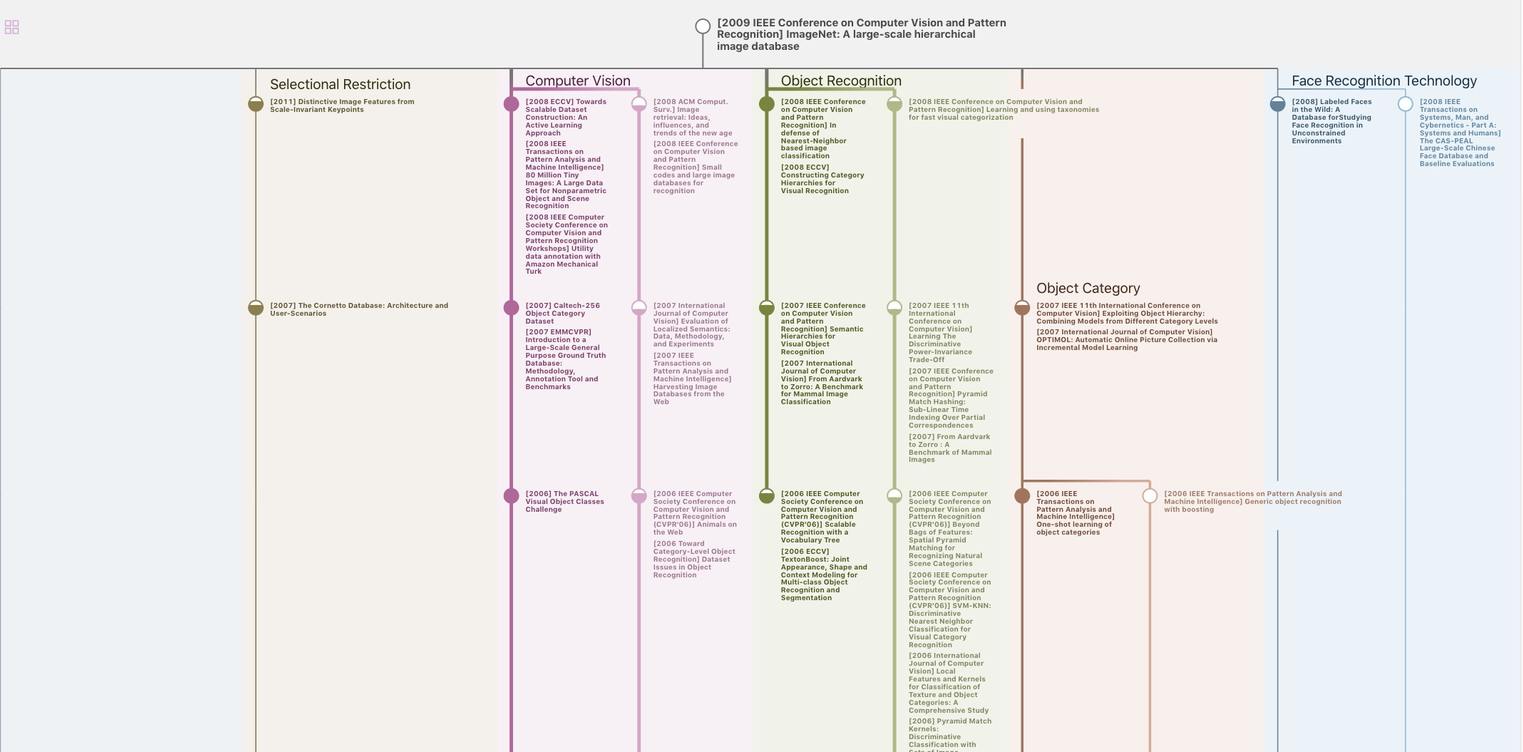
Generate MRT to find the research sequence of this paper
Chat Paper
Summary is being generated by the instructions you defined