Learning under Storage and Privacy Constraints.
International Symposium on Information Theory (ISIT)(2022)
摘要
Storage-efficient privacy-guaranteed learning is crucial due to enormous amounts of sensitive user data required for increasingly many learning tasks. We propose a framework for reducing the storage cost while at the same time providing privacy guarantees, without essential loss in the utility of the data for learning. Our method comprises noise injection followed by lossy compression. We show that, when appropriately matching the lossy compression to the distribution of the added noise, the compressed examples converge, in distribution, to that of the noise-free training data. In this sense, the utility of the data for learning is essentially maintained, while reducing storage and privacy leakage by quantifiable amounts. We present experimental results on the CelebA dataset for gender classification and find that our suggested pipeline delivers in practice on the promise of the theory: the individuals in the images are unrecognizable (or less recognizable, depending on the noise level), overall storage of the data is substantially reduced, with no essential loss of the classification accuracy. As an added bonus, our experiments suggest that our method yields a substantial boost to robustness in the face of adversarial test data.
更多查看译文
关键词
privacy constraints,storage-efficient privacy-guaranteed learning,sensitive user data,storage cost,privacy guarantees,essential loss,noise injection,lossy compression,compressed examples,noise-free training data,privacy leakage,quantifiable amounts,noise level,adversarial test data
AI 理解论文
溯源树
样例
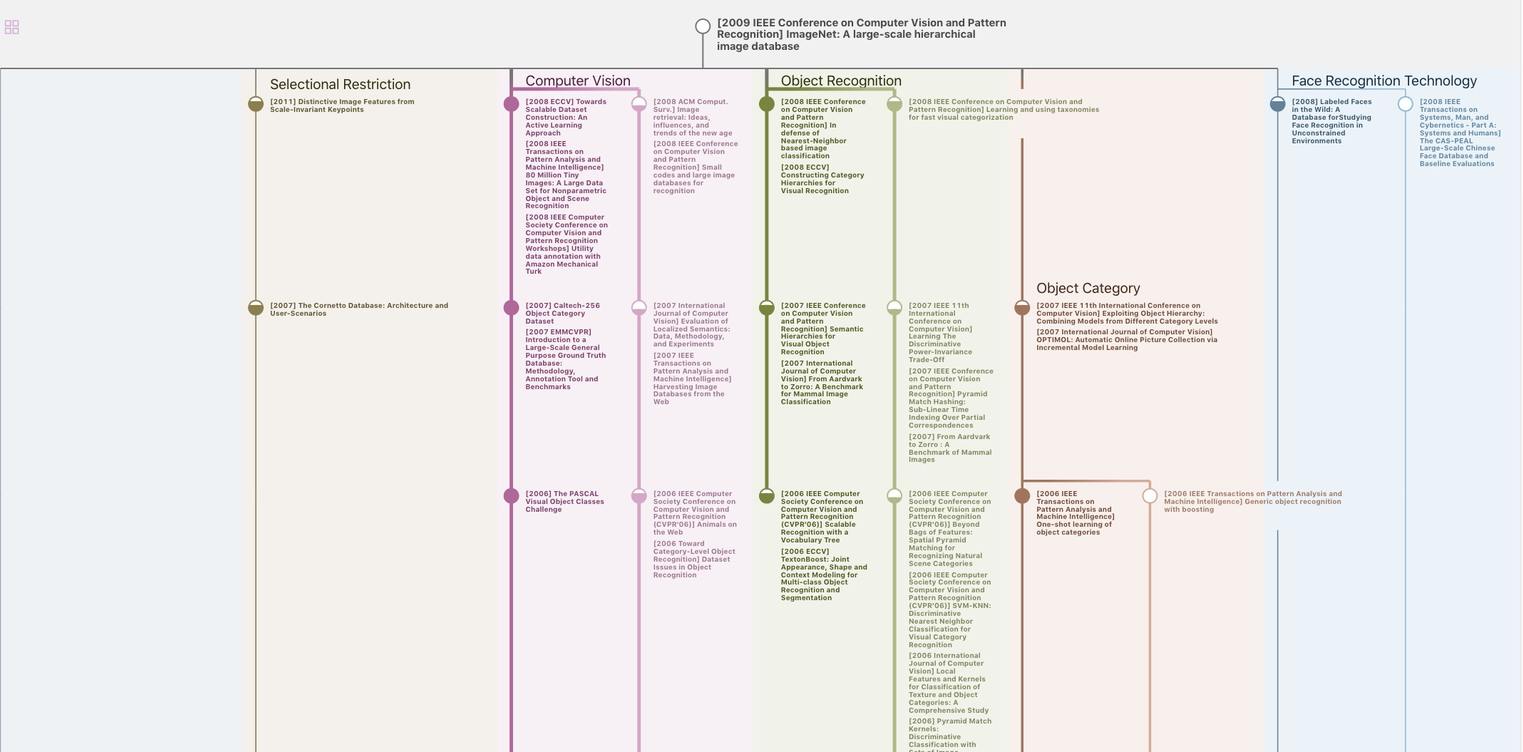
生成溯源树,研究论文发展脉络
Chat Paper
正在生成论文摘要